the Creative Commons Attribution 4.0 License.
the Creative Commons Attribution 4.0 License.
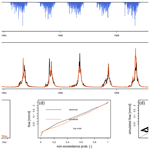
Performance assessment of daily GR conceptual rainfall-runoff models in the Upper Benue River (Cameroon) using airGR packages
Ernest Amoussou
Raphael Muamba Tshimanga
Djan'na Koubodana Houteta
Domiho Japhet Kodja
Franck Eitel Kemgang Ghomsi
André Lenouo
Many hydrological applications employ conceptual-lumped rainfall-runoff models to support water resource management techniques. Because of their ability to exploit minimal data and provide enough credible information, they provide an edge in data-scarce domains. The purpose of this research is to evaluate the feasibility of using multiple rainfall-runoff hydrologic models Génie Rural à 4, 5, 6 paramètres Journalier (GR4J, GR5J, and GR6J) in the Upper Benue River (UBR) in Northern Cameroon. The models were calibrated using Michel's calibration algorithm implemented in airGR packages, while considering different objective functions (NSE, KGE, composite criterion). The objective here was to find the optimum objective function that takes into account the various components of the hydrographs in this watershed and to assess the impact of the chosen objective function on parameter optimization. The results reveal that the model performance as well as the optimized parameters of the different GR models vary according to the selected objective function. According to the study, adopting the composite criterion as an objective function during model calibration improves model accuracy. The model's performance reveals that two of the three models (GR5J and GR6J) reproduced the discharge well in the considered catchment, with NSE and KGE greater than 0.62 during the model validation. This highlights how these two GR models can be applied to various water management concerns in the UBR.
- Article
(1526 KB) - Full-text XML
- BibTeX
- EndNote
UPH 19; Open Science; Modelling; GR hydrological models, airGR packages
Nowadays, hydrological models are essential and powerful tools for the efficient management of water resources. They are used to simulate the different hydrological processes occurring at the watershed scale, to prevent extreme events such as floods and droughts, and are now widely used to study the impacts of climate change and land cover on water resources at the global, regional and local scales (Tshimanga and Hughes, 2012; Nonki et al., 2019, 2021b; Lemaitre-Basset et al., 2021). A large number of hydrological models that vary from physical-based to conceptual models are currently available. But the choice between the distributed, semi-distributed and lumped-conceptual is very important for catchment hydrology. Given the greediness of semi-distributed and distributed models in terms of input data, conceptual-lumped rainfall-runoff models are the most commonly used especially in the context of data-scarce regions (Tegegne et al., 2017). They have the advantage that they can use limited data and generate sufficiently reliable information (Nonki et al., 2021c). They are also usually simple and relatively easy to implement.
They are GR (Génie Rural) models in terms of the number of extant and extensively used conceptual-lumped models (Perrin et al., 2009). The GR models were developed in the early 1980s by Claude Michel at Irstea, a French national applied research institute. Nowadays, there are numerous GR models that differ in terms of both time-step (annual, monthly, daily, and hourly) and the number of free model parameters. Because these models are rather parsimonious, end users can easily code them in their modeling environment. However, providing the models in a default implementation environment can make them easier to use and ensure their correct application. Coron et al. (2017) built the airGR packages in this context, which make the GR models available in R. They provided a GR simulation tool that is free, open source and easy to disseminate. Based on their accuracy to simulate discharge around the world and especially in the African catchments, this study aims to assess the feasibility of applying daily GR models in the Upper Benue River for future water resources management.
2.1 Study area and data
The study is conducted in the Upper Benue River (UBR) located in northern Cameroon between latitudes 7 and 11° N, and Longitudes 12 and 16° E (Fig. 1). It is Cameroon's second largest river with a watershed of 64 000 km2 at Garoua outlet and serves as the main tributary to the Niger River basin. This basin rises in the Adamaoua Plateau and constitutes the most socioeconomic economic impediment of the Northern region because of its potential for sustaining various water-related activities such as irrigated agriculture, hydroelectricity production and navigation. A tropical climate prevails in the basin with two main seasons: the rainy season lasts from May to October and the dry season from November to April. It's a unimodal rainfall region (maximum in August) with annual rainfall ranges between 900 and 1500 mm (Dassou et al., 2016). The prevailing vegetation type in the region is savanna, and elevation is characterized by three main hills: the Adamawa Plateau, and Mounts Alantika and Mandara.
Daily meteorological and hydrological data were used in this study to calibrate and validate the hydrological models. The meteorological data consisted of precipitation obtained from 25 stations and potential evapotranspiration (PET) computed by Penman's formula (Penman, 1948) from solar radiation, relative humidity, wind speed at 2 m, as well as minimum, maximum and average air temperatures from 4 stations located in the basin. These data were provided by the Direction of the National Meteorology of Cameroon (DNM). The daily measured discharge at the Garoua gauging stream station were obtained from the Système d'Information Environnementales sur les Ressources en Eaux en Afrique pour leur Modélisation (SIEREM) database of HydroSciences Montpellier (Boyer et al., 2006; http://www.hydrosciences.org/index.php/2020/08/26/bases-de-donnees/, last access: 1 May 2023).
2.2 Hydrological models description
Three daily time-step GR (Génie Rural) lumped-conceptual rainfall-runoff models were used: the GR model with four daily parameters (GR4J; Perrin et al., 2003), the GR model with five daily parameters (GR5J; Le Moine, 2008), and the GR model with six daily parameters (GR6J; Pushpalatha et al., 2011). To simulate discharge, these models use daily precipitation and PET as inputs. The GR5J is a modified version of the GR4J that includes an additional parameter (X5). It is a threshold for inter-catchment exchange (–) which can take positive or negative values. While GR6J is an upgraded version of GR5J which incorporates an additional routing store (X6: exponential store controlling parameter, mm) that allows more flexibility for low-flow modeling (Coron et al., 2017). Figure 2 depicts a schematic view of the various hydrological processes accounted for in the various models, where X1 is the maximum capacity of the production store (mm), X2 is the groundwater exchange coefficient (mm), X3 is the maximum capacity of the routing store (mm) and X4 is the base time of unit hydrograph (days).
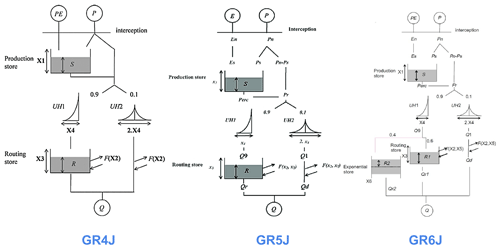
Figure 2Schematic view of the different hydrological processes accounted for in the GR4J, GR5J and GR6J Conceptual-Lumped hydrological models.
2.3 Model calibration, validation and performance assessment
The split – Sample test (Klemeš, 1986) was used for model calibration and validation. Our data time-series were divided into two sub-periods: calibration from 1 January 1961 to 31 December 1970 and validation from 1 January 1971 to 31 December 1980. Calibration/validation was repeated twice, by swapping the two periods (the second half for calibration and first half for validation). By doing this, we can get rid of the dependency of model performance to the simulated period. The model was calibrated using Michel (1991)'s optimization algorithm. These model parameters were calibrated against three different objective function: the Nash and Sutcliffe Efficiency (NSE; Nash and Sutcliffe, 1970), the Kling–Gupta efficiency (KGE; Gupta et al., 2009) and the composite criterion (OF; Lemaitre-Basset et al., 2021) that allowed a fair compromise of both low and high flows. It's the average KGE value, calculated with discharge and inverse of discharge series. The formulation of the two first criteria can be found in Nonki et al. (2021a, c) and the last criterion is given in Eq. (1):
The model's performance was assessed by using both graphical (hydrographs of observed and simulated discharge and flow duration curves) and statistical criteria such as NSE with its different transformations (log and sqrt), KGE, root mean square error (RMSE) and Pearson correlation coefficient (r).
3.1 Impact of selected objective function on optimized parameters and performance
Table 1 gives the optimized model parameters and the performance criteria obtained during the model calibration using different objective functions. The results reveal that the optimum model parameters for the three models differ depending on the criterion used. This result was rather predictable given that we trained the model for different purposes. We also notice that the use of a composite criterion as an objective function during model calibration is highly recommended due to its ability to account both low and high flows components (represented by NSE(log Q) and respectively) compared to NSE and KGE in which the models fail to simulate low flows. This result corroborates with those of Garcia et al. (2017) which recommended using OF as an objective function to simulate low-flow indices with continuous conceptual rainfall–runoff models. Despite the fact that increasing model parameters number increase the model uncertainty, we found that increasing the number of model parameters improves model accuracy. This means that the addition of one process to the model improve its performance.
3.2 Models' evaluation results
To evaluate the model performance, we used the optimized model parameters obtained using composite criterion as objective function. Figures 3 and 4 show the comparison between the observed and simulated hydrograph as well as flow duration curves during the calibration and validation using the GR6J model. It's clear that the model captures the timing and magnitude of the discharge well in the UBR. In addition, the model well captured the annual cycle of discharge. However, we also notice that the model fails to simulate the low flows compared to high flows. The same results are achieved with GR5J although they differ slightly with GR4J (Figures not shown).
Table 2Statistical summary of the model performance of the three daily GR models during the calibration and validation periods at the Benue River (Garoua hydrometric station). The models were calibrated using OF as objective function.
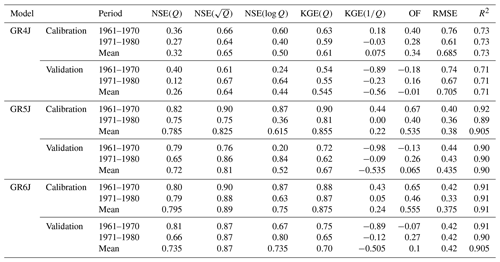
These findings are reinforced by multi-statistical criteria analysis shown in Table 2. Calibration and validation of the models within the same sub period reveal that the model performed better during model calibration. This result is not surprising as the model is calibrated to better depict the watershed's hydrologic conditions during the calibration period, which are never exactly the same throughout model validation (Merz et al., 2009). In addition, the models outperform during the first half of the calibration period and during the second half of the validation period. The GR5J and GR6J models clearly outperformed the GR4J model in the UBR. This can be explained by the fact that Northern Cameroon is a semi-arid region in Cameroon where many rivers dry up a few months after the rainy season, then the groundwater significantly contributes to the stream flow during the dry season. Therefore, the better the groundwater exchange is taken into account in the hydrological model, the better the accuracy is. An objective examination demonstrates that the GR6J model outperforms the other two models. This finding is consistent with earlier research (Flores et al., 2021; Tyralis et al., 2023).
The aim of this study was to assess the viability of applying daily GR Conceptual-Lumped models in the Upper Benue River for future water management challenges. We used the airGR packages in R, which give GR models. The Michel's algorithm implemented in these packages was used for model optimization using three different objective criteria, and the model's performance was evaluated using different statistical criteria. The results showed that the optimized parameters vary according to the selected objective function and suggested the use of composite criteria as an objective function during the model calibration. In addition, the GR5J and GR6J models outperformed the GR4J model. This demonstrates that adding one reservoir to the model for groundwater simulation outperforms the model.
This study was conducted using airGR packages available online (https://CRAN.R-project.org/package=airGR, Coron et al., 2023). Meteorological and hydrological data are the properties of the Direction of the National Meteorology of Cameroon (DNM) and the SIEREM/HSM database respectively. Interested users can contact the corresponding author to have access to the data.
RMN: Funding acquisition, Conceptualization, Methodology, Software, Formal analysis, Validation, Writing – original draft. EA: Funding acquisition, Conceptualization, Software, Writing – original draft. RMT: Conceptualization, Methodology, Validation, Writing – review and editing. DKH: Data curation, Software, Writing – review and editing. DJK: Software, visualization, Writing – review and editing. FEKG: Investigation and editing. AL: Funding acquisition, editing and supervision.
The contact author has declared that none of the authors has any competing interests.
Publisher’s note: Copernicus Publications remains neutral with regard to jurisdictional claims in published maps and institutional affiliations.
This article is part of the special issue “IAHS2022 – Hydrological sciences in the Anthropocene: Variability and change across space, time, extremes, and interfaces”. It is a result of the XIth Scientific Assembly of the International Association of Hydrological Sciences (IAHS 2022), Montpellier, France, 29 May–3 June 2022.
The authors would like to thank the airGR package developers and the data providers. We also thank the IAHS through the SYSTA award for funding three authors to attend this conference. We thank the Associate Editor and the reviewers, whose comments were important in improving the manuscript.
This research has been supported by the IAHS (through the SYSTA award) and DAAD within the framework of the climapAfrica programme with funds of the Federal Ministry of Education and Research (grant no. 57610298).
This paper was edited by Christophe Cudennec and reviewed by Alban de Lavenne and one anonymous referee.
Boyer, J. F., Dieulin, C., Rouche, N., Cres, A., Servat, E., Paturel, J. E., and Mahé, G.: SIEREM: an environmental information system for water resources, 5th World FRIEND Conference, La Havana, Cuba, November 2006, Climate Variability and Change – Hydrological Impacts IAHS Publ., 308, 19–25, 2006.
Coron, L., Thirel, G., Delaigue, O., Perrin, C., and Andréassian, V.: The suite of lumped GR hydrological models in an R package, Environ. Model. Soft., 94, 166–171, https://doi.org/10.1016/j.envsoft.2017.05.002, 2017.
Coron, L., Delaigue, O., Thirel, G., Dorchies, D., Perrin, C., and Michel, C.: airGR: Suite of GR Hydrological Models for Precipitation-Runoff Modelling, R Package Version 1.7.4, CRAN [code], https://CRAN.R-project.org/package=airGR (last access: 1 May 2023), 2023.
Dassou, E., Ombolo, A., Chouto, S., Mboudou, G., Essi, J., and Bineli, E.: Trends and geostatistical interpolation of spatio-temporal variability of precipitation in northern Cameroon, Am. J. Clim. Change, 5, 229–244, https://doi.org/10.4236/ajcc.2016.52020, 2016.
Flores, N., Rodríguez, R., Yépez, S., Osores, V., Rau, P., Rivera, D., and Balocchi, F.: Comparison of Three Daily Rainfall-Runoff Hydrological Models Using Four Evapotranspiration Models in Four Small Forested Watersheds with Different Land Cover in South-Central Chile, Water, 13, 3191, https://doi.org/10.3390/w13223191, 2021.
Garcia, F., Folton, N., and Oudin, L.: Which objective function to calibrate rainfall–runoff models for low-flow index simulations?, Hydrol. Sci. J., 62, 1149–1166, 10.1080/02626667.2017.1308511, 2017.
Gupta, H. V., Kling, H., Yilmaz, K. K., and Martinez, G. F.: Decomposition of the mean squared error and NSE performance criteria: implications for improving hydrological modelling, J. Hydrol., 377, 80–91, https://doi.org/10.1016/j.jhydrol.2009.08.003, 2009.
Klemeš, V.: Operational testing of hydrologic simulation models, Hydrol. Sci. J., 31, 13–24, https://doi.org/10.1080/02626668609491024, 1986.
Lemaitre-Basset, T., Collet, L., Thirel, G., Parajka, J., Evin, G., and Hingray, B.: Climate change impact and uncertainty analysis on hydrological extremes in a French Mediterranean catchment, Hydrol. Sci. J., 66, 888–903, 10.1080/02626667.2021.1895437, 2021.
Le Moine, N.: Le Bassin Versant De Surface Vu Par Le Souterrain: Une Voie d'amélioration Des Performances Et Du Réalisme Des Modèles Pluie-débit?, Dissertation, Université Pierre et Marie Curie, Paris, France, https://api.semanticscholar.org/CorpusID:160882984 (last access: 1 May 2023), 2008.
Merz, R., Parajka, J., and Blöschl, G.: Scale effects in conceptual hydrological modeling, Water Resour. Res., 45, W09405, https://doi.org/10.1029/2009WR007872, 2009.
Michel, C.: Hydrologie appliquée aux petits bassins ruraux, Hydrology handbook, Cemagref, Antony, France, 1991 (in French).
Nash, J. E. and Sutcliffe, J. V.: River flow forecasting through conceptual models. Part I – a discussion of principles, J. Hydrol., 10, 282–290, https://doi.org/10.1016/0022-1694(70)90255-6, 1970.
Nonki, R. M., Lenouo, A., Lennard, C. J., and Tchawoua, C.: Assessing climate change impacts on water resources in the Benue River Basin, Northern Cameroon, Environ. Earth Sci., 78, 606, https://doi.org/10.1007/s12665-019-8614-4, 2019.
Nonki, R. M., Lenouo, A., Lennard, C. J., Tshimanga, R. M., and Tchawoua, C.: Comparison between Dynamic and Static Sensitivity Analysis Approaches for Impact Assessment of Different Potential Evapotranspiration Methods on Hydrological Models' Performance, J. Hydrometeorol., 22, 345 2713–2730, https://doi.org/10.1175/JHM-D-20-0192.1, 2021a.
Nonki, R. M., Lenouo, A., Tchawoua, C., Lennard, C. J., and Amoussou, E.: Impact of climate change on hydropower potential of the Lagdo dam, Benue River Basin, Northern Cameroon, Proc. IAHS, 384, 337–342, https://doi.org/10.5194/piahs-384-337-2021, 2021b.
Nonki, R. M., Lenouo, A., Tshimanga, R. M., Donfack, F. C., and Tchawoua, C.: Performance assessment and uncertainty prediction of a daily time-step HBV-Light rainfall-runoff model for the Upper Benue River Basin, Northern Cameroon, J. Hydrol. Reg. Stud., 36, 100849, https://doi.org/10.1016/j.ejrh.2021.100849, 2021c.
Penman, H. L.: Natural evaporation from open water, bare soil and grass, Proc. Roy. Soc. A-Math. Phys., 193, 120–145, https://doi.org/10.1098/rspa.1948.0037, 1948.
Perrin, C., Michel, C., and Andréassian, V.: Improvement of a parsimonious model for streamflow simulation, J. Hydrol., 279, 275–289, https://doi.org/10.1016/S0022-1694(03)00225-7, 2003.
Perrin, C., Michel, C., and Andreassian, V.: A Set of Hydrological Models (Chapter 16), Environmental Hydraulics, edited by: Tanguy, J. M., 279, ISTE Ltd, John Wiley and Sons, Paris, 493–509. http://eu.wiley.com/WileyCDA/WileyTitle/productCd-1848211546.html (last access: 1 May 2023), 2009.
Pushpalatha, R., Perrin, C., Le Moine, N., Mathevet, T., and Andréassian, V.: A downward structural sensitivity analysis of hydrological models to improve low-flow simulation, J. Hydrol., 411, 66–76, https://doi.org/10.1016/j.jhydrol.2011.09.034, 2011.
Tegegne, G., Park, D. K., and Kim, Y. O.: Comparison of hydrological models for the assessment of water resources in a data-scarce region, the Upper Blue Nile river basin, J. Hydrol. Reg. Stud., 14, 49–66, https://doi.org/10.1016/j.ejrh.2017.10.002, 2017.
Tshimanga, R. M. and Hughes, D. A.: Climate change and impacts on the hydrology of the Congo Basin: the case of the northern sub-basins of the Oubangui and Sangha Rivers, Phys. Chem. Earth A/B/C, 50–52, 72–83, https://doi.org/10.1016/j.pce.2012.08.002, 2012.
Tyralis, H., Papacharalampous, G., and Khatami, S.: Expectile-based hydrological modelling for uncertainty estimation: Life after mean, J. Hydrol., 617, 128986, https://doi.org/10.1016/j.jhydrol.2022.128986, 2023.