the Creative Commons Attribution 4.0 License.
the Creative Commons Attribution 4.0 License.
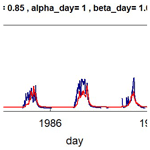
Evaluating the skill of the mesoscale Hydrologic Model (mHM) for discharge simulation in sparsely-gauged basins in Nigeria
Kingsley Nnaemeka Ogbu
Oldrich Rakovec
Luis Samaniego
Gloria Chinwendu Okafor
Bernhard Tischbein
Hadush Meresa
Predictive hydrologic modelling to understand and support agricultural water resources management and food security policies in Nigeria is a demanding task due to the paucity of hydro-meteorological measurements. This study assessed the skill of using different remotely sensed rainfall products in a multi-calibration framework for evaluating the performance of the mesoscale hydrologic Model (mHM) across four different data-scarce basins in Nigeria. Grid-based rainfall estimates obtained from several sources were used to drive the mHM in different basins in Nigeria. Model calibration was first performed using only discharge records, and also by using a combination of discharge and actual evapotranspiration, forced with different rainfall products. The mHM forced with CHIRPS produced reasonable Kling-Gupta efficiency KGE) results (0.5> KGE <0.85) under both calibration frameworks. However, constraining model parameters under a multi-calibration arrangement showed no significant discharge simulation improvement in this study. Results show the utility of the mHM for discharge simulation in data-sparse basins in Nigeria.
- Article
(2182 KB) - Full-text XML
- BibTeX
- EndNote
UPH 20; MPR; discharge; CHIRPS
Modelling of hydrologic systems is crucial to understanding how climatic variables drive hydrologic responses, which are highly sensitive to land use/cover changes and population dynamics. Quantifying the components of the water balance model is strategic to policymakers and water resources managers for developing key water resources management projects (Nesru et al., 2020). In Nigeria, in-situ measurement of hydrologic variables is largely constrained by financial instability, weak institutions and political instability resulting in a steady decline and uneven distributions of existing hydro-meteorological networks (Poméon et al., 2018; Adeoti, 2020). Precipitation acts as a key forcing in the hydrologic system and affects the spatial and temporal variability of other hydrologic processes. Gridded rainfall products provide continuous and spatially-homogenous estimates and have become an alternative, especially in data-scarce regions (Ayehu et al., 2018). However, their ability to reproduce observed hydrologic processes is a precondition for use in water resources modelling (Dembélé et al., 2020).
The availability of remotely-sensed datasets (elevation, soil, land use/cover, climatic variables) led to the development of complex hydrologic models but these efforts did not impact model results (Poméon et al., 2018). This is because the traditional method of model calibration which involves determining the best model parameter set could reproduce observed hydrologic variables but misrepresent important watershed processes (Rajib et al., 2018). The importance of understanding hydrologic processes and improving their mathematical representation is well highlighted during the International Association of Hydrologic Sciences (IAHS) scientific decade (2003–2012) (Hrachowitz et al., 2013). In other to overcome the problems of over-parameterization and equifinality, the Mesoscale Parameter Regionalization (MPR) proposed by Samaniego et al. (2010) presents a technique which links model parameters at a coarser scale with their counterparts at a finer resolution using pedotransfer functions, whereby only the global parameters that define these relationships are obtained through calibration. In comparison with other regionalization methods (e.g. standard regionalization), MPR showed superiority in preserving the spatial variability of state variables and overall performance of model hydrologic processes simulation (Samaniego et al., 2010; Kumar et al., 2010). The MPR technique reduces the number of free mHM calibration parameters and seeks to address Question 20 (reducing model uncertainty) of the Unsolved Problems in Hydrology (UPH 20) (Blöschl et al., 2019). In this study, the key research question is: what is the performance of the mHM-MPR technique in reproducing the temporal variation of the streamflow process under a paucity of input-data conditions? This is the first attempt in applying the mHM-MPR technique for hydrologic modelling in data-scarce basins within Nigeria. This study assessed the suitability of using gridded-rainfall products in a multi-calibration framework for evaluating the performance of mHM for river discharge simulations across four (4) different data-scarce basins in Nigeria.
2.1 Study Area
The study area consists of four (4) river basins located in the northern part of Nigeria (Lat 4.00–14.00° N, Lon 2.00–15.00° E), which were selected based on the availability of discharge data: Jamaare (13 929.711 km2), Hadejia (16 820.336 km2), Kaduna (64 848.594 km2) and Oroo (4500.174 km2) as shown in Fig. 1. Rainfall in this region is unimodal and is impacted by the movement of the Inter-Tropical Discontinuity (ITD). Jamaare, Hadejia and Kaduna basins receive an annual mean rainfall of about 434–969 mm while the Oroo basin is characterized by an annual mean of 897–1535 mm (Gbode et al., 2019; Ogbu et al., 2020).
2.2 The Mesoscale Hydrologic Model (mHM)
The mHM is a grid-based, conceptual and fully distributed hydrologic model which simulates various hydrologic processes (evapotranspiration, infiltration, surface and subsurface runoff, etc.) that are formulated based on the HBV model (Kumar et al., 2013; Samaniego et al., 2010). Spatial-temporal simulations of hydrologic processes in the mHM are processed at the grid/cell scale. Three levels of gridded information are required for mHM set-up; Level-0 (basin characteristics), Level-1 (dominant hydrological processes) and Level-2 (meteorological datasets), to account for sub-grid variability (Kumar et al., 2013). The MPR proposed by a study (Samaniego et al., 2010) is the main feature in the mHM model and serves to bridge the gap between observations and the basin scale (Rakovec et al., 2019). The MPR involves a two-step parameterization procedure (see Fig. 2); (1) model parameters at Level-0 are regionalized by linking them with their corresponding basin characteristics through linear or non-linear transfer functions; (2) In this stage, effective parameters are obtained by linking the regionalized parameters with their corresponding one at Level-0 through an upscaling operator. The major goal of the MPR is to derive global model parameters that are spatially seamless, scale-independent and transferable across locations (Rakovec et al., 2019).
2.3 Data
In this study, mHM was set up using grid-based meteorological, soil, land use and morphological datasets. Rainfall estimates were obtained from the Climate Hazards Group InfraRed Precipitation with Station data (CHIRPS), Climate Prediction Center (CPC), European Center for Medium-Range Weather Forecast (ECMWF) Reanalysis 5th Generation (ERA5), Global Precipitation Climatological Center (GPCC) and Multi-Source Weighted Ensemble Precipitation (MSWEP) and used as model forcing. Potential evapotranspiration was computed with the Hargreaves method using daily temperature data obtained from the ERA5 product. These climatic products were selected based on their performances in previous studies (Poméon et al., 2017; Dembélé et al., 2020; Ogbu et al., 2020, 2022; Hounguè et al., 2021) within the West African region. Soil attributes for six different soil layers were extracted from the Harmonized World Soil Database, version 1.2 while Land use and cover information were obtained from Globecover product. Slope, aspect, flow accumulation and flow direction were derived from a 90 m resolution digital elevation model obtained from the Shuttle Radar Topographic Mission database. mHM calibration and validation were performed using discharge and AET information obtained from the German-developed Global Runoff Data Center (GRDC) and Global Land Evaporation Amsterdam Model (GLEAM) databases, respectively.
2.4 Model set-up
mHM Version 5.11 (Samaniego et al., 2021) was set up in four (4) data-scarce basins while varying rainfall inputs for each setup. In the first case, calibration and validation were performed using only Qobs while in the second case, they were performed using Qobs and AET. Simulation and calibration periods vary for all basins due to the paucity of data and large gaps in existing discharge time series. Discharge (Q) optimization was performed using the Dynamically Dimensioned Search (DDS) (Tolson and Shoemaker, 2007) algorithm (4000 iterations), based on the Kling-Gupta Efficiency (KGE) (Kling et al., 2012; Gupta et al., 2009) metric at a daily time step. Best model parameter sets are obtained with the DDS algorithm by using about 10 %–20 % of the number of iterations required by the Shuffle Complex Evolution optimization method (Rakovec et al., 2019). The KGE is an improved version of Nash Sutcliffe Efficiency (NSE) and constitutes correlation (r), variability and mean bias as shown in Eq. (1).
where, r= linear correlation, α= measure of flow variability error, β= bias.
The multivariable calibration, using Q and domain average AET (Eq. 2) was used to complement Qobs to assess if a more realistic result will be achieved.
where, SO30= mHM objective function Number 30, KGE = Kling-Gupta Efficiency, RMSE root mean square error of the basin average actual ET simulation.
3.1 Results
3.1.1 Model Performance for Discharge
Generally, model results varied across rainfall datasets for all domains during calibration and validation periods for Qobs simulation (Fig. 3). Daily discharge simulations showed reasonable results (KGE >0.5) in all domains except for MSWEP (in Hadejia), GPCC (in Oroo) and CPC (in Kaduna) during model calibration. On the other hand, validation results showed KGE >0.5 in Jamaare Basin (for ERA5, CHIRPS and CPC) and Oroo Basin (for CHIRPS).
An example of daily mHM simulated hydrographs for Jamaare River Basin during model calibration (Fig. 4) and validation (Fig. 5) showed an acceptable fit with the Qobs time series. However, the KGE value decreased from 0.85 (during model calibration) to 0.61 (during model validation) (see Figs. 4 and 5).
3.1.2 Model Performance for Qobs and AET Model Calibration Scheme
In the Qobs/AET model calibration setup, daily streamflow simulations exhibited the same trend if not slightly worse as in the Qobs model calibration scheme (Fig. 6). This result is similar to a previous mHM study (Poméon et al., 2018) in West Africa, with the Qobs model calibration scheme showing improved discharge predictions. Unsatisfactory KGE results (<0.5) were obtained in Oroo Basin (driven by GPCC) and Kaduna Basin (forced with GPCC) during model calibration. For the validation period, discharge simulations showed acceptable results (SO30 >0.5) in Oroo (driven by CHIRPS), Hadejia (CPC and MSWEP) and Jamaare (ERA5, CHIRPS, 0.51068). We showed example hydrographs for the Jamaare River Basin produced using Qobs/AET model calibration setup (Fig. 7) and for model validation (Fig. 8). For this example, SO30 value decreased from 0.85 (calibration) –0.64 (validation) while correlation followed the same trend from 0.85–0.78.
3.2 Discussions
The flow simulation performances driven by the different gridded rainfall products under the different optimization frameworks vary across the different domains, modelled in this study. Overall, simulated streamflow exhibited acceptable KGE values (KGE >0.50) during calibration periods than during model validation for most of the rainfall products. These poor performances could be attributed to gaps in discharge observations, which exist more within validation periods. Small KGE improvements achieved when mHM was calibrated with both Qobs and AET were only evident in Hadejia and Jamaare basins and do not reflect mHM robustness for this study. This poor performance in discharge simulation displayed when model parameters are constrained under a multivariable calibration scheme was also reported in another study (Poméon et al., 2018) conducted within the West Africa region. Visual inspection of simulated and observed hydrographs showed that the CHIRPS outperformed other rainfall products in mimicking observed discharge trends as shown in Figs. 4, 5, 7 and 8. The performances of the CHIRPS dataset in most of the domains are in line with its ability in reproducing observed rainfall at a point-to-pixel scale in the West African region as shown in several studies (Ogbu et al., 2020; Poméon et al., 2017; Dembélé and Zwart, 2016). In this study, satisfactory KGE scores obtained in most domains while using remotely sensed rainfall datasets, especially during model calibration could be attributed to the implementation of the MPR technique within the mHM structure, which ensured that the number of free calibration parameters was reduced while preserving its spatial variability.
In this study, the discharge simulation skill of the mHM was evaluated in four data-limited basins located in Nigeria, under multivariable optimization setups. The MPR technique, which integrates the spatial heterogeneity of a domain's physiographic characteristics and overcomes the problem of model over-parameterization, is well suited for application in this data-scarce region. Notwithstanding the large gaps in Qobs, the reanalysis product (ERA5) and the satellite rainfall dataset (CHIRPS) were consistent in satisfactory discharge simulations in most of the domains. However, significant improvements in discharge simulations were not observed when mHM was calibrated using Qobs and AET. Currently, the mHM lacks a reservoir component and several dams, which exist within the study domains, were not included during model setups. Furthermore, mHM accepts only three land use and land cover classes (impervious, pervious, and forest) which do not represent different existing classes. This study presents the utility of different gridded-rainfall datasets for discharge simulation in data-scarce regions in Nigeria. However, investments in hydro-climatic instrumentations should be given top priority by the government at all levels in Nigeria as remote-sensing rainfall products can only complement in-situ records.
The mHM code, version 5.10 (accessed on 1 December 2020) can be obtained from Zenodo (https://doi.org/10.5281/zenodo.1069202, Samaniego et al., 2021).
Conceptualization: KNO, LS, BT. Formal analysis: KNO, OR. Methodology: KNO, OR, HM. Supervision: LS, BT. Visualization: KNO, OR, GCO. Writing: KNO, OR, LS, BT, GCO, HM.
The contact author has declared that none of the authors has any competing interests.
Publisher's note: Copernicus Publications remains neutral with regard to jurisdictional claims in published maps and institutional affiliations.
This article is part of the special issue ”IAHS2022 – Hydrological sciences in the Anthropocene: Past and future of open, inclusive, innovative, and society-interfacing approaches”. It is a result of the XIth Scientific Assembly of the International Association of Hydrological Sciences (IAHS 2022), Montpellier, France, 29 May–3 June 2022.
The first author acknowledges the providers of all freely available gridded climate dataset used in this study and the mHM team at the Department of Computational Hydrosystems, Helmholtz Centre for Environmental Research (UFZ), Germany for the computing resources used in this research.
This work is a part of the doctoral research of the first author and was funded by Nnamdi Azikiwe University, Awka, Nigeria and the Centre for Development Research (ZEF), University of Bonn, Germany.
This paper was edited by Christophe Cudennec and reviewed by two anonymous referees.
Adeoti, O.: Constraints on data collection implementation at the river basin level in Nigeria, J. Hydrol. Reg. Stud., 32, 100738, https://doi.org/10.1016/j.ejrh.2020.100738, 2020.
Ayehu, G. T., Tadesse, T., Gessesse, B., and Dinku, T.: Validation of new satellite rainfall products over the Upper Blue Nile Basin, Ethiopia, Atmos. Meas. Tech., 11, 1921–1936, https://doi.org/10.5194/amt-11-1921-2018, 2018.
Blöschl, G., Bierkens, M. F. P., Chambel, A., Cudennec, C., Destouni, G., Fiori, A., Kirchner, J. W., McDonnell, J. J., Savenije, H. H. G., Sivapalan, M., Stumpp, C., Toth, E., Volpi, E., Carr, G., Lupton, C., Salinas, J., Széles, B., Viglione, A., Aksoy, H., Allen, S. T., Amin, A., Andréassian, V., Arheimer, B., Aryal, S. K., Baker, V., Bardsley, E., Barendrecht, M. H., Bartosova, A., Batelaan, O., Berghuijs, W. R., Beven, K., Blume, T., Bogaard, T., Borges de Amorim, P., Böttcher, M. E., Boulet, G., Breinl, K., Brilly, M., Brocca, L., Buytaert, W., Castellarin, A., Castelletti, A., Chen, X., Chen, Y., Chen, Y., Chifflard, P., Claps, P., Clark, M. P., Collins, A. L., Croke, B., Dathe, A., David, P. C., de Barros, F. P. J., de Rooij, G., Di Baldassarre, G., Driscoll, J. M., Duethmann, D., Dwivedi, R., Eris, E., Farmer, W. H., Feiccabrino, J., Ferguson, G., Ferrari, E., Ferraris, S., Fersch, B., Finger, D., Foglia, L., Fowler, K., Gartsman, B., Gascoin, S., Gaume, E., Gelfan, A., Geris, J., Gharari, S., Gleeson, T., Glendell, M., Gonzalez Bevacqua, A., González-Dugo, M. P., Grimaldi, S., Gupta, A. B., Guse, B., Han, D., Hannah, D., Harpold, A., Haun, S., Heal, K., Helfricht, K., Herrnegger, M., Hipsey, M., Hlaváčiková, H., Hohmann, C., Holko, L., Hopkinson, C., Hrachowitz, M., Illangasekare, T. H., Inam, A., Innocente, C., Istanbulluoglu, E., Jarihani, B., Kalantari, Z., Kalvans, A., Khanal, S., Khatami, S., Kiesel, J., Kirkby, M., Knoben, W., Kochanek, K., Kohnová, S., Kolechkina, A., Krause, S., Kreamer, D., Kreibich, H., Kunstmann, H., Lange, H., Liberato, M. L. R., Lindquist, E., Link, T., Liu, J., Loucks, D. P., Luce, C., Mahé, G., Makarieva, O., Malard, J., Mashtayeva, S., Maskey, S., Mas-Pla, J., Mavrova-Guirguinova, M., Mazzoleni, M., Mernild, S., Misstear, B. D., Montanari, A., Müller-Thomy, H., Nabizadeh, A., Nardi, F., Neale, C., Nesterova, N., Nurtaev, B., Odongo, V. O., Panda, S., Pande, S., Pang, Z., Papacharalampous, G., Perrin, C., Pfister, L., Pimentel, R., Polo, M. J., Post, D., Prieto Sierra, C., Ramos, M. H., Renner, M., Reynolds, J. E., Ridolfi, E., Rigon, R., Riva, M., Robertson, D. E., Rosso, R., Roy, T., Sá, J. H. M., Salvadori, G., Sandells, M., Schaefli, B., Schumann, A., Scolobig, A., Seibert, J., Servat, E., Shafiei, M., Sharma, A., Sidibe, M., Sidle, R. C., Skaugen, T., Smith, H., Spiessl, S. M., Stein, L., Steinsland, I., Strasser, U., Su, B., Szolgay, J., Tarboton, D., Tauro, F., Thirel, G., Tian, F., Tong, R., Tussupova, K., Tyralis, H., Uijlenhoet, R., van Beek, R., van der Ent, R. J., van der Ploeg, M., Van Loon, A. F., van Meerveld, I., van Nooijen, R., van Oel, P. R., Vidal, J. P., von Freyberg, J., Vorogushyn, S., Wachniew, P., Wade, A. J., Ward, P., Westerberg, I. K., White, C., Wood, E. F., Woods, R., Xu, Z., Yilmaz, K. K., and Zhang, Y.: Twenty-three unsolved problems in hydrology (UPH) – a community perspective, Hydrol. Sci. J., 64, 1141–1158, https://doi.org/10.1080/02626667.2019.1620507, 2019.
Dembélé, M. and Zwart, S. J.: Evaluation and comparison of satellite-based rainfall products in Burkina Faso, West Africa, Int. J. Remote Sens., 37, 3995–4014, https://doi.org/10.1080/01431161.2016.1207258, 2016.
Dembélé, M., Schaefli, B., van de Giesen, N., and Mariéthoz, G.: Suitability of 17 gridded rainfall and temperature datasets for large-scale hydrological modelling in West Africa, Hydrol. Earth Syst. Sci., 24, 5379–5406, https://doi.org/10.5194/hess-24-5379-2020, 2020.
Gbode, I. E., Adeyeri, O. E., Menang, K. P., Intsiful, J. D. K., Ajayi, V. O., Omotosho, J. A., and Akinsanola, A. A.: Observed changes in climate extremes in Nigeria, Meteorol. Appl., 26, 642–654, https://doi.org/10.1002/met.1791, 2019.
Gupta, H. V., Kling, H., Yilmaz, K. K., and Martinez, G. F.: Decomposition of the mean squared error and NSE performance criteria: Implications for improving hydrological modelling, J. Hydrol., 377, 80–91, https://doi.org/10.1016/j.jhydrol.2009.08.003, 2009.
Hounguè, N. R., Ogbu, K. N., Almoradie, A. D. S., and Evers, M.: Evaluation of the performance of remotely sensed rainfall datasets for flood simulation in the transboundary Mono River catchment, Togo and Benin, J. Hydrol. Reg. Stud., 36, 100875, https://doi.org/10.1016/j.ejrh.2021.100875, 2021.
Hrachowitz, M., Savenije, H. H. G., Blöschl, G., McDonnell, J. J., Sivapalan, M., Pomeroy, J. W., Arheimer, B., Blume, T., Clark, M. P., Ehret, U., Fenicia, F., Freer, J. E., Gelfan, A., Gupta, H. V., Hughes, D. A., Hut, R. W., Montanari, A., Pande, S., Tetzlaff, D., Troch, P. A., Uhlenbrook, S., Wagener, T., Winsemius, H. C., Woods, R. A., Zehe, E., and Cudennec, C.: A decade of Predictions in Ungauged Basins (PUB)–a review, Hydrolog. Sci. J., 58, 1198–1255, https://doi.org/10.1080/02626667.2013.803183, 2013.
Kling, H., Fuchs, M., and Paulin, M.: Runoff conditions in the upper Danube basin under an ensemble of climate change scenarios, J. Hydrol., 424–425, 264–277, https://doi.org/10.1016/J.JHYDROL.2012.01.011, 2012.
Kumar, R., Samaniego, L., and Attinger, S.: The effects of spatial discretization and model parameterization on the prediction of extreme runoff characteristics, J. Hydrol., 392, 54–69, https://doi.org/10.1016/j.jhydrol.2010.07.047, 2010.
Kumar, R., Samaniego, L., and Attinger, S.: Implications of distributed hydrologic model parameterization on water fluxes at multiple scales and locations, Water Resour. Res., 49, 360–379, https://doi.org/10.1029/2012WR012195, 2013.
Nesru, M., Shetty, A., and Nagaraj, M. K.: Multi-variable calibration of hydrological model in the upper Omo-Gibe basin, Ethiopia, Acta Geophys., 68, 537–551, https://doi.org/10.1007/s11600-020-00417-0, 2020.
Ogbu, K. N., Hounguè, N. R., Gbode, I. E., and Tischbein, B.: Performance evaluation of satellite-based rainfall products over Nigeria, Climate, 8, 103, https://doi.org/10.3390/cli8100103, 2020.
Ogbu, K. N., Rakovec, O., Shrestha, P. K., Samaniego, L., Tischbein, B., and Meresa, H.: Testing the mHM-MPR Reliability for Parameter Transferability across Locations in North–Central Nigeria, Hydrology, 9, 1–23, https://doi.org/10.3390/hydrology9090158, 2022.
Poméon, T., Jackisch, D., and Diekkrüger, B.: Evaluating the performance of remotely sensed and reanalysed precipitation data over West Africa using HBV light, J. Hydrol., 547, 222–235, https://doi.org/10.1016/j.jhydrol.2017.01.055, 2017.
Poméon, T., Diekkrüger, B., and Kumar, R.: Computationally efficient multivariate calibration and validation of a grid-based hydrologic model in sparsely gauged West African river basins, Water, 10, 1418, https://doi.org/10.3390/w10101418, 2018.
Rajib, A., Evenson, G. R., Golden, H. E., and Lane, C. R.: Hydrologic model predictability improves with spatially explicit calibration using remotely sensed evapotranspiration and biophysical parameters, J. Hydrol., 567, 668–683, https://doi.org/10.1016/j.jhydrol.2018.10.024, 2018.
Rakovec, O., Mizukami, N., Kumar, R., Newman, A. J., Thober, S., Wood, A. W., Clark, M. P., and Samaniego, L.: Diagnostic Evaluation of Large-Domain Hydrologic Models Calibrated Across the Contiguous United States, J. Geophys. Res.-Atmos., 124, 13991–14007, https://doi.org/10.1029/2019JD030767, 2019.
Samaniego, L., Kumar, R., and Attinger, S.: Multiscale parameter regionalization of a grid-based hydrologic model at the mesoscale, Water Resour. Res., 46, 1–25, https://doi.org/10.1029/2008WR007327, 2010.
Samaniego, L., Brenner, J., Craven, J., Cuntz, M., Dalmasso, G., Demirel, C. M., Döring, N., Jing, M., Kaluza, M., Kumar, R., Langenberg, B., Mai, J., Müller, S., Musuuza, J., Prykhodko, V., Rakovec, O., Schäfer, D., Schneider, C., Schrön, M., Schüler, L., Schweppe, R., Shrestha, P. K., Spieler, D., Stisen, S., Thober, S., Zink, M., and Attinger, S.: mesoscale Hydrologic Model – mHM v5.11.2, Zenodo, https://doi.org/10.5281/zenodo.1069202, 2021.
Tolson, B. A. and Shoemaker, C. A.: Dynamically dimensioned search algorithm for computationally efficient watershed model calibration, Water Resour. Res., 43, 1–16, https://doi.org/10.1029/2005WR004723, 2007.