the Creative Commons Attribution 4.0 License.
the Creative Commons Attribution 4.0 License.
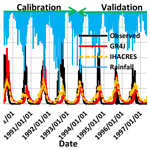
Surface runoff prediction and comparison using IHACRES and GR4J lumped models in the Mono catchment, West Africa
Houteta Djan'na Koubodana
Kossi Atchonouglo
Julien G. Adounkpe
Ernest Amoussou
Domiho Japhet Kodja
Dambré Koungbanane
Koba Yaovi Afoudji
Yao Lombo
Kossi E. Kpemoua
This study aims to assess simulated surface runoff before and after dam construction in the Mono catchment (West Africa) using two lumped models: GR4J (Rural Engineering with 4 Daily Parameters) and IHACRES (Identification of unit Hydrographs and Component flows from Rainfall, Evapotranspiration and Stream data) over two different periods (1964–1986 and 1988–2010). Daily rainfall, mean temperature, evapotranspiration and discharge in situ data were collected for the period 1964–2010. After the model's initialization, calibration and validation; performances analysis have been carried out using multi-objectives functions developed in R software (version 3.5.3). The results indicate that statistical metrics such as the coefficient of determination (R2), the Kling–Gupta Efficiency (KGE), the Nash–Sutcliffe coefficient (NSE) and the Percent of Bias (PBIAS) provide satisfactory insights over the first period of simulation (1964–1986) and low performances over the second period of simulation (1988–2010). In particular, IHACRES model underestimates extreme high runoff of Mono catchment between 1964 and 1986. Conversely, GR4J model overestimates extreme high runoff and has been found to be better for runoff prediction of the river only between 1964 and 1986. Moreover, the study deduced that the robustness of runoff simulation between 1964 and 1986 is better than between 1988 and 2010. Therefore, the weakness of simulated runoff between 1988 and 2010 was certainly due to dam management in the catchment. The study suggests that land cover changes impacts, soil proprieties and climate may also affect surface runoff in the catchment.
- Article
(754 KB) - Full-text XML
- BibTeX
- EndNote
Hydrological modelling is a veritable challenge in West Africa as in many regions in the world owing to limited data access and high percent of gaps in these data. Consequently, climate change impacts on water resource and management and surface runoff prediction become difficult (Komi et al., 2016). Furthermore, dam management, industrialization, irrigation and human activities influences hydrology processes in a watershed (Thompson et al., 2014). For example, land use and land cover play an important role in runoff generation and ground water process (Obahoundje et al., 2018). For example, in Mono catchment Koubodana et al. (2019) have mentioned that forest and savannah have decreased in the benefit of cropland from 1975 to 2013. In the same, catchment the studies such as Amoussou et al. (2012), Kissi et al. (2015), Lawin et al. (2019) and Ntajal et al. (2017) have concluded that the source of high discharge causing flooding in the downstream is not only due to climate change impacts but also to the regulation of the Nangbéto dam, land use and the social factors of the communities living in this catchment. Therefore, it is important to investigate discharge simulation over the catchment using different lumped models and also by considering land cover change constant for the period before Nangbéto dam construction and the period after dam installation in the catchment.
The study is conducted in Mono catchment located in West Africa (Fig. 1). It is a main river in Togo, which is shared with Benin country in its last kilometers in the south. This river is located between 06∘16′ and 9∘20′ N latitude and 0∘42′ and 1∘40′ E longitude. With a perimeter of 872 092 km, the catchment covers from Alédjo to Athiémé an area of 22 013.14 km2 and with 88 % of it area in Togo country and the rest (12 %) in Benin (PCCP, 2008). Its length of 308 773 km, Mono catchment has it source in Alédjo mountains in North of Benin before throwing in Atlantic Ocean by the lagoon system. The elevation of the catchment is range from 12 to 948 m (https://srtm.csi.cgiar.org/, last access: 3 June 2021). The watershed shelters the biggest dam of Nangbéto that produce 20 % of total hydroelectricity used by the two countries (Houessou, 2016). The climate is a subequatorial from 6 to 8∘ N and with two rainy seasons and two dry seasons. It totals 1200 to 1500 mm yr−1 in the mountainous area of the South-West and only, 800 to 1000 mm yr−1 on the coastal zone. From 8 to 10∘ N the climate is tropical humid with one rainy season and one dry season (1000 to 1200 mm yr−1). In the winter months (December to March), there is an anti-cyclonic high-pressure area Centered over the Sahara (Koubodana et al., 2020).
It drives the Harmattan, a desiccating, dusty wind that blows rather persistently from the northeast, drying out landscapes all the way to the coast. However, the hydrograph has one peak that indicates that river discharge is mostly controlled by upstream tributaries. The mean annual temperature ranges from 22 to 30 ∘C (CILSS, 2016; Speth et al., 2010). The population within the Mono catchment is around 3.5 million inhabitants (https://www.oieau.fr/, last access: 3 June 2021). The mains socio economic activities are agriculture, trade, fisheries and livestock husbandry.
3.1 Data used
Daily historical hydro-climatic time series including observation river discharge, rainfall, mean temperature and evapotranspiration collected at the gauge stations are used for this analysis. The datasets were collected at Direction General de l'Eau et Assainissement du Togo (DGEA-Togo), Direction General de l'Eau (DGEau-Benin), General Direction Générale de la Météorologie Nationale du Togo (DGMN-Togo) and Agence Nationale de la Météorologie du Bénin (METEO-Benin) and cover the period from 1964 to 2010. These data sets were initially used for quality control and initial analysis over the catchment by Amoussou et al. (2012) and Koubodana et al. (2020).
3.2 Methods
The mean average of rainfall at Athiémé have been computed by using Thiensen polygons methods of the 21 gauges stations (Fig. 1) in Quantum Geographic Information System (QGIS) (Koubodana et al., 2020; Thiessen, 1911).
The study period was divided first into two-sub period as rainfall breakpoint in 1987 (Koubodana et al., 2020) the pre-dam installation period (1964–1986) and the post-dam installation period (1988–2010) (Table 1).
IHACRES model was used under Hydromad package in R and GR4J model under AirGR package in R. One year was used as model warm-up period in each sub period (Perrin et al., 2003). Calibration was performed for 4 years in each sub period and the same parameters from calibration were used for validation within 5 years after a reasonable compared models sensitivity analysis (Badjana et al., 2017). Four and five years are used with equal length of dry and wet year for calibration and validation periods. The sensitive analysis of IHACRES and GR4J models leading to select optimization parameters and ranges was based on the literature of the past studies done in the region and on Sobol method sensitivity analysis (Badjana et al., 2017; Kodja et al., 2018; Oyerinde et al., 2016).
Many multi-objective functions criterion were adopted in order to evaluate the model parameters optimization: coefficient of determination (R2) (Dougherty et al., 2000), the Nash–Sutcliffe coefficient (NSE) (Nash and Sutcliffe, 1970), Kling–Gupta Efficiency (KGE) (Gupta et al., 2009) and Percent Bias (PBIAS) (Gupta et al., 2009). These criteria were used after calibration and validation in the catchment and for each sub period (Adeaga et al., 2012; Vansteenkiste et al., 2014).
4.1 IHACRES and GR4J model model's performance and uncertainties
GR4J and IHACRES model performance analysis, uncertainties and optimal values are showing in Table 2. The performances values of such as KGE, NSE, R2 and PBIAS after daily calibration and validation are summarized in Table 2. The acceptable values of KGE, NSE and R2 are in bolt in the table below.
Table 2Model's performance – uncertainty and optimized parameters. Acceptable performance values are in bold.
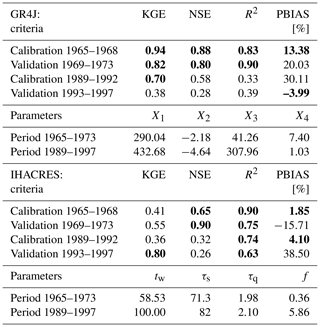
Note: In GR4J model, X1 is maximum capacity of production store (mm), X2 is the groundwater exchange coefficient (mm); X3 is the 1 d ahead maximum capacity of routing store (mm) and X4 is the time peak ordinate of hydrograph unit (day) (Perrin et al., 2003). IHACRES model parameters: tw is the drying rate reference temperature [%], τs is the time constant for slow flow stor (day), τq is the time constant for quick flow stor (day) and f is temperature to potential evapotranspiration conversion factor (Andrews et al., 2011).
IHACRES model over the pre-dam have reliable value of KGE, NSE and R2 higher than 0.5 whereas the PBIAS are minimized in the same period. During the second period of post-dam period, KGE, NSE and R2 are only acceptable on the calibration (1989–1992) period while poor during the validation period (1993–1997) model performances criterions are insignificant. The parameters after optimization of the model respect the range at 95 % significant level as the value plotted in Table 2.
4.2 IHACRES and GR4J model simulated and observed runoff evolution dynamic
The graphs of Fig. 2 indicate the evolution of daily areal rainfall, simulated and observed runoff over calibration and validation periods both for the two models. The result shows that the observed and simulated runoff values predicted by GR4J and IHACRES models follow exactly the areal rainfall patterns but present some small differences. The GR4J model overestimates discharges whereas IHACRES models underestimates over the period of calibration (1965–1968) and period of validation (1969–1973) as shown in Fig. 2.
The two models accurately simulated base flow in the catchment. The lowest runoff periods (1971, 1972 and 1973) which can be explained by the period of drought and the periods of floods (1967 and 1958) in Togo and in West Africa (Koubodana et al., 2020; Oguntunde et al., 2006). The models simulated well base flow over between 1964 and 1986. IHACRES model during pre-dam simulation presents reliable value of KGE, NSE and R2 coefficients higher than 0.5 whereas and PBIAS are minimized in the same period.
During the second period of post-dam simulation the coefficients KGE, NSE and PBIAS are only acceptable on the calibration (1989–1992) period while poor during the validation period (1993–1997) as displayed in Fig. 3.
The model performances criterions are significant only for R2 criteria. The parameters after optimization of the model respect the range at 95 % significant level as the value plotted in Table 2. According to the results, during the pre-dam period predicted runoff and areal rainfall dynamic are similar and the simulated runoff from IHACRES and GR4J models matches with observed runoff during calibration (1965–1968) and validation (1969–1973) periods with R2, NSE and KGE coefficient between 0.6 and 0.87. The performances of GR4J models are seen to be reliable and robust than IHACRES model between 1964 and 1986. This can be explained by model parameterization, assumptions and number of the parameters in the models. Indeed GR4J model has four parameters while IHACRES model has more than four parameters (Andrews et al., 2011; Perrin et al., 2001). Difference between the hydrograph of the two sub periods (1964–1986) and (1988–2010) maybe due to many factors responsible of runoff generation in tropical zone. According to Dubreuil (1985), the generation of runoff and subsurface runoff depend on spatial variation of rainfall, soil surface formations, texture and structure, vegetation cover, geological layers, drainage density, weathered material and climate and even on Nangbéto dam management. Other factor is the Nangbéto dam management which affects surface runoff dynamic in the downstream of the reservoir and particularly at Athiémé gauge stations.
This study has assessed Mono catchment surface runoff using two rainfall–runoff models over the period before dam installation (1964–1986) and after dam installation (1988–2010). The lumped rainfall–runoff IHACRES and GR4J models were successfully calibrated and validated over the two sub-periods using multi-objectives functions. The results show that at daily time step, GR4J model overestimates the observation whereas IHACRES model underestimates observations during pre-dam installation period (1964–1986). However, over post-dam installation period (1988–2010), there is a weak prediction performance of observation with a shift by GR4J model while IHACRES model is underestimating observations. Study has deduced that runoff generation is not linear to rainfall variability in the catchment and maybe influences by anthropogenic and natural factors. Dam management has influences on surface runoff after the year 1987 and the catchment response depends strongly on the data used for the model used parameterization before or after Nangbéto dam installation in 1987. Study suggested a semi-distributed model for an accurate simulation of water components in the catchment for further analysis.
All climatic and hydrological data used in the research were collected from Togolese and Beninese Meteorological and hydrological services. The data can be obtained from DGMN-Togo, DGEA-Togo, METEO-Benin and DGEau.
HDK, KA, JGA and EA initiated the concept, HDK collected, analyzed the data and wrote the first manuscript. DJK, DK, KYA, YL and KEP contributed in the manuscript and KA, JGA and EA supervised the work.
The contact author has declared that neither they nor their co-authors have any competing interests.
Publisher's note: Copernicus Publications remains neutral with regard to jurisdictional claims in published maps and institutional affiliations.
This article is part of the special issue “Hydrology of Large River Basins of Africa”. It is a result of the 4th International Conference on the “Hydrology of the Great Rivers of Africa”, Cotonou, Benin, 13–20 November 2021.
The authors would like to thank the German Ministry of Education and Research (BMBF), IAHS SYSTA award funding to participate at the “4th International Conference on the Hydrology of African Large River Basins”, Academy of Sciences–Islamic Development Bank (TWAS–IsDB) Collaborative Programmes 2020/2021 for the support and Togolese and Beninese Meteorological and hydrological services for data provision.
This research has been supported by the Bundesministerium für Bildung und Forschung – BMBF (grant no. Batch-3) and World Academy of Sciences–Islamic Development Bank (TWAS–IsDB) Collaborative Programmes 2020/2021.
Adeaga, O., Mahe, G., Dieulin, C., Elbaz-Poulichet, F., Rouche, N., Seide, J. L., and Servat, E.: Rainfall–Runoff simulation in part of lower Niger basin, J. Environ. Sci. Eng. B, 1, 812–819, 2012.
Amoussou, E., Camberlin, P., and Mahé, G.: Impact de la variabilité climatique et du barrage Nangbéto sur l'hydrologie du système Mono-Couffo (Afrique de l'Ouest) l'hydrologie du système Mono-Couffo (Afrique de l'Ouest), Hydrolog. Sci. J., 57, 805–817, https://doi.org/10.1080/02626667.2011.643799, 2012.
Andrews, F. T., Croke, B. F. W., and Jakeman, A. J.: An open software environment for hydrological model assessment and development, Environ. Model. Softw., 26, 1171–1185, https://doi.org/10.1016/j.envsoft.2011.04.006, 2011.
Badjana, H. M., Fink, M., Helmschrot, J., Diekkrüger, B., Kralisch, S., Afouda, A. A., and Wala, K.: Hydrological system analysis and modelling of the Kara River basin (West Africa) using a lumped metric conceptual model, Hydrolog. Sci. J., 62, 1094–1113, https://doi.org/10.1080/02626667.2017.1307571, 2017.
CILSS: Landscapes of West Africa – A Window on a Changing World, Garretson, USA, 2016.
Dougherty, E. R., Kim, S., and Chen, Y.: Coefficient of determination in nonlinear signal processing, Sig. Process., 80, 2219–2235, 2000.
Dubreuil, P. L.: Review of Field Observations of Runoiff generation in the Tropics, J. Hydrol., 80, 237–264, 1985.
Gupta, H. V., Kling, H., Yilmaz, K. K., and Martinez, G. F.: Decomposition of the mean squared error and NSE performance criteria: Implications for improving hydrological modelling, J. Hydrol., 377, 80–91, https://doi.org/10.1016/j.jhydrol.2009.08.003, 2009.
Houessou, S.: Les inondataions et les risques prévisionnels liés aux barrages hydroéléctriques dans la basse vallée du Mono, These de doctorant, Soutenu publiquement le 11 Octobre 2016, Universite d'Abomey Calavi, Benin, 2016.
Kissi, A. E., Abbey, G. A., Agboka, K., and Egbendewe, A.: Quantitative Assessment of Vulnerability to Flood Hazards in Downstream Area of Mono Basin, South-Eastern Togo: Yoto District, J. Geogr. Inf. Syst., 7, 607–619, https://doi.org/10.4236/jgis.2015.76049, 2015.
Kodja, D. J., Mahé, G., Amoussou, E., Boko, M., and Paturel, J.-E.: Assessment of the Performance of Rainfall–Runoff Model GR4J to Simulate Streamflow in Ouémé Watershed at Bonou's outlet (West Africa), Preprints, 2018030090, 18 pp., https://doi.org/10.20944/PREPRINTS201803.0090.V1, 2018.
Komi, K., Amisigo, B. A., and Diekkrüger, B.: Integrated Flood Risk Assessment of Rural Communities in the Oti River Basin, West Africa, Hydrology, 3, 42, https://doi.org/10.3390/hydrology3040042, 2016.
Koubodana, H. D., Diekkrüger, B., Näschen, K., Adounkpe, J., and Atchonouglo, K.: Impact of the Accuracy of Land Cover Data Sets on the Accuracy of Land Cover Change Scenarios in the Mono River Basin, Togo, West Africa, Int. J. Adv. Remote Sens. GIS, 8, 3073–3095, https://doi.org/10.23953/cloud.ijarsg.422, 2019.
Koubodana, H. D., Adounkpe, J., Tall, M., Amoussou, E., Atchonouglo, K., and Mumtaz, M.: Trend Analysis of Hydroclimatic Historical Data and Future Scenarios of Climate Extreme Indices over Mono River Basin in West Africa, Am. J. Rural Dev., 8, 37–52, 2020.
Lawin, A. E., Hounguè, N. R., Biaou, C. A., and Badou, D. F.: Statistical Analysis of Recent and Future Rainfall and Temperature Variability in the Mono River Watershed (Benin, Togo), Climate, 7, 8, https://doi.org/10.3390/cli7010008, 2019.
Nash, J. E. and Sutcliffe, J. V.: River flow forecasting through conceptual models part I – A discussion of principles, J. Hydrol., 10, 282–290, 1970.
Ntajal, J., Lamptey, B. L., Mahamadou, I. B., and Nyarko, B. K.: Flood Disaster Risk Mapping in the Lower Mono River Basin in Togo, West, Int. J. Disast. Risk Reduct., 23, 93–103, https://doi.org/10.1016/j.ijdrr.2017.03.015, 2017.
Obahoundje, S., Diedhiou, A., Ofosu, E. A., Anquetin, S., François, B., Adounkpe, J., Amoussou, E., and Kouame, Y. M.: Assessment of Spatio-Temporal Changes of Land Use and Land Cover over South-Western African Basins and Their Relations with Variations of Discharges, Hydrology, 5, 56, https://doi.org/10.3390/hydrology5040056, 2018.
Oguntunde, P. G., Friesen, J., Van De Giesen, N., and Savenije, H. H. G.: Hydroclimatology of the Volta River Basin in West Africa: Trends and variability from 1901 to 2002, Phys. Chem. Earth Pt. A/B/C, 31, 1180–1188, https://doi.org/10.1016/j.pce.2006.02.062, 2006.
Oyerinde, G. T., Wisser, D., Hountondji, F. C. C., Odofin, A. J., Lawin, A. E., Afouda, A. A., and Diekkrüger, B.: Quantifying Uncertainties in Modeling Climate Change Impacts on Hydropower Production, Climate, 4, 1–15, https://doi.org/10.3390/cli4030034, 2016.
PCCP: PROGRAMME PCCP – From Potential Conflict to Cooperation Potential: cas du basin du Mono (Togo-Benin), Lomé, Togo, 2008.
Perrin, C., Michel, C., and Andre, V.: Does a large number of parameters enhance model performance? Comparative assessment of common catchment model structures on 429 catchments, J. Hydrol., 242, 275–301, 2001.
Perrin, C., Michel, C., and Andre, V.: Improvement of a parsimonious model for streamflow simulation, J. Hydrol., 279, 275–289, https://doi.org/10.1016/S0022-1694(03)00225-7, 2003.
Speth, P., Christoph, M., and Diekkrüger, B.: Impacts of Global Change on the Hydrological Cycle in West and Northwest Africa, Springer, Berlin, Heidelberg, https://doi.org/10.1007/978-3-642-12957-5_1, 2010.
Thiessen, A. H.: Precipitation averages for large areas, Mon. Weather Rev., 39, 1082–1089, 1911.
Thompson, J. R., Green, A. J., and Kingston, D. G.: Potential evapotranspiration-related uncertainty in climate change impacts on river flow: An assessment for the Mekong River basin, J. Hydrol., 510, 259–279, https://doi.org/10.1016/j.jhydrol.2013.12.010, 2014.
Vansteenkiste, T., Tavakoli, M., Van Steenbergen, N., De Smedt, F., Batelaan, O., Pereira, F., and Willems, P.: Intercomparison of five lumped and distributed models for catchment runoff and extreme flow simulation, J. Hydrol., 511, 335–349, https://doi.org/10.1016/j.jhydrol.2014.01.050, 2014.