the Creative Commons Attribution 4.0 License.
the Creative Commons Attribution 4.0 License.
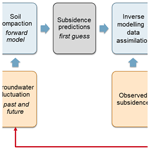
Towards regionally forecasting shallow subsidence in the Netherlands
Thibault Candela
Kay Koster
Jan Stafleu
Wilfred Visser
Peter Fokker
The Netherlands is subject to anthropogenically induced deep-source and shallow subsidence. Deep sources are related to the extraction of hydrocarbons or salt mining activities, whereas shallow subsidence comprise compaction, shrinkage and oxidation of clay and peat under progressive lowering groundwater levels. At TNO – Geological Survey of the Netherlands, deep-source and shallow subsidence are presently investigated separately. Forward and inverse modelling techniques are generally deployed to forecast subsidence caused by deep sources, whereas shallow subsidence is predicted using the high-resolution geological 3-D subsurface model GeoTOP. A new approach is proposed which encompasses forward and inverse modelling techniques and GeoTOP. Such combination will yield a powerful shallow subsidence forecasting model, which would be a critical step forward in analyzing shallow subsidence in the Netherlands on a regional scale.
In the present contribution, we sketch the setup of this new approach that combines subsidence measurements, GeoTOP subsurface data, and data assimilation of subsidence with the help of state-of-the-art forward and inverse modelling techniques. The setup uses ensemble technology to catch uncertainties of parameters, different model choices, and implicit correlations. With such a setup, forecasts can be faithfully accompanied with a quality measure that enables to judge its relevance and confidence range.
- Article
(1790 KB) - Full-text XML
- BibTeX
- EndNote
Anthropogenic subsidence processes in the Netherlands are divided into deep and shallow sourced, which are both in the order of up to 1 to 2 cm yr−1 (Van den Akker et al., 2008; Van Thienen-Visser and Fokker, 2017). Deep-sourced subsidence in the Netherlands is a result of the extraction of hydrocarbons and salt mining activities. During gas extraction, reservoir pressure decreases leading to its compaction. This reduction in volume at reservoir depth may induce surface subsidence (Doornhof, 1992). Shallow subsidence in the Netherlands is caused by the progressive lowering of groundwater levels, which results in compaction, shrinkage and oxidation of shallow clay and peat layers (Fokker et al., 2019). Besides anthropogenically induced subsidence, the Netherlands is also subjected to natural subsidence processes caused by tectonics and glacio-isostatic adjustments (Fokker et al., 2018). Tectonic subsidence is related to submergence of the North Sea basin which commenced during the Early Miocene (Zagwijn, 1989), whereas superimposed glacio-isostatic adjustments are in play since the Late Glacial Maximum (Vink et al., 2007).
On a regional scale however, the Netherlands is most under threat by shallow subsidence processes. These processes increase flood risks during storm-surges and high river water levels, damage infrastructures and houses, and cause saline water intrusions. Therefore, shallow subsidence poses large environmental and socioeconomic risks.
Prognoses indicate that the total costs related to shallow subsidence in the Netherlands are ca. EUR 20 billion for the period until 2050 (Van den Born et al., 2016). To limit these costs, forecasting subsidence is of utmost importance for policy makers, spatial planners and stakeholders. Such predictions can be used to identify areas most prone to subsidence and design mitigation strategies. Therefore, a model that faithfully forecasts shallow subsidence on a regional scale and constrains it using available data is of great value for such decision making.
In the field of subsurface information, TNO – Geological Survey of the Netherlands (TNO-GSN) has a unique position in the Netherlands, because it manages an exhaustive borehole dataset of the shallow subsurface, which is available in the high-resolution geological subsurface model GeoTOP. Furthermore, TNO-GSN has developed forward modelling and subsidence inversion techniques to forecast deep-source related subsidence. When combining the multiple expertise of TNO-GSN, a powerful novel approach can be developed that would be a critical step forward in understanding and forecasting shallow subsidence in the Netherlands. In this contribution, a way forward modelling workflow for the shallow subsidence predictions is proposed. This integrated workflow has been lacking so far in the Netherlands and even worldwide.
TNO-GSN recently developed an integrated approach, coined Ensemble-based Subsidence Interpretation and Prediction (ESIP) to take advantage of prior knowledge in terms of processes and subsurface parameters. In the context of Bayesian probability “Ensemble” here implies building multiple realizations of the subsidence based on the possible choices of processes and subsurface parameters. The objective is to carefully span the complete prior model space with our ensemble of subsidence realizations. Later, during an inversion step, the ensemble is confronted with the ground surface displacement data to refine our predictions.
Salt mining activities and hydrocarbons production in the Netherlands can both induce deformation (compaction) of the subsurface, and in response to it the ground surface subsides. Subsidence forecasting approaches are thus required for assessing the consequences of reservoir production and changes in it. However, the link between reservoir production and subsidence is non-trivial. As an example, for some gas fields in the Netherlands it has been observed that (i) a delay exists between the start of production and the onset of subsidence, (ii) the subsidence continued even after production has stopped, and (iii) the relationship between production and subsidence is non-linear (van Thienen-Visser et al., 2015). Thus, large uncertainties in the subsurface processes and related subsurface parameters imply that following a deterministic forecasting approach is not appropriate. Instead, and as in many scientific realms where uncertainties are abundant like weather forecasting, a probabilistic ensemble-based approach is an appropriate and fruitful way forward (e.g. Jaynes, 2003; Evensen, 2003; Reggiani and Weerts, 2008; Emerick and Reynolds, 2013).
It is worth noting here that ESIP can be used for subsidence predictions to support mitigation strategies, but also can be used as a probe of the reservoir activity at depth, and ultimately to reduce uncertainties with respect to the reservoir behavior in time and space. Indeed, besides the subsidence predictions, ESIP can be used to retrieve the driving input parameters of the subsurface and reservoir, like reservoir porosity, fault behavior, and aquifer strength.
Ensemble-based approaches for inversion of surface subsidence had already been developed in the past (see e.g. Baù et al., 2015; Candela et al., 2016; Fokker et al., 2012, 2016). However, these pioneering works were designed for specific applications, where only few specific model parameters were varied to build the prior ensemble of subsidence realizations. For example, Fokker et al. (2012) varied fault transmissibility only, because they were interested in detecting reservoir compartmentalization. In another study, Fokker et al. (2016) assumed a linear compaction model and only varied the reservoir compaction coefficient and the subsurface elastic moduli. Furthermore, Baù et al. (2015) focused on the general reservoir and geomechanical parameters like pressure drop, reservoir location and size, elastic parameters, and compressibility. With ESIP, we can take into consideration most (if not all) of the physical processes and parameters attached to them for modelling subsidence due to reservoir gas production.
The 3-D geological subsurface model GeoTOP has a x, y, z resolution of m and schematizes the subsurface to a depth of 50 m b.m.s.l. (Fig. 1) (Stafleu et al., 2011). Each voxel in the model contains most likely estimates of the stratigraphical unit and the lithological class (Fig. 1). At present, GeoTOP comprises 12 model areas. It is based on ca. 455 000 coded borehole descriptions from the national subsurface DINO database operated by TNO-GSN, complemented with 125 000 borehole logs from Utrecht University. The six model areas in the southwest, west and north of the country are characterized by a thick Holocene coastal wedge that is underlain by a stack of Pleistocene sandy units. The Holocene sequence includes thick occurrences of peat and clay, which makes GeoTOP the de facto standard in predictive land subsidence studies (Van der Meulen et al., 2013).
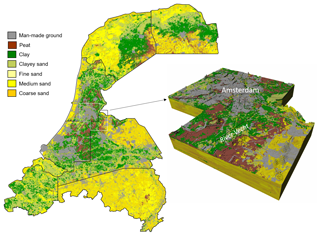
Figure 1GeoTOP illustration of the Netherlands (left) and an enlarged area around Amsterdam (right).
Recent subsidence studies deploying GeoTOP focused on past peat compaction (Koster et al., 2018a), peat oxidation potential (Koster et al., 2018b), and scenarios of near future subsidence in urbanized and agricultural peat areas (Koster et al., 2018c). However, parameterizing peat and clay voxels on a regional scale with properties essential to forecast compaction and oxidation under changing groundwater levels remains challenging. For single site locations, progression was made by TNO-GSN by applying an inversion workflow to constrain and estimate clay and peat properties from ca. 50 years of monitored subsidence (Fokker et al., 2019). This approach is generally applied to deep-source subsidence studies (see Sect. 2 of the present contribution), and engaging it to unravel shallow subsidence sources was therefore an innovative approach.
TNO-GSN manages a national database for subsurface information (DINO). This database contains borehole information, geotechnical and geophysical soundings, and groundwater monitoring data. This dataset comprises measurements dating back to the late 19th century. For constraining ESIP this information is essential, because groundwater level lowering resulting in volumetric loss of subsurface layers is reflected in the thickness reduction of documented thicknesses of peat and clay layers in DINO (Vonhögen et al., 2010).
We propose to use the newly developed ESIP probabilistic TNO-GSN workflow in the context of shallow subsidence on a regional scale. More specifically, the probabilistic ESIP workflow combined with GeoTOP and present-day subsidence measurements is essential to identify and quantify the contribution of each subsidence process, and to support quality decisions in terms of future groundwater management. The main steps of the proposed workflow are presented in Fig. 2.
The first step consists of combining ESIP with GeoTOP to produce subsidence forward models. This encompasses populating GeoTOP voxels with estimates of peat and clay parameters. These parameters are derived from samples that have been collected during TNO-GSN's nationwide mapping campaign initiated in 2006. At present, this campaign yields a few hundred of 30m continuous cores spread throughout GeoTOP's model areas. The cores have been intensively sampled for mechanical, chemical, and hydrological analysis. One can consider these first subsidence forward models as “blind predictions”, and most likely their predictive power is limited due to a wide confidence interval. Up to now we covered the first 3 boxes of the workflow in Fig. 2. It is now required to cross-check these forward models against the observed subsidence during the data assimilation step; this corresponds to the box “inverse modelling/data assimilation” in Fig. 2. After leveraging the information content of the data, new refined subsidence predictions and their uncertainty band can be generated (box “Subsidence predictions – refined guess with uncertainty band” in Fig. 2).
The second step consists of using scenarios of groundwater level changes to forecast future subsidence with parameters derived from the first step. Groundwater level information can be retrieved from the DINO database (see Sect. 4). Ultimately, the newly generated subsidence predictions can help to optimize the groundwater management strategy on a regional scale.
The subsidence measurements will be selected based on to be selected sub-areas of interest; the GeoTOP model can be accessed via at https://www.dinoloket.nl/en (last access: November 2019) (TNO-GSN, 2019).
All authors contributed equally to the design of the workflow to regionally forecast shallow subsidence in the Netherlands. TC and KK drafted the manuscript.
The authors declare that they have no conflict of interest.
This article is part of the special issue “TISOLS: the Tenth International Symposium On Land Subsidence – living with subsidence”. It is a result of the Tenth International Symposium on Land Subsidence, Delft, the Netherlands, 17–21 May 2021.
Baù, D., Ferronato, M., Gambolati, G., Teatini, P., and Alzraiee, A.: Ensemble smoothing of land subsidence measurements for reservoir geomechanical characterization, Int. J. Num. Anal. Meth. Geomech., 39, 207–228, 2015.
Candela, T., Osinga, S., van der Veer, E. F., ter Heege, J. H., and Fokker, P.: Improving Reservoir Exploitation Using Fast Geomechanical Modelling Coupled with Surface Displacement Data Assimilation, in: SPE Reservoir Characterisation and Simulation Conference and Exhibition, SPE-186028-MS, 8–10 May 2016, Abu Dhabi, 2016.
Doornhof, D.: Surface subsidence in The Netherlands: The Groningen gas field, Geologie en Mijnbouw, 71, 119–130, 1992.
Emerick, A. A. and Reynolds, A. C.: Ensemble smoother with multiple data assimilation, Comput. Geosci., 55, 3–15, 2013.
Evensen, G.: The Ensemble Kalman Filter: theoretical formulation and practical implementation, Ocean Dynam., 53, 343–367, 2003.
Fokker, P. A., Visser, K., Peters, E., Kunakbayeva, G., and Muntendam-Bos, A. G.: Inversion of surface subsidence data to quantify reservoir compartmentalization: A field study, J. Petrol. Sci. Eng., 96, 10–21, 2012.
Fokker, P. A., Wassing, B. B. T., Van Leijen, F. J., Hanssen, R. F., and Nieuwland, D. A.: Application of an ensemble smoother with multiple data assimilation to the Bergermeer gas field, using PS-InSAR, Geom. Energ. Environ., 5, 16–28, 2016.
Fokker, P. A., van Leijen, F. J., Orlic, B., van der Marel, H., and Hanssen, R. F.: Subsidence in the Dutch Wadden Sea, Neth. J. Geosci., 97, 129–181, 2018.
Fokker, P. A., Gunnink, J. L., Koster, K., and De Lange, G.: Disentangling and parameterizing shallow sources of subsidence: application to a reclaimed coastal area, Flevoland, the Netherlands, J. Geophys. Res.-Earth, 124, 1099–1117, 2019.
Jaynes, E. T.: Probability theory. The logic of science, Cambridge University Press, Cambridge, 2003.
Koster, K., Cohen, K. M., Stafleu, J., and Stouthamer, E.: Using 14C-dated peat beds for reconstructing subsidence by compression in the Holland coastal plain of the Netherlands, J. Coast. Res., 34, 1035–1045, 2018a.
Koster, K., Stafleu, J., Cohen, K. M., Stouthamer, E., Busschers, F. S., and Middelkoop, H.: Three-dimensional distribution of organic matter in coastal-deltaic peat: Implications for subsidence and carbon dioxide emissions by human-induced peat oxidation, Anthropocene, 22, 1–9, 2018b.
Koster, K., Stafleu, J., and Stouthamer, E.: Differential subsidence in the urbanized coastal-deltaic plain of the Netherlands, Neth. J. Geosci., 97, 215–227, 2018c.
Reggiani, P. and Weerts, A. H.: A Bayesian approach to decision-making under uncertainty: an application to real-time forecasting in the river Rhine, J. Hydrol., 356, 56–69, 2008.
Stafleu, J., Maljers, D., Gunnink, J. L., Menkovic, A., and Busschers, F. S.: 3D modeling of the shallow subsurface of Zeeland, the Netherlands, Neth. J. Geosci., 90, 293–310, 2011.
TNO-GSN: Online portal for digital geo-information, Geological Survey of the Netherlands, available at: https://www.dinoloket.nl/en, last access: November 2019.
Van den Akker, J. J. H., Kuikman, P. J., de Vries, F., Hoving, I., Pleijter, M., Hendriks, R. F. A., Wolleswinkel, R. J., Simões, R. T. L., and Kwakernaak, C.: Emission of CO2 from agricultural peat soils in the Netherlands and ways to limit this emission, edited by: Farrell, C. and Feehan, J., in: Vol. 1 Oral Pres., Proc. of the 13th Int. Peat Congr. After Wise Use – The Future of Peatlands, 8–13 June 2008, Tullamore, Ireland, Int. Peat Soc., Jyväskylä, Finland, 645–648, 2008.
Van den Born, G. J., Kragt, F., Henkens, D., Rijken, Van Bemmel, B., and Van der Sluis, S.: Dalende bodem, stijgende kosten, PBL, Den Haag, 94 pp., 2016.
Van der Meulen, M. J., Doornenbal, J. C., Gunnink, J. L., Stafleu, J., Schokker, J., Vernes, R. W., Van Geer, F. C., Van Gessel, S. F., Van Heteren, S., Van Leeuwen, R. J. W., Bakker, M. A. J., Bogaard, P. J. F., Busschers, F. S., Griffioen, J., Gruijters, S. H. L. L., Kiden, P., Schroot, B. M., Simmelink, H. J., Van Berkel, W. O., Van der Krogt, R. A. A., Westerhoff, W. E., and Van Daalen, T. M.: 3D Geology in a 2D country: perspectives for geological surveying in the Netherlands, Neth. J. Geosci., 92, 217–241, 2013.
Van Thienen-Visser, K. and Fokker, P. A.: The future of subsidence modelling: compaction and subsidence due to gas depletion of the Groningen gas field in the Netherlands, Neth. J. Geosci., 96, 105–116, 2017.
van Thienen-Visser, K., Pruiksma, J. P., and Breunese, J. N.: Compaction and subsidence of the Groningen gas field in the Netherlands, Proc. IAHS, 372, 367–373, https://doi.org/10.5194/piahs-372-367-2015, 2015.
Vink, A., Steffen, H., Reinhardt, L., and Kaufmann, G.: Holocene relative sea-level change, isostactic subsidence and the radial viscosity structure of the mantle of northwest Europe (Belgium, the Netherlands, Germany, southern North Sea), Quaternary Sci. Rev., 26, 3249–3275, 2007.
Vonhögen, L. M., Doornebal, P. J., De Lange, G., Fokker, P. A., and Gunnink, J. L.: Subsidence in the Holocene delta of the Netherlands, Proc. IAHS, 339, 158–163, 2010.
Zagwijn, W. H.: The Netherlands during the Tertiary and Quaternary: a case history of coastal lowlands evolution, Geologie en Mijnbouw, 68, 107–121, 1989.