the Creative Commons Attribution 4.0 License.
the Creative Commons Attribution 4.0 License.
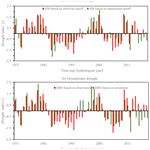
Modelling human impacts on surface and subsurface hydrological drought
Tesfaye Belay Senbeta
Krzysztof Kochanek
Emilia Karamuz
Jaroslaw Jan Napiorkowski
The scientists tend to assume that climatic factors are the only drivers in the development of drought. However, this classical assumption is no longer valid in the Anthropocene, as most catchments have been significantly modified by human factors. Recent theoretical developments have shown that there are mutual interactions and feedback mechanisms – drought shapes humans and vice versa. Therefore, this study aims to assess the role of human impacts on hydrological drought (HD) characteristics by considering runoff and baseflow separately. For this purpose, the two rainfall-runoff models: SWAT (Soil and Water Assessment Tool) and HBV (Hydrologiska Byrans Vattenbalansavdelning) models are compared. The results show significant differences in the magnitude of change between the two models, suggesting that the ensemble method of multiple models need to be considered for modelling human impact. However, the results confirm that despite significant differences in the magnitude of change, both hydrological models indicate similar impacts, i.e. positive and negative.
- Article
(1451 KB) - Full-text XML
- BibTeX
- EndNote
Human factors; Panta Rhei; observation-modelling framework; UPH9; SWAT; HBV; hydrological drought
Catchment responses to precipitation inputs depend on geophysical characteristics, climatic conditions, and human factors (Kubiak-Wójcicka and Bąk, 2018). These factors affecting hydrological processes are mainly divided into two categories: natural factors (climatic factors, watershed characteristics) and human factors (Harrigan et al., 2014; Jiang et al., 2015). Watershed characteristics include watershed size, terrain slope, natural vegetation, geology, orientation, and prevailing soil moisture conditions, while human factors include development of irrigation, water transfer, water abstraction and land cover dynamics (B. Liu et al., 2016). Local climate variability and its impact on droughts have increased and are likely to increase further due to the release of greenhouse gases that have caused global warming (Kang and Sridhar, 2017). In northern Europe, human-modified climate change is likely to be responsible for recent drought (Gudmundsson and Seneviratne, 2016). Frequent drought is also a serious problem for the environment and socio-economic situation in Poland (Bąk and Kubiak-Wójcicka, 2017), with severe droughts occurring frequently in the central part of the River Vistula basin (Karamuz et al., 2021; Bąk and Kubiak-Wójcicka, 2017). Studies have also identified the impact of human factors on HD as either exacerbating (Wanders and Wada, 2015) or ameliorating (Jiao et al., 2020). Human factors impact the development of HD, by accumulating, attenuating, delaying, prolonging, etc. (Y. Liu et al., 2016). Which of these factors – climate or human – determines the characteristics of HD depends on the spatial and temporal scales considered for studies. This means that climate is mainly the dominant factor at the global level and on a longer-term scale (Y. Liu et al., 2016), while human factors are mainly dominant at the local level and on a short-term scale (Zou et al., 2017). Therefore, site-specific water management measures are recommended to address the different HD problems in different river reaches (Qiu et al., 2016).
A drought is generally when available water (i.e. precipitation, soil moisture, surface water, and groundwater) is significantly scarce for an extended period. The overall impact on the components of the hydrological cycle can lead to a long-term socio-economic drought, which in turn leads to water scarcity, i.e. an imbalance between water demand and available water supply (van Loon et al., 2016a). The study of drought has a long tradition of assuming that climatic factors are the only driving factors for its development (Nasiri et al., 2022). However, this classical assumption is no longer valid in the Anthropocene, in which the Earth's systems have been significantly modified by human impacts (van Loon et al., 2016b). This led to a redefinition of drought by van Loon et al. (2016b) that takes human responses into account. The existence of interactions between drought impacts and human responses suggests a reciprocal influence – drought impacts shape human societies and vice versa (Di Baldassarre et al., 2017). Moreover, the IAHS has proclaimed the period 2013–2022 as the Scientific Decade, which will focus on research into the interactions and feedbacks between humans and the environment under the title “Panta Rhei – everything flows” (Montanari et al., 2013). Furthermore, Blöschl et al. (2019) identified the occurrence of droughts and their changes as an “Unsolved Problem in Hydrology” (UPH9), which states: “How do droughts occur, do they change and if so, why?”.
Although the studies on droughts are not new, the dynamics of drought impacts and human responses, as well as the modelling of this feedback, have not been sufficiently explored (Di Baldassarre et al., 2017). For instance, Wu et al. (2019) and Rangecroft et al. (2019) have used total runoff to determine HD, while other studies consider HD by looking separately at the components of the hydrological process, particularly baseflow drought (e.g. Bazrkar and Chu, 2020). Our study, therefore, focuses on assessing the impact of human factors on HD characteristics considering (sub)surface runoff separately. Two rainfall-runoff (RR) models: SWAT (Arnold et al., 1998) and HBV (Bergström and Forsman, 1973) were applied for reconstructing (simulating) the “proxy” natural runoff during the human-modified period, which was compared with the observed runoff (human-modified runoff) by applying the observation – modelling framework proposed by van Loon and van Lanen (2013). Naturalized and observed runoff and the corresponding filtered baseflow were used as indicators of HD.
The aim of this study is therefore to model the impact of human factors on the characteristics of HD by comparing simulation-based and observed-based using standardized HD indices. We also considered two RR models of different complexity, the lumped version of the HBV model and the semi-distributed SWAT model, to determine the role of using different RR models of different complicities in identifying HD characteristics.
2.1 Study area
The Kamienna catchment is located in Central Poland, with a catchment area of about 2000 km2, and river length of about 156 km. It is a tributary of the Vistula River basin and lies at geographical coordinates between 20.60–21.70° E and 50.79–51.22° N (Fig. 1). The topography of the catchment ranges from about 130 m to just over 600 m (Senbeta and Romanowicz, 2021), with a mean elevation of 260 m above mean sea level. The catchment has experienced the greatest change in land cover from agriculture to forests and natural areas, as well as a significant change in the hydrological regime (Senbeta and Romanowicz, 2021).
2.2 Data
Two rainfall-runoff (RR) models were used in our study: the SWAT and the HBV models with different complexity and input data requirements. Climate variables such as precipitation and temperature for the period 1968–2018 were used for both models, while additional spatial GIS data (topography, land use, and soil map) were used for the SWAT model; see Senbeta and Romanowicz (2021) for more details. Potential evapotranspiration (Eo) was calculated externally with the temperature-based method for the HBV model. Precipitation and Eo at each station were then aggregated to catchment areal-average value using the Thiessen polygon and used as input to the HBV model. River runoff at the Czekarzewice gaging station was also used as an additional input for model calibration, validation, and further HD analysis. The periods 1971–1976 and 1977–1982 were used for model calibration and validation for both models, while the entire period, 1971–2018, was used for HD analysis.
2.3 Human impacts modelling approach
The study applied “observational-modelling” comparative approach (van Loon and van Lanen, 2013) that compares observed river runoff (i.e. human-modified runoff) with simulated (naturalized) runoff using the RR models to determine the human impact on the hydrological processes. In this approach, the observed runoff is considered as an indicator of the combined impacts of human and climatic factors, while the simulated runoff is an indicator of only the impact of climate variability. This means that the difference between the observed and simulated runoff is an indicator of human influence on river runoff. The selected RR models – the SWAT and HBV models – calibrated and validated in the reference (natural conditions) period (1971–1982) were applied to model natural runoff during the human period (1983–2018). This significant change in the river runoff regime in the early 1980s in the Kamienna catchment was noted in an earlier study (Senbeta and Romanowicz, 2021). Details on the setup, calibration, and validation of the SWAT can also be found in Senbeta and Romanowicz (2021), while details on the HBV modelling processes are described in Sect. 2.4.
The study also aims to consider baseflow as a proxy indicator for groundwater drought, but no observed baseflow data were available throughout the study period. Therefore, the baseflow data were filtered out from the observed and RRs simulated runoff using the Wittenberg algorithm (Wittenberg, 1999). This baseflow filtering method was proposed based on a non-linear reservoir algorithm and the calculation is done backwards, starting with the last value of the baseflow recession as follows:
where Qo is an initial discharge [mm d−1], Qt is a baseflow recession at time t, and a is a parameter with the dimension [mm1−b db]; b is a dimensionless exponent [0.5], Δt is a time step [day].
2.4 HBV model
The HBV model (Bergström and Forsman, 1973), originally developed by the Swedish Meteorological and Hydrological Institute is a rainfall-runoff model that simulates river runoff at daily time scales. The conceptual model consists of three reservoirs: the soil box, the upper layer, and the lower layer, representing respectively the soil moisture, the fast response of the subsurface, and the slow response of the groundwater. The model uses the degree-day method to simulate snowmelt. The model is lumped when a single input is used for the entire catchment, while it is considered semi-distributed when a catchment is divided into sub-catchments based on elevation and vegetation zones. In the study, the lumped version of HBV-Light was used. For more details on this version of the model, Seibert and Vis (2012), is recommended.
2.5 Hydrological drought identification method
The parametric standardized approach was applied to determine the Streamflow Drought Index (SDI; Nalbantis and Tsakiris, 2009) and the Standardized Baseflow Index (SBFI; Bazrkar and Chu, 2020) as indicators of surface and subsurface HD, respectively. In this approach, both drought indices (DI) are derived based on the Log-normal distribution function. The HD characteristics were derived from DI, based on the run theory with the threshold level (TL) of −1. This means that a DI of less than −1 indicates drought, while a value above −1 indicates either mild (normal) drought or wet conditions. Furthermore, drought can be classified into three states: moderate (), Severe () and extreme () (Nalbantis and Tsakiris, 2009). The number of months for a particular drought event is referred to as the duration of drought (DD), the sum of the DI values for a single drought event indicates the severity of the drought (S), and the ratio of S to DD indicates the intensity of the drought (I) (Mckee et al., 1993).
The change in the characteristics of HD is quantified by comparing observation-based and simulation-based results as follows:
where ΔHDC refers to change in HD characteristics [%], HDIO refers to observation-based HD index (SDI/SBFI), and HDIN refers to simulation-based (naturalized) HD index (SDI/SBFI).
3.1 Comparison of the RR models in HD analysis
Changes in HD characteristics based on SWAT and HBV-based runoff are compared with observation-based runoff to model human impacts, as shown in Table 1.
The duration of drought (DD), the severity of the drought (S), the number of drought events (ND), and the intensity of the drought (I) changed by 47.9 %, 19.3 %, 70.0 %, and −19.3 % based on the SWAT model and by 17.4 %, 9.9 %, 21.4 %, −6.5 % based on the HBV model, respectively, indicating that there are significant differences in human impacts on HD characteristic between the two RR models. Even though the magnitude of human impacts differs significantly between the two models, both models show the same direction of human impacts, i.e. either positive or negative changes for each of the considered HD characteristic.
Table 1Change in hydrological drought characteristics based on streamflow drought index (SDI).
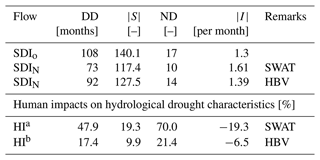
Note. DD = drought duration, S = drought severity, ND = number of drought events, I = drought intensity, SDIN = Naturalized-based streamflow drought index, SDIO = Observation-based streamflow drought index, a Human impact based on the SWAT model simulation, b Human impact based on the HBV model simulation
3.2 Hydrological drought analysis
The HD indices were calculated based on the observed and naturalized runoff and the respective filtered baseflow to determine the change in the characteristics of HD using the TL value of −1. The results show that 17 HD events in different drought categories (i.e., moderate, severe, or extreme) occurred during the human-influenced period, which is more than the 12 HD events for naturalized conditions. Due to the significantly different results found between the SWAT and HBV models (Sect. 3.1), the study used the arithmetic mean of the two RR models for further analysis of human impacts on HD. The HD events of 1973–1974, 1984–1985, 1989, 1990, 1992, 2006, 2007, and 2008 were sustained streamflow droughts of more than 5 consecutive months based on observations, while the HD events of 1973–1974, 1984–1985, 1989–1990, and 2012–2013 were streamflow droughts of more than 5 months under naturalized conditions. Moreover, the results show variations in the magnitude of drought severity between natural and human-modified conditions for both surface and subsurface HD (Fig. 2). The early 1980s and 1990s were the most severely affected decades with successive HD events of longer DD (15 and 17 months) and I (−1.4 and −1.47 intense per month) based on observed runoff (not shown). Unlike the other decades considered in this study, the results for the 2010s decade show a greater number of HD events for the naturalized condition than for the observation-based analysis.
During the perturbed period (1983–2018), HD characteristics such as ND events, mean DD, and S increased by 83.1 %, 18.5 %, and 5.6 % for observation-based runoff and 25.3 %, 39.3 %, and 41.6 % for observation-based baseflow as compared to the baseline period (1971–1982) (not shown).
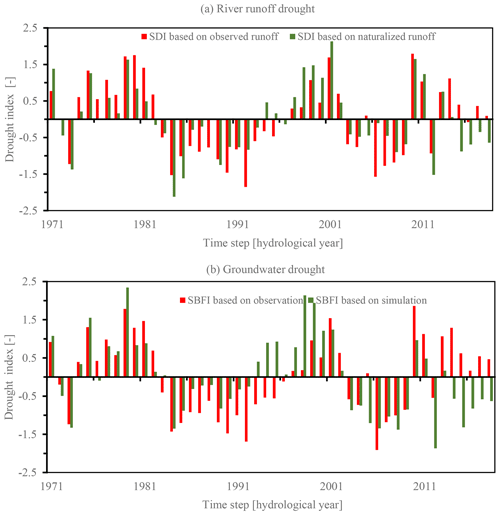
Figure 2Hydrological drought analysis (a) Streamflow Drought Index (SDI); (b) the Standardized Baseflow Index (SBFI).
The result also shows that the frequency of HD increases from 12.2 % to 19.1 % due to the influence of human interventions (Table 2), indicating a 6.9 % increase in HD frequency.
In this study, two RR models (SWAT and HBV) were applied for modelling the impacts of humans on the characteristics of HD, using the “observational-modelling” comparison approach. A comparison of the RR models revealed that naturalized runoff based on SWAT and HBV produced significantly different results in quantifying changes in HD characteristics (Table 1). Obviously, the differences in results were generally expected, but contrary to expectations, too large differences, e.g., up to 30 % and 50 % attribution of human impacts on surface HD characteristics for DD and ND events shown between the two models, respectively. This implies that the percentage change of HD characteristics in this approach depends on the RR models applied, their performance during calibration and validation (van Loon and van Lanen, 2013), and the ability of the model to simulate runoff during the dry season. However, the study confirms that a similar direction of impact for all HD characteristics is achieved, i.e. positive changes (worsening of drought) or negative (weakening of drought). The negative value of drought intensity (I) could be due to the buffering impact that human interventions (such as the release of water reservoirs) have during the DD. It is also worth noting that, human-modified conditions reduced the maximum duration of drought as well as the number of drought events in the 2010s. This is related to the fact that the Wiory reservoir, the largest reservoir in the study area, was put into operation a few years later. Reservoirs increases in baseflow index (BFI) in long-term, which is a positive effect of water storage (Marcinkowski and Grygoruk, 2017; Raczyński, 2020). However, the applied approach suffers from the limitation that observed and simulated runoff were compared, which contains different uncertainties, and that only two RR models are used. Therefore, reconstruction (simulation) of observed runoff during the human-modified period (Nasiri et al., 2022) and multi-model ensemble methods and/or integrated surface-subsurface hydrological models are recommended for the future to overcome the respective limitations.
This study has evidently shown that modelling human impacts on HD with two RR models – the SWAT and the HBV model – of different complexity led to significant differences, although both models indicate similar impacts, i.e. positive or negative. We, therefore, conclude that modelling human impacts on HD based on comparing observations and simulations by applying multi-models need be considered in the future to draw reliable conclusions from the results. Future research on human influence modelling could therefore extend this approach by considering an ensemble of multiple models. In addition, further research is needed to confirm this new finding by applying the method to different case studies.
The SWAT and HBV models were utilized in this research. The source code for both models is open-source and can be downloaded from the following websites: https://swat.tamu.edu/software/arcswat/ (TAMU, 2021) and https://www.geo.uzh.ch/en/units/h2k/Services/HBV-Model/HBV-Download.html (UZH, 2021). The version of SWAT used in this research was ArcSWAT 2012.10_7.24 and the version of HBV was the HBV-light.
The hydroclimatic variables used in this study were obtained from the Institute of Meteorology and Water Management (IMGW-PIB). The topographic and soil data used in this study were prepared by Piniewski et al. (2017), and the land use data was obtained from Corine data (https://land.copernicus.eu/pan-european/corine-land-cover, Copernicus, 2020).
Conceptualization: TBS, KK, EK and JJN; methodology: TBS, KK, EK and JJN; data curation: TBS and EK; writing – original draft preparation: TBS; writing – review and editing: TBS, KK, EK and JJN; supervision: KK and EK; funding acquisition: JJN. All authors have read and agreed to the published version of the manuscript.
The contact author has declared that none of the authors has any competing interests.
Publisher's note: Copernicus Publications remains neutral with regard to jurisdictional claims in published maps and institutional affiliations.
This article is part of the special issue “IAHS2022 – Hydrological sciences in the Anthropocene: Variability and change across space, time, extremes, and interfaces”. It is a result of the XIth Scientific Assembly of the International Association of Hydrological Sciences (IAHS 2022), Montpellier, France, 29 May–3 June 2022.
This work is part of the HUMDROUGHT project conducted at the Institute of Geophysics of the Polish Academy of Sciences. The project website can be found at https://humdrought.igf.edu.pl/ (last access: 4 April 2023). We would like to thank the Institute of Meteorology and Water Management (IMGW-PIB) for providing hydroclimatic data. We also thank the two anonymous reviewers and the Guest Editor of Proceedings of the International Association of Hydrological Sciences, Christophe Cudennec, for all valuable comments and suggestions that helped us to improve the quality of the manuscript.
This research has been supported by the National Science Center (grant no. 2018/30/Q/ST10/00654).
This paper was edited by Christophe Cudennec and reviewed by two anonymous referees.
Arnold, J. G., Srinivasan, R., Muttiah, R. S., and Williams, J. R.: Large area hydrologic modeling and assessment part I: model development, Journal of the Americal Water Resources Association, 34, 73–89, 1998.
Bąk, B. and Kubiak-Wójcicka, K.: Impact of meteorological drought on hydrological drought in Toruń (central Poland) in the period of 1971–2015, Journal of Water and Land Development, 32, 3–12, https://doi.org/10.1515/jwld-2017-0001, 2017.
Di Baldassarre, G., Martinez, F., Kalantari, Z., and Viglione, A.: Drought and flood in the Anthropocene: feedback mechanisms in reservoir operation, Earth Syst. Dynam., 8, 225–233, https://doi.org/10.5194/esd-8-225-2017, 2017.
Bazrkar, M. H. and Chu, X.: New Standardized Base Flow Index for Identification of Hydrologic Drought in the Red River of the North Basin, Nat. Hazards Rev., 21, 05020011, https://doi.org/10.1061/(asce)nh.1527-6996.0000414, 2020.
Bergström, S. and Forsman, A.: Development of a conceptual deterministic rainfall-runoff model, Hydrol. Res., 4, 147–170, https://doi.org/10.2166/nh.1973.0012, 1973.
Blöschl, G., Bierkens, M. F. P., Chambel, A., Cudennec, C., Destouni, G., Fiori, A., Kirchner, J. W., McDonnell, J. J., Savenije, H. H. G., Sivapalan, M., Stumpp, C., Toth, E., Volpi, E., Carr, G., Lupton, C., Salinas, J., Széles, B., Viglione, A., Aksoy, H., Allen, S. T., Amin, A., Andréassian, V., Arheimer, B., Aryal, S. K., Baker, V., Bardsley, E., Barendrecht, M.H., Bartosova, A., Batelaan, O., Berghuijs, W. R., Beven, K., Blume, T., Bogaard, T., Borges de Amorim, P., Böttcher, M. E., Boulet, G., Breinl, K., Brilly, M., Brocca, L., Buytaert, W., Castellarin, A., Castelletti, A., Chen, X., Chen, Y., Chen, Y., Chifflard, P., Claps, P., Clark, M. P., Collins, A. L., Croke, B., Dathe, A., David, P. C., de Barros, F. P. J., de Rooij, G., Di Baldassarre, G., Driscoll, J. M., Duethmann, D., Dwivedi, R., Eris, E., Farmer, W. H., Feiccabrino, J., Ferguson, G., Ferrari, E., Ferraris, S., Fersch, B., Finger, D., Foglia, L., Fowler, K., Gartsman, B., Gascoin, S., Gaume, E., Gelfan, A., Geris, J., Gharari, S., Gleeson, T., Glendell, M., Gonzalez Bevacqua, A., González-Dugo, M. P., Grimaldi, S., Gupta, A. B., Guse, B., Han, D., Hannah, D., Harpold, A., Haun, S., Heal, K., Helfricht, K., Herrnegger, M., Hipsey, M., Hlaváčiková, H., Hohmann, C., Holko, L., Hopkinson, C., Hrachowitz, M., Illangasekare, T. H., Inam, A., Innocente, C., Istanbulluoglu, E., Jarihani, B., Kalantari, Z., Kalvans, A., Khanal, S., Khatami, S., Kiesel, J., Kirkby, M., Knoben, W., Kochanek, K., Kohnová, S., Kolechkina, A., Krause, S., Kreamer, D., Kreibich, H., Kunstmann, H., Lange, H., Liberato, M. L. R., Lindquist, E., Link, T., Liu, J., Loucks, D. P., Luce, C., Mahé, G., Makarieva, O., Malard, J., Mashtayeva, S., Maskey, S., Mas-Pla, J., Mavrova-Guirguinova, M., Mazzoleni, M., Mernild, S., Misstear, B. D., Montanari, A., Müller-Thomy, H., Nabizadeh, A., Nardi, F., Neale, C., Nesterova, N., Nurtaev, B., Odongo, V. O., Panda, S., Pande, S., Pang, Z., Papacharalampous, G., Perrin, C., Pfister, L., Pimentel, R., Polo, M. J., Post, D., Prieto Sierra, C., Ramos, M. H., Renner, M., Reynolds, J. E., Ridolfi, E., Rigon, R., Riva, M., Robertson, D. E., Rosso, R., Roy, T., Sá, J. H. M., Salvadori, G., Sandells, M., Schaefli, B., Schumann, A., Scolobig, A., Seibert, J., Servat, E., Shafiei, M., Sharma, A., Sidibe, M., Sidle, R. C., Skaugen, T., Smith, H., Spiessl, S. M., Stein, L., Steinsland, I., Strasser, U., Su, B., Szolgay, J., Tarboton, D., Tauro, F., Thirel, G., Tian, F., Tong, R., Tussupova, K., Tyralis, H., Uijlenhoet, R., van Beek, R., van der Ent, R. J., van der Ploeg, M., Van Loon, A. F., van Meerveld, I., van Nooijen, R., van Oel, P. R., Vidal, J. P., von Freyberg, J., Vorogushyn, S., Wachniew, P., Wade, A. J., Ward, P., Westerberg, I. K., White, C., Wood, E. F., Woods, R., Xu, Z., Yilmaz, K. K., and Zhang, Y.: Twenty-three unsolved problems in hydrology (UPH) – a community perspective, Hydrolog. Sci. J., 64, 1141–1158, https://doi.org/10.1080/02626667.2019.1620507, 2019.
Copernicus: CORINE Land Cover, Copernicus [data set], https://land.copernicus.eu/pan-european/corine-land-cover, last access: 4 November 2020.
Gudmundsson, L. and Seneviratne, S. I.: Anthropogenic climate change affects meteorological drought risk in Europe, Environ. Res. Lett., 11, 044005, https://doi.org/10.1088/1748-9326/11/4/044005, 2016.
Harrigan, S., Murphy, C., Hall, J., Wilby, R. L., and Sweeney, J.: Attribution of detected changes in streamflow using multiple working hypotheses, Hydrol. Earth Syst. Sci., 18, 1935–1952, https://doi.org/10.5194/hess-18-1935-2014, 2014.
Jiang, C., Xiong, L., Wang, D., Liu, P., Guo, S., and Xu, C. Y.: Separating the impacts of climate change and human activities on runoff using the Budyko-type equations with time-varying parameters, J. Hydrol. (Amst), 522, 326–338, https://doi.org/10.1016/j.jhydrol.2014.12.060, 2015.
Jiao, D., Wang, D., and Lv, H.: Effects of human activities on hydrological drought patterns in the Yangtze River Basin, China, Nat. Hazards, 104, 1111–1124, https://doi.org/10.1007/s11069-020-04206-2, 2020.
Kang, H. and Sridhar, V.: Combined statistical and spatially distributed hydrological model for evaluating future drought indices in Virginia, J. Hydrol. Reg. Stud., 12, 253–272, https://doi.org/10.1016/j.ejrh.2017.06.003, 2017.
Karamuz, E., Bogdanowicz, E., Senbeta, T. B., Napiórkowski, J. J., and Romanowicz, R. J.: Is It a Drought or Only a Fluctuation in Precipitation Patterns? – Drought Reconnaissance in Poland, Water (Basel), 13, 807, https://doi.org/10.3390/w13060807, 2021.
Kubiak-Wójcicka, K. and Bąk, B.: Monitoring of meteorological and hydrological droughts in the Vistula basin (Poland), Environ. Monit. Assess., 190, 87–100, https://doi.org/10.1007/s10661-018-7058-8, 2018.
Liu, B., Zhou, X., Li, W., Lu, C., and Shu, L.: Spatiotemporal Characteristics of Groundwater Drought in Jiangsu Province, China, Water (Basel), 8, 480, https://doi.org/10.3390/w8110480, 2016.
Liu, Y., Ren, L., Zhu, Y., Yang, X., Yuan, F., Jiang, S., and Ma, M.: Evolution of Hydrological Drought in Human Disturbed Areas: A Case Study in the Laohahe Catchment, Northern China, Adv. Meteorol., 2016, 5102568, https://doi.org/10.1155/2016/5102568, 2016.
Marcinkowski, P. and Grygoruk, M.: Long-term downstream effects of a dam on a lowland river flow regime: Case study of the upper narew, Water (Switzerland), 9, 783, https://doi.org/10.3390/w9100783, 2017.
Mckee, T. B., Doesken, N. J., and Kleist, J.: The relationship of drought frequency and duration to time scales, in: Proceedings of the Eighth Conference on Applied Climatology, 17–22 January 1992, Anaheim, California, American Meteorological Society, 179–184, https://www.droughtmanagement.info/literature/AMS_Relationship_Drought_Frequency_Duration_Time_Scales_1993.pdf (last access: 4 April 2023), 1993.
Montanari, A., Young, G., Savenije, H. H. G., Hughes, D., Wagener, T., Ren, L. L., Koutsoyiannis, D., Cudennec, C., Toth, E., Grimaldi, S., Blöschl, G., Sivapalan, M., Beven, K., Gupta, H., Hipsey, M., Schaefli, B., Arheimer, B., Boegh, E., Schymanski, S. J., Di Baldassarre, G., Yu, B., Hubert, P., Huang, Y., Schumann, A., Post, D. A., Srinivasan, V., Harman, C., Thompson, S., Rogger, M., Viglione, A., McMillan, H., Characklis, G., Pang, Z., and Belyaev, V.: “Panta Rhei-Everything Flows”: Change in hydrology and society-The IAHS Scientific Decade 2013–2022, Hydrolog. Sci. J., 58, 1256–1275, https://doi.org/10.1080/02626667.2013.809088, 2013.
Nalbantis, I. and Tsakiris, G.: Assessment of hydrological drought revisited, Water Resour. Manag., 23, 881–897, https://doi.org/10.1007/s11269-008-9305-1, 2009.
Nasiri, N., Asghari, K., and Besalatpour, A. A.: Quantitative analysis of the human intervention impacts on hydrological drought in the Zayande-Rud River Basin, Iran, J. Water Clim. Change, 13, 3473–3495, https://doi.org/10.2166/wcc.2022.188, 2022.
Piniewski, M., Szcześniak, M., Kardel, I., Berezowski, T., Okruszko, T., Srinivasan, R., Vikhamar Schuler, D., and Kundzewicz, Z. W.: Hydrological modelling of the Vistula and Odra river basins using SWAT, Hydrolog. Sci. J., 62, 1266–1289, https://doi.org/10.1080/02626667.2017.1321842, 2017.
Qiu, L., Peng, D., Xu, Z., and Liu, W.: Identification of the impacts of climate changes and human activities on runoff in the upper and middle reaches of the Heihe River basin, China, J. Water Clim. Change, 7, 251–262, https://doi.org/10.2166/wcc.2015.115, 2016.
Raczyński, K.: Influence of a Multipurpose Retention Reservoir on Extreme River Flows, a Case Study of the Nielisz Reservoir on the Wieprz River (Eastern Poland), Water Resour., 47, 29–40, 2020.
Rangecroft, S., Van Loon, A. F., Maureira, H., Verbist, K., and Hannah, D. M.: An observation-based method to quantify the human influence on hydrological drought: upstream–downstream comparison, Hydrolog. Sci. J., 64, 276–287, https://doi.org/10.1080/02626667.2019.1581365, 2019.
Seibert, J. and Vis, M. J. P.: Teaching hydrological modeling with a user-friendly catchment-runoff-model software package, Hydrol. Earth Syst. Sci., 16, 3315–3325, https://doi.org/10.5194/hess-16-3315-2012, 2012.
Senbeta, T. B. and Romanowicz, R. J.: The role of climate change and human interventions in affecting watershed runoff responses, Hydrol. Process., 35, e14448, https://doi.org/10.1002/hyp.14448, 2021.
TAMU (Texas A&M University): ArcSWAT: ArcGIS interface for SWAT 2012, TAMU [code], https://swat.tamu.edu/software/arcswat/, last access: 23 September 2021.
UZH (University of Zurich): HBV-light, UZH [code], https://www.geo.uzh.ch/en/units/h2k/Services/HBV-Model/HBV-Download.html, last access: 24 October 2021.
van Loon, A. F. and van Lanen, H. A. J.: Making the distinction between water scarcity and drought using an observation-modeling framework, Water Resour. Res., 49, 1483–1502, https://doi.org/10.1002/wrcr.20147, 2013.
Van Loon, A. F., Stahl, K., Di Baldassarre, G., Clark, J., Rangecroft, S., Wanders, N., Gleeson, T., Van Dijk, A. I. J. M., Tallaksen, L. M., Hannaford, J., Uijlenhoet, R., Teuling, A. J., Hannah, D. M., Sheffield, J., Svoboda, M., Verbeiren, B., Wagener, T., and Van Lanen, H. A. J.: Drought in a human-modified world: reframing drought definitions, understanding, and analysis approaches, Hydrol. Earth Syst. Sci., 20, 3631–3650, https://doi.org/10.5194/hess-20-3631-2016, 2016a.
van Loon, A. F., Gleeson, T., Clark, J., van Dijk, A. I. J. M., Stahl, K., Hannaford, J., Di Baldassarre, G., Teuling, A. J., Tallaksen, L. M., Uijlenhoet, R., Hannah, D. M., Sheffield, J., Svoboda, M., Verbeiren, B., Wagener, T., Rangecroft, S., Wanders, N., and Van Lanen, H. A. J.: Drought in the Anthropocene, Nat. Geosci., 9, 89–91, https://doi.org/10.1038/ngeo2646, 2016b.
Wanders, N. and Wada, Y.: Human and climate impacts on the 21st century hydrological drought, J. Hydrol. (Amst), 526, 208–220, https://doi.org/10.1016/j.jhydrol.2014.10.047, 2015.
Wittenberg, H.: Baseflow recession and recharge as nonlinear storage processes, Hydrol. Process., 13, 715–726, https://doi.org/10.1002/(SICI)1099-1085(19990415)13:5<715::AID-HYP775>3.0.CO;2-N, 1999.
Wu, J., Chen, X., Yu, Z., Yao, H., Li, W., and Zhang, D.: Assessing the impact of human regulations on hydrological drought development and recovery based on a “simulated-observed” comparison of the SWAT model, J. Hydrol. (Amst), 577, 123990, https://doi.org/10.1016/j.jhydrol.2019.123990, 2019.
Zou, L., Xia, J., and She, D.: Analysis of Impacts of Climate Change and Human Activities on Hydrological Drought: a Case Study in the Wei River Basin, China, Water Resour. Manag., 32, 1421–1438, https://doi.org/10.1007/s11269-017-1877-1, 2017.