the Creative Commons Attribution 4.0 License.
the Creative Commons Attribution 4.0 License.
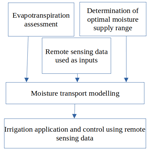
Conceptual principles of water resources management in irrigated agriculture
Mykhailo Romashchenko
Vsevolod Bohaienko
Tetiana Matiash
Andrii Shatkovskyi
Serhii Kolomiets
Iuliia Danylenko
Climate change causes a progressive deterioration of natural water supply increasing the need for irrigation and more rational use of water resources in it as the main mean for ensuring sustainable agriculture. Here we present the conceptual provisions intended to provide a capability to achieve maximum irrigation efficiency, which generalize our experience in irrigation water management in Ukraine. Main principles that should be integrated in an efficient decision support system include the use of a narrow high range of maintained moisture content in the root zone of soil; pressure as a criterion of moisture content level; differential, particularly fractional-order, models of moisture transfer for the prediction of irrigation schedules and rates; several methods of evapotranspiration assessment to improve the accuracy; remote sensing data to disseminate predictions to areas not covered by instrumental monitoring tools. The application of these principles support an increase in crop yields accompanied by irrigation water volumes reduction eliminating water losses for infiltration and the development of flooding and secondary salinization processes.
- Article
(1267 KB) - Full-text XML
- BibTeX
- EndNote
Soil moisture; evapotranspiration; irrigation; decision support; water management
Climate change, which complicates the conditions for agricultural production in most regions, is particularly significant for Ukraine where it is characterized by the fastest (over 0.6 °C in 10 years) increase of average annual air temperature in Europe (Krakovska et al., 2018).
The cultivation of crops in the Steppe and a large part of the Forest-steppe zone of Ukraine, which is of the total cropped area, is carried out in the conditions of the significant deficit (from 150 to > 450–500 mm, Fig. 1) of climatic water balance (Romashchenko et al., 2020b) making urgent the expansion of irrigation.
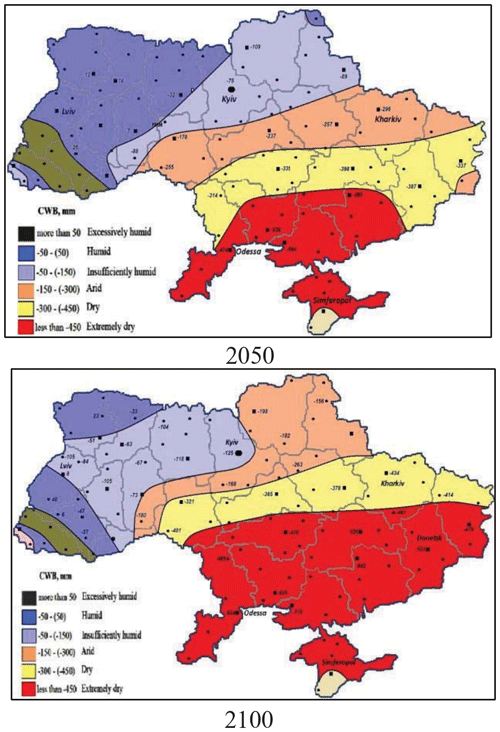
Figure 1Forecast of moisture supply conditions in Ukraine according to annual climatic water balance. * source: Romashchenko et al. (2020b).
The solution of this issue is provided by the “Strategy of Irrigation and Drainage in Ukraine for the period up to 2030” approved by the Cabinet of Ministers of Ukraine and the “Action Plan” for its implementation. Fulfilling their tasks in the aspect of increasing irrigation areas by 1.0–1.2 million ha will require the involvement of significant (> 4.0–4.5 billion m3 considering the data given in Romashchenko et al., 2020b) additional volumes of water. Given the negative impact of climate change on the state of usable water resources, efficient water use is an important condition for increasing irrigation capacity.
The main components of solving the problem of efficient water use are the application of water-saving irrigation methods (varieties of drip irrigation and low-pressure sprinklers) and decision support systems (DSS) in irrigation management (Mainaa et al., 2014).
DSSs in irrigation are tools used in the management of irrigation processes aimed at regulating water supply to plants according to their needs, which contributes to the increase of water productivity. They are usually based on biomass accumulation and evapotranspiration models. The most common approach is grounded on the relationship between seasonal transpiration of crops under proper water supply and their biomass (de Wit, 1958).
Necessary water supply regimes are provided using a variety of methods including an approach based on plant state assessment (Jones, 2004) and an approach based on the control of moisture supply to the root layer of soil (Campbell et al., 1982).
Methodological background of the decisions on the formation of irrigation regimes determines the level of efficiency and environmental friendliness of irrigation water usage and the ways to achieve it when managing irrigation using DSSs.
Thus, the aim of this study is to determine conceptual foundations of irrigation management using DSSs aimed at increasing the efficiency and environmental safety of irrigation. The use of these foundations should allow the fullest use of the potential (the maximum yield under close to optimal agricultural practices) of varieties and hybrids of crops with minimal irrigation water use per unit of yield. This should be accompanied by the minimization of infiltration water losses and, therefore, the risks of flooding and secondary salinization of irrigated lands. The scheme of core components of the considered methodology is depicted in Fig. 2.
Irrigation should ensure the maintenance of moisture supply to the root layer of soil throughout the growing season in the optimal range for the growth and development of crops. Optimal one is such a level of moisture supply at which water consumption of irrigated crops is equal to or close to potentially possible under current conditions (Campbell et al., 1982).
According to the results of most studies, the upper limit of the optimal moisture supply range (OMSR) should be taken at the level of field capacity (FC), and the lower limit (pre-irrigation threshold) is a dynamic indicator that varies depending on the type of crop and its development stage (see, e.g., Wang et al., 2011). OMSR is an indicator that directly influences irrigation rate, hence the approach to its determination needs to be clarified.
The most common methods for determining FC by plots flooding in field conditions overestimate its value (Romashchenko et al., 2019) provoking a higher level of soil moisture during irrigation, causing the formation of easily mobile moisture, and creating preconditions for irrigation water losses on infiltrating below the root layer.
Therefore, in Romashchenko et al. (2019) a method for laboratory determination of FC on soil monoliths was proposed. The values of FC obtained using this technique are usually a few percent less than the values determined by plots flooding. So, for practical purposes, we propose to use as the upper limit of OMSR the values of FC determined by this method.
Regarding the lower limit of OMSR many experimental plant-related studies conducted for the specific crop and growing conditions propose the use of soil moisture values in the range from 60 % to 90 % FC (Muromtsev, 2011; Shatkovskyi et al., 2022). We propose to adhere a different approach (see, e.g., Shahnazari et al., 2007) that consists of maintaining moisture supply in the root layer in a narrow high range (usually above 80 % FC). According to its rationing this ensures the actual water consumption of crops to be equal to or close to potentially possible in current weather conditions.
The most accurate approaches to determining the lower limit of OMSR are related to the use of the water retention curves (WRC) and suction pressure as an indicator of moisture supply (Campbell et al., 1982). The use of WRC makes it possible to consider moisture content in combination with pore structure and to determine FC in terms of pressure. Further, the limits of OMSR are determined also in terms of pressure through a given range of per cents of FC. As an additional constraint on pre-irrigation threshold we propose it to be determined considering the reservation of soil moisture in the root layer of soil for at least one day of water consumption at the highest potentially possible level. This constraint is aimed at protecting plants from water stress in the conditions of rapid change of weather or potential failures in the application of watering.
Such an approach leads to the reduction of irrigation rates by 1.5–2.0 times making possible the implementation of environmentally friendly irrigation. It also contributes to obtaining yields close to the maximum possible at other unchanged elements of agricultural technology thus reducing the cost of irrigation water to form a unit of yield.
The presented conceptual principles were formed on the basis of the generalization of the results of long-term research of the authors performed in Ukraine and research data of other authors.
An important component of modern DSSs in irrigation management is the forecasting of soil moisture dynamics. Currently, the most used for these purposes is the Richards differential equation (Richards, 1931) that describes moisture transfer in soils with properties independent of scale. To increase modelling accuracy in porous media of fractal structure, it is proposed to use fractional-differential equations (Kavvas et al., 2017). Such models can, in particular, be considered as semi-empirical with the increased number of degrees of freedom while identifying their parameters in specific complex hydrogeological conditions (Romashchenko et al., 2021).
We also recommend to use equations that describe water transfer processes in terms of soil moisture potential and thus consider the vadose zone within the same hydrodynamic scheme with groundwater horizons.
Data from direct measurements of moisture level should be used to determine initial conditions, assess the quality of forecast, and calibrate the models. It is logical to use also the indicator of moisture potential and measure it using suction pressure sensors. The number of measurement points within a farm is determined according to the need to have at least one observation point for each “soil-crop” pair and perform measurements at two or more depths.
The use of moisture potential as an indicator of moisture supply level is substantiated by the fact that it is a direct indicator that characterizes the force with which soil moisture is retained in pores and does not depend on soil type. At moisture content level close to pre-irrigation threshold, its small changes may be accompanied by significant changes in pressure, which leads to higher accuracy in determining the state of moisture supply by pressure sensors (Degré et al., 2017).
The accuracy of evapotranspiration estimates is one of the determining factors in modelling the state of moisture supply in the process of irrigation management. On the one hand, quantifying evapotranspiration intensity and more accurate modelling of moisture transfer requires integrated models that take into account soil and atmospheric physics along with plant physiology (Overgaard et al., 2006). Such models include the Penman-Monteith, Shuttleworth-Wallace, and Priestley-Taylor models and have numerous parameters that are often hard to assess. On the other hand, there is a large amount of easy to calculate empirical methods, the effectiveness of which is limited by specific agroclimatic conditions. The most used in Ukraine are, in particular, the methods of Dmytro Shtoyko and Mykola Ivanov (Romashchenko et al., 2020a).
The problem of determining the accuracy of evapotranspiration estimates remains relevant for each specific situation because under irrigation the maintained moisture ranges in the root layer of soil significantly influence the availability of water to plants, changing main factors influencing evapotranspiration. Thus, a specific empirical model or the parametrization of a phenomenological model that gives the best accuracy of pressure dynamics modelling should be determined in each particular case.
As currently no universal method exists for assessing evapotranspiration, in DSSs in irrigation we recommend (Romashchenko et al., 2020a) simultaneous usage of the models with the maximum number of parameters. It could be the Penman-Monteith model with mandatory determination of crop coefficients for each specific case (for Ukraine see Shatkovskyi et al., 2020) and empirical models developed for specific agroclimatic conditions. Such a combination makes it possible to more accurately determine evapotranspiration in the process of changes of crop development stages.
A critical input for the forecast calculations of evapotranspiration is weather forecast data. Our experience shows that with sufficient accuracy for the practice of irrigation management one can use the average weather forecast data from at least three of the most common weather information websites (Kovalchuk et al., 2018) and perform forecasting of irrigation schedules for up to 5 d.
Another important aspect of modern methods for irrigation management is the intensive use of remote sensing data. The combination of hydrological models and remote sensing overcomes many of the shortcomings associated with low spatial resolution of these models and low temporal resolution of remote sensing data (Droogers and Bastiaanssen, 2002). This combination facilitates detailed spatial and temporal analysis while assessing irrigation efficiency, and can be used to identify areas where improved irrigation management is needed (Santos et al., 2008).
The ability of remote sensing to provide assessment of soil moisture content (Babaeian et al., 2019; Danylenko and Bohaienko, 2020) is highly important. When some fields have in-field monitoring tools and the other ones not, the usage of remote sensing allows irrigation management in fields not covered by monitoring tools using monitoring data from other fields to calibrate relevant models.
Progressive deterioration of natural moisture supply due to global warming requires the expansion of the use of irrigation as the main technological method for ensuring the sustainability of agriculture in the conditions of climate change.
In these conditions, the highest efficiency and environmental safety of irrigation is achieved when using for irrigation management the decision support systems that implement the above-described conceptual principles.
Based on the results of research carried out by the authors, the introduction of these principles in the practice of irrigation management in Ukraine ensures yields of irrigated crops at the level of 85 %–90 % of the potential of their varieties and hybrids (the maximum yield under close to optimal agricultural practices indicated in the passport of the variety (hybrid) in the State register of plant varieties suitable for distribution in Ukraine). It also reduces irrigation costs per unit of yield and allows conducting irrigation according to environmentally friendly standards minimizing or eliminating irrigation water losses on infiltration along with the development of flooding and secondary salinization processes.
Supporting software codes are available from the authors upon reasonable request.
The data are available from the authors upon reasonable request.
MR performed conceptualization; MR, VB, and SK designed methodology; VB, TM, AS, SK, and ID conducted an investigation; MR and VB wrote the manuscript; TM and ID reviewed and edited the manuscript.
The contact author has declared that none of the authors has any competing interests.
Publisher's note: Copernicus Publications remains neutral with regard to jurisdictional claims in published maps and institutional affiliations.
This article is part of the special issue “IAHS2022 – Hydrological sciences in the Anthropocene: Past and future of open, inclusive, innovative, and society-interfacing approaches”. It is a result of the XIth Scientific Assembly of the International Association of Hydrological Sciences (IAHS 2022), Montpellier, France, 29 May–3 June 2022.
This paper was edited by Christophe Cudennec and reviewed by two anonymous referees.
Babaeian, E., Sadeghi, M., Jones, S. B., Montzka, C., Vereecken, H., and Tuller, M.: Ground, proximal, and satellite remote sensing of soil moisture, Rev. Geophys., 57, 530–616, https://doi.org/10.1029/2018RG000618, 2019.
Campbell, G. S., Campbell, M. D., and Hillel, D.: Irrigation scheduling using soil moisture measurements: theory and practice, Advances in Irrigation, 1, 25–42, https://doi.org/10.1016/B978-0-12-024301-3.50008-3, 1982.
Danylenko, I. and Bohaienko, V.: Monitoring of soil moisture in the south of Ukraine using active and passive remote sensing data, Remote Sensing for Agriculture, Ecosystems, and Hydrology XXII, 11528, 1152807, https://doi.org/10.1117/12.2571049, 2020.
Degré, A., van der Ploeg, M. J., Caldwell, T., and Gooren, H. P.: Comparison of soil water potential sensors: a drying experiment, Vadose Zone J., 16, 1–8 https://doi.org/10.2136/vzj2016.08.0067, 2017.
de Wit, C. T.: Transpiration and crop yields, Versl. Landbouwk, Onderz. 64.6, Institute of Biological Chemistry Research on Field Crops and Herbage, Wageningen, The Netherlands, https://edepot.wur.nl/186445 (last access: 6 March 2023), 1958.
Droogers, P. and Bastiaanssen, W.: Irrigation performance using hydrological and remote sensing modeling, J. Irrig. Drain. E.-ASCE, 128, 11–18, https://doi.org/10.1061/(ASCE)0733-9437(2002)128:1(11), 2002.
Jones, H. G.: Irrigation scheduling: advantages and pitfalls of plant-based methods, J. Exp. Bot., 55, 2427–2436, https://doi.org/10.1093/jxb/erh213, 2004.
Kavvas, M. L., Ercan, A., and Polsinelli, J.: Governing equations of transient soil water flow and soil water flux in multi-dimensional fractional anisotropic media and fractional time, Hydrol. Earth Syst. Sci., 21, 1547–1557, https://doi.org/10.5194/hess-21-1547-2017, 2017.
Kovalchuk, V., Demchuk, O., Demchuk, D., and Voitovich, O.: Data mining for a model of irrigation control using weather web-services, in: International Conference on Computer Science, Engineering and Education, edited by: Hu, Z., Petoukhov, S., Dychka, I., and He, M., Advances in Intelligent Systems and Computing, Vol. 754, Springer, Cham, 133–143, https://doi.org/10.1007/978-3-319-91008-6_14, 2018.
Krakovska, S. V., Palamarchuk, L. V., Gnatiuk, N. V., and Shpytal, Ò. M.: Projections of air temperature and relative humidity in Ukraine regions to the middle of the 21st century based on regional climate model ensembles. Geoinformatika, 3, 62–77, http://www.geology.com.ua/en/7514-2/ (last access: 6 March 2023), 2018.
Mainaa, M. M., Amina, M. S. M., and Yazidb, M. A.: Web geographic information system decision support system for irrigation water management: a review, Acta Agr. Scand. B–S. P., 64, 283–293, https://doi.org/10.1080/09064710.2014.896935, 2014.
Muromtsev, N.: Plant moisture availability assessment, Dokuchaev Soil Bulletin, 67, 20–31, https://doi.org/10.19047/0136-1694-2011-67-20-31, 2011 (in Russian).
Overgaard, J., Rosbjerg, D., and Butts, M. B.: Land-surface modelling in hydrological perspective – a review, Biogeosciences, 3, 229–241, https://doi.org/10.5194/bg-3-229-2006, 2006.
Richards, L. A.: Capillary conduction of liquids through porous mediums, Physics, 1, 318–333, https://doi.org/10.1063/1.1745010, 1931.
Romashchenko, M. I., Kolomiets, S. S., and Bilobrova, A. S.: Laboratory diagnostic system for water-physical soil properties, Melioratisia i vodne hospodarstvo, 2, 199–208, https://doi.org/10.31073/mivg201902-193, 2019 (in Ukrainian).
Romashchenko, M. I., Bohaienko, V. O., Matiash, T. V., Kovalchuk, V. P., and Danylenko, I. I.: Influence of evapotranspiration assessment on the accuracy of moisture transport modeling under the conditions of sprinkling irrigation in the south of Ukraine, Arch. Agron. Soil Sci., 66, 1424–1435, https://doi.org/10.1080/03650340.2019.1674445, 2020a.
Romashchenko, M. I., Husiev, I. V., Shatkovskyi, A. P., Saidak, R. V., Iatsiuk, M. V., Shevchenko, A. M., and Matiash, T. V.: Impact of climate change on water resources and agricultural production, Melioratsiia i vodne hospodarstvo, 1, 5–22, https://doi.org/10.31073/mivg202001-235, 2020b (in Ukrainian).
Romashchenko, M. I., Bohaienko, V. O., Matiash, T. V., Kovalchuk, V. P., and Krucheniuk, A. V.: Numerical simulation of irrigation scheduling using fractional Richards equation, Irrigation Sci., 39, 385–396, https://doi.org/10.1007/s00271-021-00725-3, 2021.
Santos, C., Lorite, I. J., Tasumi, M., Allen, R. G., and Fereres, E.: Integrating satellite-based evapotranspiration with simulation models for irrigation management at the scheme level, Irrigation Sci., 26, 277–288, https://doi.org/10.1007/s00271-007-0093-9, 2008.
Shahnazari, A., Liu, F., Andersen, M. N., Jacobsen, S. E., and Jensen, C. R.: Effects of partial root-zone drying on yield, tuber size and water use efficiency in potato under field conditions, Field Crop. Res., 100, 117–124, https://doi.org/10.1016/j.fcr.2006.05.010, 2007.
Shatkovskyi, A., Romashchenko, M., Zhuravlov, O., Riabkov, S., Cherevychnyi, Y., and Hulenko, O.: Optimization of the parameters of drip irrigation regimes for crops in the steppe of Ukraine, Land Reclamation and Water Management, 2, 45–50, https://doi.org/10.31073/mivg202202-338, 2022.
Shatkovskyi, A. P., Romashchenko, M. I., Zhuravlov, O. V., Vasyuta, V. V., Melnychuk, F. S., Ovchatov, I. M., Yarosh, A. V., and Semenko, L. O.: Evaluation of the “Penman-Monteith” model for determination of soybeans' evapotranspiration in irrigated conditions of the Steppe of Ukraine, Modern Phytomorphology, 14, 111–113, https://doi.org/10.5281/zenodo.5078016, 2020.
Wang, F. X., Wu, X. X., Shock, C. C., Chu, L. Y., Gu, X. X., and Xue, X.: Effects of drip irrigation regimes on potato tuber yield and quality under plastic mulch in arid Northwestern China, Field Crop. Res., 122, 78–84, https://doi.org/10.1016/j.fcr.2011.02.009, 2011.
- Abstract
- Keywords
- Introduction
- Principles of irrigation appointment
- Principles of forecasting soil moisture dynamics
- Principles of evapotranspiration assessment
- Principles of remote sensing data usage
- Conclusions
- Code availability
- Data availability
- Author contributions
- Competing interests
- Disclaimer
- Special issue statement
- Review statement
- References
- Abstract
- Keywords
- Introduction
- Principles of irrigation appointment
- Principles of forecasting soil moisture dynamics
- Principles of evapotranspiration assessment
- Principles of remote sensing data usage
- Conclusions
- Code availability
- Data availability
- Author contributions
- Competing interests
- Disclaimer
- Special issue statement
- Review statement
- References