the Creative Commons Attribution 4.0 License.
the Creative Commons Attribution 4.0 License.
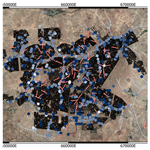
Rainfall measurement from mobile telecommunication network and potential benefit for urban hydrology in Africa: a simulation framework for uncertainty propagation analysis
Maxime Turko
Marielle Gosset
Christophe Bouvier
Nanee Chahinian
Matias Alcoba
Modeste Kacou
Apoline Yappi
Urban floods due to intense precipitation is a major problem in many developing countries, especially in Africa. There are few available solutions for effective and yet affordable flood early warning systems for these regions. Weather radar is common in industrialized countries but too costly for most tropical cities. Satellite provides interesting information in real time but not yet quantitative enough at the space and time scales needed for urban flood monitoring. Rainfall measurement using commercial microwave links (CML) from cellular communication networks is a cost effective alternative to conventional methods. The method is based on measuring rain induced fluctuations between telecommunication antennas; if the operator provides this information rain maps can be produced and used for hydrological prediction. Many CML studies have been carried out in Europe and Israel. Recently IRD implemented pilot sites in Africa in order to test this rainfall estimation technique and quantify the uncertainties. After reviewing the method principles and providing an overview of the current research on CML, we present a simulation framework to analyse the propagation of CML rainfall uncertainties in an urban hydrological model.
- Article
(1005 KB) - Full-text XML
- BibTeX
- EndNote
Urban floods in West Africa engender every year much human and material damage. To monitor these risks, flood alert systems based on reliable rainfall estimation are needed. In industrialized countries, alert systems are usually based on weather radar networks that are not yet available in developing countries. Satellite provides interesting information in real time but not yet quantitative enough at the space and time scales needed for urban flood monitoring. In the last decade, studies have demonstrated the possibility to use mobile telecommunication networks to estimate rainfall.
The method exploits the fluctuation of the microwave signal due to attenuation by rainfall. This method is cost effective because an existing network is used rather then costly investment in new instruments. The high density of the mobile network in cities is advantageous for urban applications. The IRD (Institut de Recherche pour le Développement) initiated the Raincell (for Rain measurement from Cellular phone network) project to promote and demonstrate the use of commercial microwave links (CML) for rainfall measurement in West Africa (Gosset et al., 2016; film “Raincell Africa: Alert signal” available at: https://www.youtube.com/watch?v=QM9GHchJIf4&feature=youtu.be, last access: 12 August 2020). Pilot experiments have been set up in several African cities: Ouagadougou (Burkina-Faso; Doumounia et al., 2014), Bamako (Mali), Niamey (Niger) and Yaounde and Douala in Cameroun.
1.1 Rainfall measurement with commercial microwave links (CML)
Rainfall measurement by CML is based on the robust relationship between microwave signal attenuation and rainfall intensity over the link (between two telecommunication antennas). This principle is summarized in Eq. (1):
the Path Integrated Attenuation (PIA in dB) measured by the link of length L, can be related to the Rainfall intensity over the link (R), through a simple power law relation – the coefficients a and b depend on the operation frequency and can be estimated easily. a increases with frequency. b is close to 1 (0.5 to 2) for common telecom frequencies and the relation is quasi-linear near 30 GHz. If the PIA is provided by the mobile operator, Eq. (1) can be inverted to estimate rainfall all over the network.
The accuracy in rainfall measurement using the CML network (from Eq. 1) depends on many factors: the variability of the coefficients a and b in Eq. (1); the density and length of the links; the accuracy of the PIA itself. The latter depends on the method used by the operator to collect and record the raw power levels (typically accuracy of 1 dB or 0.1 dB is common).
1.2 Current research
Research on rainfall measurement from CML started in 2003 in the Netherlands and Israel, later followed by several international research groups. The first papers focused on the method feasibility at link scale (Messer et al., 2006) and on the uncertainties (Leijnse et al., 2007; Berne and Uijlenhoet, 2007). These research groups have shown the possibility to use this method in various environment and region in the world, and at different spatial scales, from a single link to an agglomeration or a country. The state of the art about the CML technique and its applications is well described in Uijlenhoet et al. (2018).
In the next section we introduce a simulation framework develop to analyse the potential of this technique for urban hydrology, accounting for rainfall uncertainty propagation.
Several studies have analysed and quantified the uncertainties in CMLs' rainfall estimation, for different network configuration and rainfall regime (Berne and Uijlenhoet, 2007; Leijnse et al., 2007, 2008a, b, 2010; Zinevich et al., 2010; Schleiss et al., 2013; van Leth et al., 2017). Here a simulation framework has been set up in order to analyse how typical errors in CMLs rainfall estimation may propagate within a hydrological model. Building on previous studies, the considered uncertainties relate to: the accuracy of attenuation and rainfall estimation for each link ; the network configuration (density; length; frequency).
The city of Ouagadougou where the first Raincell pilot started (Doumounia et al., 2014) is taken as an example. A simulator of a network of links was created based on radar rainfall fields used as a reality proxy. The radar rain fields were obtained from a campaign that took place in Ouagadougou in 2012 and 2013 (http://meghatropiques.ipsl.polytechnique.fr/the-ouagadougou-super-site/, last access: 12 August 2020).
2.1 A link simulator to generate pseudo estimation and their uncertainties
Each link is emulated as the weighted mean of the radar map pixels crossed by the link:
where Rlink is the mean rainfall intensity over the link of length Llink. Rpixi is the rainfall intensity of a pixel i weighted by the link size li in this pixel. Using Equation 1 the PIA over the link is then computed. To take into account measurement accuracy the PIA is rounded at in 1 dB or at 0.1 dB, which are typical values for the data provided by the operators. Several network configurations can be simulated by varying the a, b and L parameters in Eq (1).
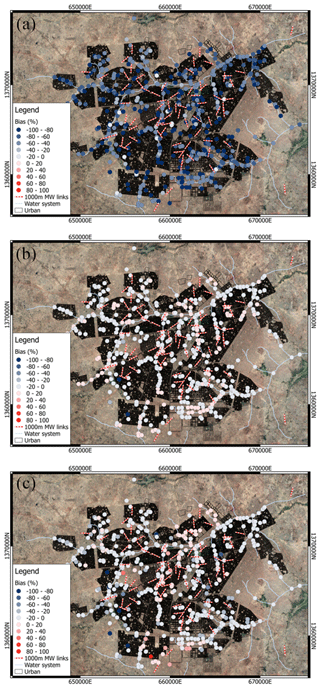
Figure 1Map of the bias (%) between the simulated discharge when the CML network provides perfect accuracy on the attenuation (reference) and the simulated discharge with less accurate measurement of CML attenuation (1 dB or 0.1 dB). (a) CML configuration with 6 GHz frequency and 0.1 dB precision. (b) CML configuration with 30 GHz and 0.1 dB. (c) CML configuration with 30 GHz and 1 dB. Map background information provided by © Google Maps and © OpenStreetMap contributors 2016. Distributed under a Creative Commons BY-SA License.
2.2 Analysis of the uncertainties in the simulated discharge
An urban distributed hydrological model (Athys, Bouvier et al., 2018) is used to analyse the propagation of the CML rainfall uncertainties in the simulated discharge. The discharge uncertainty analysis is performed at several discharge points within the watershed in order to study its variability. The sensitivity of the discharge to various sources of uncertainty in the rain field is analysed with numerical experiments: using the original rain map as a forcing field, or using the simulated CMLs derived rain maps, with different network configurations and PIA accuracy. As an example, Fig. 1 compares the bias (in %) in the discharge for a CMLs network with coarse accuracy (1 dB) with the same network but with full accuracy (no error on the attenuation PIA). Two frequencies (6 and 30 GHz) and two signal accuracy hypothesis (0.1 and 1 dB) are displayed. In this example all links are 1000 m long. The 6 GHz and 1 dB configuration is not represented because the bias was homogenous (−100 %). The figure highlights the importance of the raw signal accuracy, especially when the network frequency is low. At, 6 GHz frequency, 1 dB accuracy on a 1 km link rainfall below 100 mm h−1 would not be detected; with 0.1 dB accuracy th threshold would be 28.4 mm h−1. At higher frequency, the attenuation for a given rain rate is higher; at 30 GHz frequency, 0.1 dB over 1 km is equivalent to 0.4 mm h−1 and 1 dB to 4.4 mm h−1; in both cases the amount of rainfall missed by the network is low, and the bias in the discharge is therefore small (Fig. 1b and c) whatever the accuracy.
The presented CML simulation framework coupled with a hydrological model can be used to test various sensitivities (network configuration and density within the basin). It is a useful tool to demonstrate to operators the potential use of the information collected on their network and the importance of raw data accuracy.
Data available from the corresponding author upon request.
MT run the simulations and provided all plots and analysis; MG supervised the study and wrote the English text; CB and NC provided and configured the hydrological model; MK, MA and AY participated in the technical discussions on the CML processing.
The authors declare that they have no conflict of interest.
This article is part of the special issue “Hydrological processes and water security in a changing world”. It is a result of the 8th Global FRIEND–Water Conference: Hydrological Processes and Water Security in a Changing World, Beijing, China, 6–9 November 2018.
Berne, A. and Uijlenhoet, R.: Path-averaged rainfall estimation using microwave links: Uncertainty due to spatial rainfall variability, Geophys. Res. Lett., 34, 1–5, https://doi.org/10.1029/2007GL029409, 2007.
Bouvier, C., Chahinian, N., Adamovic, M., Cassé, C., Crespy, A., Crès, A., and Alcoba, M.: Large-Scale GIS-Based Urban Flood Modelling: A Case Study on the City of Ouagadougou, Adv. Hydroinformatics, 703–717, https://doi.org/10.1007/978-981-10-7218-5_50, 2018.
Doumounia, A., Gosset, M., Cazenave, F., Kacou, M., and Zougmore, F.: Rainfall monitoring based on microwave links from cellular telecommunication networks: First results from a West African test bed: wireless networks for rainfall in Africa, Geophys. Res. Lett., 41, 6016–6022, https://doi.org/10.1002/2014GL060724, 2014.
Gosset, M., Kunstmann, H., Zougmore, F., Cazenave, F., Leijnse, H., Uijlenhoet, R., Chwala, C., Keis, F., Doumounia, A., Boubacar, B., Kacou, M., Alpert, P., Messer, H., Rieckermann, J., and Hoedjes, J.: Improving Rainfall Measurement in Gauge Poor Regions Thanks to Mobile Telecommunication Networks, B. Am. Meteorol. Soc., 97, ES49–ES51, https://doi.org/10.1175/BAMS-D-15-00164.1, 2016.
Leijnse, H., Uijlenhoet, R., and Stricker, J. N. M.: Hydrometeorological application of a microwave link: 2. Precipitation: Microwave Link, 2, Water Resour. Res., 43, 1–9, https://doi.org/10.1029/2006WR004989, 2007.
Leijnse, H., Uijlenhoet, R., and Stricker, J. N. M.: Microwave link rainfall estimation: Effects of link length and frequency, temporal sampling, power resolution, and wet antenna attenuation, Adv. Water Resour., 31, 1481–1493, https://doi.org/10.1016/j.advwatres.2008.03.004, 2008a.
Leijnse, H., Uijlenhoet, R., and Holleman, I.: Sources of error in microwave link rainfall estimation, Proceedings of the International Symposium on Weather Radar and Hydrology, Grenoble, France, 10–15 March 2008, p. 4, 2008b.
Leijnse, H., Uijlenhoet, R., and Berne, A.: Errors and Uncertainties in Microwave Link Rainfall Estimation Explored Using Drop Size Measurements and High-Resolution Radar Data, J. Hydrometeor., 11, 1330–1344, https://doi.org/10.1175/2010JHM1243.1, 2010.
Messer, H., Zinevich, A., and Alpert, P.: Environmental Monitoring by Wireless Communication Networks, Science, 312, 713–713, https://doi.org/10.1126/science.1120034, 2006.
Schleiss, M., Rieckermann, J., and Berne, A.: Quantification and Modeling of Wet-Antenna Attenuation for Commercial Microwave Links, IEEE Geosci. Remote Sens., 10, 1195–1199, https://doi.org/10.1109/LGRS.2012.2236074, 2013.
Uijlenhoet, R., Overeem, A., and Leijnse, H.: Opportunistic remote sensing of rainfall using microwave links from cellular communication networks, WIREs Water 5, 1–15, https://doi.org/10.1002/wat2.1289, 2018.
van Leth, T. C., Overeem, A., Leijnse, H., and Uijlenhoet, R.: A measurement campaign to assess sources of error in microwave link rainfall estimation, Atmos. Meas. Tech., 11, 4645–4669, https://doi.org/10.5194/amt-11-4645-2018, 2018.
Zinevich, A., Messer, H., and Alpert, P.: Prediction of rainfall intensity measurement errors using commercial microwave communication links, Atmos. Meas. Tech., 3, 1385–1402, https://doi.org/10.5194/amt-3-1385-2010, 2010.