the Creative Commons Attribution 4.0 License.
the Creative Commons Attribution 4.0 License.
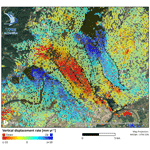
Nationwide deformation monitoring with SqueeSAR® using Sentinel-1 data
Christine A. Bischoff
Alessandro Ferretti
Fabrizio Novali
Andrea Uttini
Chiara Giannico
Francesco Meloni
Subsidence can now be routinely mapped on a national scale thanks to ESA's Sentinel-1 sensors and advanced scalable SqueeSAR® processing. In order to be integrated into existing monitoring programmes, the SqueeSAR® datasets can be calibrated with GNSS measurements. The dense spatial coverage of SqueeSAR® deformation maps captures local deformation phenomena, and with appropriate calibration, can advance the understanding of regional deformation trends. The regular and reliable SAR image acquisitions by Sentinel-1, as well as significant improvements in the scalability of SqueeSAR® processing allow regular updates of deformation maps on a national scale. Filtering the large amount of data for relevant information is achieved by using an algorithm to detect changes in displacement trends.
The Sentinel-1 satellites of the European Space Agency (ESA) are acquiring SAR images since late 2014, and are the first (civilian) sensors specifically designed for surface deformation monitoring over large areas. The Sentinel-1 image stacks have been used to produce the deformation maps presented here, which have been processed using TRE ALTAMIRA's advanced InSAR processing algorithm called SqueeSAR®. Significant improvements in computing power and adapting the SqueeSAR® processing to cloud computing allow regular updates of nationwide InSAR deformation maps. The calibration methodology presented here provides a way to integrate InSAR deformation maps with GNSS measurements. The results show examples from some of the yearly updated national deformation maps currently provided for Denmark, Japan, France and California (US) (e.g. Ferretti et al., 2019). Furthermore, examples from Tuscany are presented, where as part of a continuous monitoring programme, regional deformation maps are updated every two weeks. To retrieve relevant information from this large amount of data, the maps are filtered with a trend change detection algorithm to highlight areas of significant deformation (Del Soldato et al., 2019).
SqueeSAR® is a proprietary multi-interferogram technique (Ferretti, 2014; Ferretti et al., 2011), providing high precision measurements of ground displacement by processing multi-temporal satellite SAR images acquired over the same area, from the same acquisition geometry. By means of a statistical analysis of amplitude and phase data, the SqueeSAR® technique can select a sparse grid of image pixels, which can be used as a “natural geodetic network” to study and monitor slow surface deformation phenomena, with a precision of a few millimetres (Ferretti, 2014).
2.1 2-D Displacement Data
As any other InSAR analysis, the SqueeSAR® technique measures the projection of the displacement vector affecting each radar target along the satellite's line of sight (LOS) (Ferretti et al., 2019). However, a proper combination of SqueeSAR® results obtained from two different acquisition geometries (i.e. ascending and descending), acquired over the same area in the same temporal period, allows one to estimate 2-D measurements, along vertical and east-west direction.
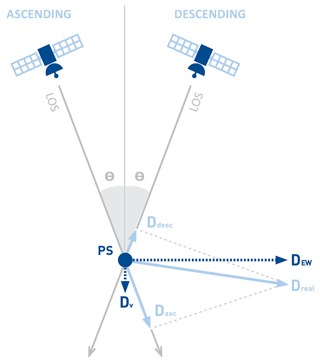
Figure 1Decomposition of LOS displacements into vertical and horizontal components from images acquired in ascending and descending orbits.
This methodology requires the same target to be visible from both ascending and descending orbits: a condition not met very often in real-life scenarios. To overcome this limitation, the constraint of the radar target is relaxed: the area of interest is split into small patches of terrain and the measurements of all measurement points within the same cell are averaged, creating just one “pseudo-PS”. The combination of the results and the estimation of the 2-D displacement time series is then performed for each cell containing data from both acquisition geometries (Ferretti, 2014; Teatini et al., 2011).
It should be noted that, since the acquisition dates usually differ from one dataset to another, the displacement measurements of each “pseudo-PS” are interpolated and re-sampled on a common temporal grid. For Sentinel-1 data, the spatial grid used for the estimation of 2-D displacement data is typically 80×80 m, while the sampling step in time is usually kept equal to 12 d.
2.2 Wide area monitoring with InSAR (SqueeSAR®)
Compared to InSAR analyses over areas of interest of hundreds or a few thousand square kilometres, Wide Area Processing (WAP) is characterized by four main challenges (Ferretti et al., 2019): Atmospheric effects – It is well known that the variance of atmospheric effects increases with the distance from a reference point (Ferretti, 2014; Hanssen, 2001). Moreover, ionospheric effects can become more significant. It is then extremely important, for the generation of high quality InSAR results over wide areas, to reduce the impact of this kind of disturbances, by using prior information (e.g. using GNSS data) or numerical weather models (Yague-Martinez et al., 2016).
Data mosaicking – in WAP, it is mandatory to deal with data mosaicking, to avoid introducing inconsistencies in combining results obtained from a number of independent “processing sites”. As a minimum, for Sentinel-1 data acquired in TOPS mode, the processing algorithm should not introduce any phase variations passing from one burst to another (Yague-Martinez et al., 2016).
Computational burden – It is somewhat obvious that what is feasible when running a multi-interferogram approach on 30 images acquired over an area of interest of 100 km2 can become extremely challenging, if not impossible, when extended to 300 000 km2 covered by thousands of SAR images. Requirements on data storage, processing times, number of CPUs involved, and speed for data transfer can change by orders of magnitude. Although cloud computing can indeed be the solution, it is worth recalling that a proper and efficient use of the cloud requires bespoke software development, at least for complex processing chains, which can have major impact on processing costs.
All three points mentioned above, were carefully considered when developing the new processing chain for wide area processing used to generate the InSAR results presented in this paper.
2.3 SqueeSAR® calibration using GNSS networks
Calibration with other measurements, such as GNSS data, is essential to validate and to tie nationwide InSAR data into existing monitoring programmes. In order to limit the impact of spurious regional trends on the estimated displacement data, it is recommended to rely on several GNSS stations to calibrate the SqueeSAR® datasets. An example of the calibrated SqueeSAR® results for the Denmark national deformation map is shown in Fig. 2, with the GNSS stations marked in red.
The calibration methodology can be applied to both LOS measurements and the derived vertical and east-west components. The following outlines the main steps in the calibration procedure:
Time series filtering: GNSS time series are filtered using a moving average to reduce noise in the measurements. The time series of SqueeSAR® measurement points within a certain radius of each GNSS station (e.g. 200 m) are averaged.
Low frequency phase component: during the initial SqueeSAR® processing, the large-scale low frequency phase patterns are removed, to avoid biases caused by uncompensated atmospheric components. To recover low frequency patterns due to real motion, first the difference in average velocity (linear trend) between each average SqueeSAR® time series and the corresponding filtered GNSS station time series was calculated. These differences were then used to estimate a first order surface (plane), which was subtracted from the SqueeSAR® data. This ensures that SqueeSAR® measurement points now also contain the low frequency component of the motion that was removed during the initial SqueeSAR® processing.
Calibration: this step ties the two measurement techniques together and references the relative SqueeSAR® measurements to the reference of the GNSS network. The procedure involves the generation of a time series of residuals, which is derived from comparing the averaged SqueeSAR® time series to the corresponding GNSS time series for each GNSS station. All the time series of residuals are then averaged to define a common time series of residuals (cRTS). This cRTS represents the movement of the local SqueeSAR® reference points with respect to the GNSS reference frame. The cRTS was then removed from every SqueeSAR® measurement point time series.
The flow chart in Fig. 3 is a summary of these steps.
The following examples are taken from the national SqueeSAR® map for California (US), which was calibrated with GNSS data, from the national SqueeSAR® map for Japan, which was not calibrated with GNSS data and from the SqueeSAR® monitoring service provided to the region of Tuscany (Italy), which is updated every two weeks.
3.1 Aquifer related subsidence in San Joaquin Valley, California (US)
Nationwide ground deformation maps can deliver unique information by constraining the spatial extent of large-scale ground deformation phenomena, such as the subsidence in San Joaquin Valley, California, shown in Fig. 4. It is well established that land subsidence in San Joaquin Valley is a danger to infrastructure, such as bridges, and that it has been caused by a combination of anthropic and natural factors, such as water pumping for agriculture and droughts (Faunt et al., 2016). In fact, water overdraft and the subsequent land subsidence over San Joaquin Valley already started in the 1920s, and became a widespread concern in the 1950s, when the water levels were lowered at unprecedented rates (Ireland et al., 1981). Since land use, surface-water availability and aquifer recharge vary, land subsidence across the valley is heterogenous, which makes monitoring crucial for managing the risk posed to infrastructures (Faunt et al., 2016). The nationwide ground deformation map created with SqueeSAR®, based on Sentinel-1 SAR images, reveals these heterogenous patterns. The vertical displacement time series in Fig. 4 demonstrate the different subsidence behaviours at two location, which appear to be influenced by varying degrees of aquifer recharge.
3.2 Post-seismic subsidence in Kumamoto (Japan)
Monitoring ground deformation on a large spatial scale benefits from GNSS calibration, since the calibration allows low frequency components of motion to be included. However, if no GNSS data are available, large scale phenomena can still be detected, such as the deformation occurring in the aftermath of the earthquakes in April 2016 in Kumamoto, Japan, shown in Fig. 5. This example was taken from the SqueeSAR® national deformation map for Japan, which is based on Sentinel -1 SAR images (Ferretti et al., 2019).
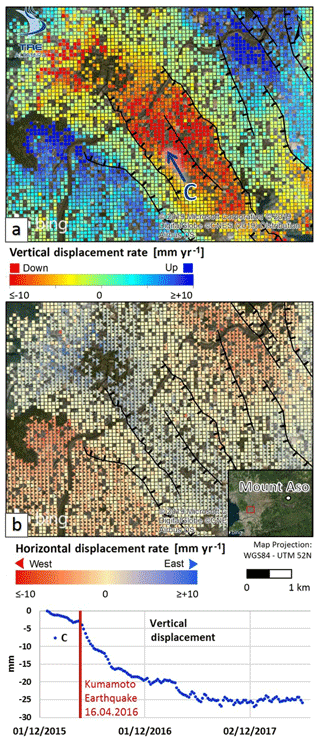
Figure 5Maps showing (a) vertical and (b) east-west displacement rates between December 2015–June 2018 over Kumamoto, Japan. The location is indicated on the overview map in bottom right corner. Black lines show faults inferred from ALOS-2 SAR interferometry by Fujiwara et al. (2016). The time series shows vertical displacement at location C, indicated in map a).
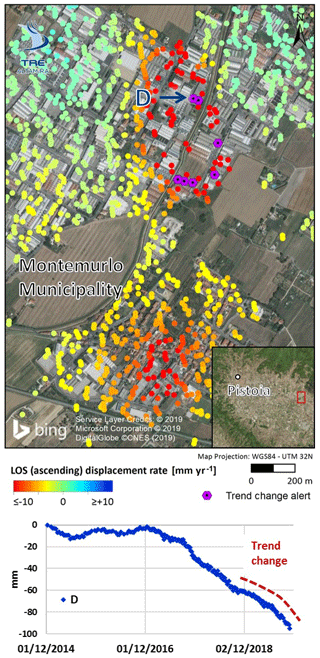
Figure 6LOS deformation map from the continuous monitoring service provided to the region of Tuscany (Italy). The location is indicated on the overview map in bottom right corner. The violet markers highlight SqueeSAR® measurement points that display a significant recent trend change in their displacement. The time series is an example of a measurement point highlighted by the trend change algorithm.
The ground displacement patterns shown in Fig. 5 match well with the results of ALOS-2 interferometry published by Fujiwara et al. (2016). The structures shown in Fig. 5 are secondary faults interpreted from the results of the ALOS-2 interferograms by Fujiwara et al. (2016). According to the authors, these faults form a graben group and are not directly related to the main faults on which the earthquakes occurred. The SqueeSAR® time series in Fig. 5 shows that there is approximately −15 mm vertical displacement between April and December 2016. A second phase of relatively fast vertical displacement started in March 2017, however the cause for this is unknown (see time series in Fig. 5). From June 2017 onwards, the area appears to stabilise.
Fujiwara et al. (2016) point out that there remains a question as to whether these secondary fault systems could become “primary” earthquake faults in the future. While there is currently no concrete answer for this, Fujiwara et al. (2016) suggest that preparing for the possibility is important. Routinely monitoring displacement using InSAR may help to better understand and predict the behaviour of these structures.
3.3 Local subsidence in the region of Tuscany (Italy) automatically detected by a trend change algorithm
Despite the benefits of a dense spatial coverage of SqueeSAR® measurement points over wide areas, filtering the data for relevant information can become challenging. This is especially important if deformation maps are updated more regularly, such as for the service provided to the region of Tuscany (Italy), where SqueeSAR® deformation maps based on Sentinel-1 SAR images are updated every two weeks. This continuous monitoring generates a stream of data that needs to be filtered for significant changes in the displacement trends. This task is currently performed by an automatic trend change detection algorithm. Variables such as the magnitude of the trend change deemed significant, or the time period considered, can be changed depending on the phenomena of interest (Del Soldato et al., 2019).
The map in Fig. 6 shows the displacement rate along ascending LOS, over an industrial area in the Montemurlo Municipality, Tuscany. The time series in the graph in Fig. 6 shows that after negative displacement started in December 2016, there has been another more recent trend change, which was highlighted by the trend change algorithm.
The negative displacement in LOS shown in Fig. 6 is likely to be related to the over-pumping of water to meet the needs of local textile factories, which are numerous in the Montemurlo Municipality (Del Soldato et al., 2019). The displacement pattern observed in this industrial estate (see Fig. 6) is discussed as a case study in more detail by Del Soldato et al. (2019).
Deformation can now be mapped routinely on a national scale with the frequent and reliable coverage provided by ESA's Sentinel-1 SAR sensors, both in LOS and vertical- and east-west direction. Producing these maps is possible with bespoke cloud-based software using the SqueeSAR® algorithm, which allows scalable processing of the large SAR image stacks. By calibrating the SqueeSAR® data with absolute GNSS data, the deformation maps can be integrated into existing monitoring programmes. Furthermore, GNSS calibration allows the monitoring of large-scale, low frequency deformation patterns with unprecedented spatial resolution. In addition, the great amount of data generated in this way can be filtered for recent localised displacement trend changes, as for example caused by landslides.
All data presented in this paper have been obtained in the framework of commercial projects. Results cannot be shared or distributed by the authors without written consent of the final users.
CB wrote the paper and was in charge of data calibration and reporting activities together with CG and AU. FN was in charge of the team who processed all InSAR data stacks. FM provided information about the Denmark dataset and its challenges. AF acted as supervisor and reviewed the manuscript.
The authors declare that they have no conflict of interest.
This article is part of the special issue “TISOLS: the Tenth International Symposium On Land Subsidence – living with subsidence”. It is a result of the Tenth International Symposium on Land Subsidence, Delft, the Netherlands, 17–21 May 2021.
The authors would like to thank the whole technical team of TRE ALTAMIRA for their support in processing all InSAR datasets mentioned in this paper.
Faunt, C. C., Sneed, M., Traum, J., and Brandt, J. T.: Water availability and land subsidence in the Central Valley, California, USA, Hydrogeol. J., 24, 675–684, https://doi.org/10.1007/s10040-015-1339-x, 2016.
Ferretti, A.: Satellite InSAR Data – Reservoir Monitoring from Space, EAGE Publications bv., 156 pp.,2014.
Ferretti, A., Fumagalli, A., Novali, F., Prati, C., Rocca, F., and Rucci, A.: A new algorithm for processing interferometric data-stacks: SqueeSAR, IEEE T. Geosci. Remote, 49, 3460–3470, https://doi.org/10.1109/TGRS.2011.2124465, 2011.
Ferretti, A., Novali, F., Giannico, C., Uttini, A., Iannicella, I., and Mizuno, T.: A squeesar database over the entire japanese territory, in: IGARSS 2019, IEEE, Yokohama, 28 July–2 August 2019, https://doi.org/10.1109/IGARSS.2019.8900052, 2019.
Fujiwara, S., Yarai, H., Kobayashi, T., Morishita, Y., Nakano, T., Miyahara, B., Nakai, H., Miura, Y., Ueshiba, H., Kakiage, Y., and Une, H.: Small-displacement linear surface ruptures of the 2016 Kumamoto earthquake sequence detected by ALOS-2 SAR interferometry, Earth Planets Space, 68, p. 160, https://doi.org/10.1186/s40623-016-0534-x, 2016.
Hanssen, R. F.: Radar Interferometry – Data Interpretation and Error Analysis, Technische Universiteit Delft, available at: http://resolver.tudelft.nl/uuid:a83859d5-c034-427e-b6a9-114c4b008d19 (last access: 20 January 2020), 330 pp., 2001.
Ireland, R. L., Poland, J. F., and Riley, F. S.: Land subsidence in the San Joaquin Valley, California, as of 1980, US Geol. Surv. Prof. Pap., 437, 82–370, https://doi.org/10.3133/ofr82370, 1981.
Del Soldato, M., Solari, L., Raspini, F., Bianchini, S., Ciampalini, A., Montalti, R., Ferretti, A., Pellegrineschi, V., and Casagli, N.: Monitoring Ground Instabilities Using SAR Satellite Data: A Practical Approach, ISPRS Int. J. Geo-Inf., 8, 307, https://doi.org/10.3390/ijgi8070307, 2019.
Teatini, P., Castelletto, N., Ferronato, M., Gambolati, G., Janna, C., Cairo, E., Marzorati, D., Colombo, D., Ferretti, A., Bagliani, A., and Bottazzi, F.: Geomechanical response to seasonal gas storage in depleted reservoirs: A case study in the Po River basin, Italy, J. Geophys. Res.-Earth, 116, 1–21, https://doi.org/10.1029/2010JF001793, 2011.
Yague-Martinez, N., Prats-Iraola, P., Gonzalez, F. R., Brcic, R., Shau, R., Geudtner, D., Eineder, M., and Bamler, R.: Interferometric Processing of Sentinel-1 TOPS Data, IEEE T. Geosci. Remote, 54, 2220–2234, https://doi.org/10.1109/TGRS.2015.2497902, 2016.