the Creative Commons Attribution 4.0 License.
the Creative Commons Attribution 4.0 License.
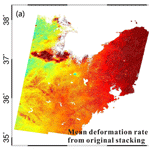
General Survey of Large-scale Land Subsidence by GACOS-Corrected InSAR Stacking: Case Study in North China Plain
Ruya Xiao
Chen Yu
Zhenhong Li
Chuang Song
Xiufeng He
Satellite-based InSAR (Interferometric Synthetic Aperture Radar) provides an effective way to measure large-scale land surface motions. Currently, the atmospheric phase delay is one of the most critical issues in InSAR deformation monitoring. Generic Atmospheric Correction Online Service (GACOS) is a free, globally available and easy-to-implement tool to generate high-resolution zenith total delay maps, which could be used for InSAR atmospheric delay correction. The mean velocity could then be estimated by stacking multiple GACOS-corrected interferograms. We applied the proposed GACOS-corrected InSAR stacking method in the North China Plain and analysed its performance. Within the 549 interferograms, more than 85 % gained positive correction performances. The correlation between the phase-dZTD indicator and the performance reached 0.89, demonstrating a significant relationship. Deformation maps revealed by InSAR stacking with and without GACOS corrections showed that GACOS could mainly remove the topography-related and long wavelength signals.
The successful operation of the European Space Agency's (ESA) Sentinel-1 satellites provide unprecedented possibilities and convenience for large-scale land surface deformation measurements. Ground motion monitoring using InSAR are extended from local, regional practices to full, nationwide scale applications. In recent years, the implementations of researches or projects covering a whole country, such as Italy (Costantini et al., 2017), Germany (Haghighi and Motagh, 2017), Norway (https://insar.ngu.no, last access: 2 March 2020) and Japan (Ferretti et al., 2019), indicate that dynamic monitoring of land surface deformation with a certain level of automation will be expected to become a routine operation. The achievements of general surveys will be the foundation and guide for detailed investigation.
Although InSAR has been proven successful in many existing cases, there are still inherent limitations in the technique. At this stage, the atmospheric phase delay, mainly affected by the differences in propagation paths through the troposphere, is one of the most critical issues in large-scale InSAR deformation monitoring.
The second part of this paper briefly introduces InSAR atmospheric effects and the existing methods for mitigation. Section 3 describes the data processing strategy of the proposed GACOS-corrected InSAR stacking method. Study area, satellite data used, and results are presented in Sect. 4, followed by the conclusions in Sect. 5.
The propagation paths of the microwave signals of the SAR sensors on the satellite are affected during the 2-pass through the Earth's atmosphere, expressed as the changes in the transit time, which is always known as the atmospheric effects of InSAR (Li et al., 2005). The tropospheric effects are mainly related to the variations of the water vapour in the troposphere, as well as the temperature and pressure changes at different SAR image acquisition time. Generally, the atmospheric effect could result in up to 15–20 cm errors in the interferograms, which may interfere with or even make the signals of interest indistinguishable. Researchers have developed numerous means to mitigate atmospheric effects. According to the data used, there are mainly two types of methods: atmospheric corrections with or without external data.
No external data employed means there are no other atmospheric parameter measurements, such as water vapour and temperature, involved in the processing. This kind of phase-based corrections, such as phase-elevation estimate (Bekaert et al., 2015) or PSI (Ferretti et al., 2001), are always based on certain assumptions. As its name suggests, the major benefit of this type of methods is that external data are not needed, and it is straightforward and easy-to-implement. However, the disadvantages are obvious: (i) this method may have the risk that the signal of interest is removed, (ii) in some scenarios, it is difficult or impossible to evaluate the performance of atmospheric signal removal quantitatively.
The external data used in InSAR atmospheric correction mainly includes three types of data, i.e., satellite-based spectrometer observations, ground observations and numerical meteorological models. MEdium Resolution Imaging Spectrometer (MERIS) (Li et al., 2012) and Moderate Resolution Imaging Spectroradiometer (MODIS) offer near-IR water vapour products at a fine resolution (∼ 1 km) and high accuracy (∼ 1 mm), but it is sensitive to the presence of clouds and daytime available only. Ground-based atmospheric parameters used in InSAR atmospheric corrections refer to observations from meteorological stations and GNSS ZTD. However, even the densest GPS networks are overshadowed compared to the spatial resolution of SAR remote sensing images. These numerical weather models, such as widely used ERA-Interim (ERA-I) released by the European Center for Medium-Range Weather Forecasts (ECMWF) (Jolivet et al., 2011), have the properties of high availability, relative “continuity”, and insensitivity to the clouds, making them popular atmospheric delay correction data sources.
Although there are successful cases, every type of correction methods has limitations and cannot always promise success (Murray et al., 2019). Moreover, the processing of these external data further increases the complexity of InSAR data processing procedure. The development of Generic Atmospheric Correction Online Service for InSAR (GACOS), to some extent, has solved the above problems.
Launched in June 2017, GACOS has distributed more than 60 000 tasks all over the world. Together with DEM data, it utilises atmospheric model High RESolution 10-day forecast (HRES) datasets, which is ECMWF's highest-resolution model of up to lat/long grid, 137 levels at every 6 h. The innovative Iterative Tropospheric Decomposition (ITD) model is implemented to separate stratified and turbulent components from tropospheric delays (Yu et al., 2017), and then high spatial resolution (default 90 m) ZTD maps are generated. GACOS is not only globally available and near real-time but also free and easy-to-implement, that is, the users only need to submit a request with the location of their study area and the acquisition time of SAR data, and then get the ZTD products. More information can be found on http://ceg-research.ncl.ac.uk/v2/gacos/ (last access: 2 March 2020).
The averaging of multiple interferograms (stacking) is the simplest attempt to remove the influences of errors such as the atmosphere in time-series InSAR analysis. In this model, the signal of interest, i.e., deformation in the interferograms, is assumed to have a systematic pattern, and the atmospheric noise is random. The method of least squares could significantly increase the signal-to-noise ratio by reducing the random noises (Wright et al., 2001). Mean velocity map is the most intuitive way to show the characteristics of deformation. A constant rate of each pixel is estimated by N individual interferograms following Eq. (1):
where Vmean is the mean velocity, φi demonstrates the unwrapped phase, and ΔTi is the temporal baseline of the ith interferogram. In Eq. (1), the interferometric phases are weighted by the time interval.
As shown in Fig. 1, we proposed a new method estimating the deformation rate by InSAR stacking with GACOS-corrected interferometric phases. The interferograms generation process is similar to the traditional small baseline subset method. After phase unwrapping, the phases are corrected pair by pair using GACOS differential ZTDs (dZTDs). We can employ performance indicators to evaluate the effectiveness of the corrections and decide whether to apply the correction to one specific interferogram or not. Finally, the mean velocities are estimated using the updated phases.
4.1 Study area and data used
As China's largest alluvial plain, the North China Plain covers the area of 32–40∘ N, 114–121∘ E, and is inhabited by more than 20 % population of China. The region is flat, most of which are less than 50 m, and the eastern part is less than 10 m above sea level.
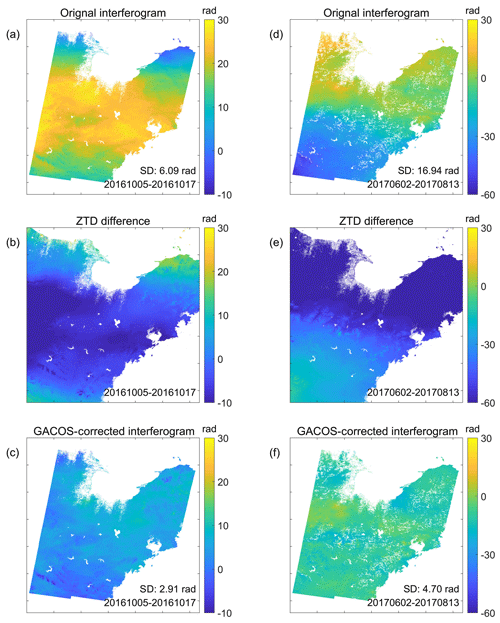
Figure 5GACOS ZTD difference (b, e) and interferograms before (a, d) and after (c, f) corrections for two pairs with different baselines.
We explore the study area with SAR images acquired on 66 days between October 2016 and March 2019, by Sentinel-1b. SAR imaging time is 22:04 UTC. Figure 2 demonstrates the location and topography of the study area. The red rectangle region involves 55 bursts in 3 sub-swaths. We multi-look the interferograms by the factor of 40 (range) and 8 (azimuth), which refers to the resolution of ∼ 120 m. Totally 549 interferograms are formulated with the restriction that temporal baselines are less than 300 days and at most nine interferometric pairs are generated for each date. The spatial and temporal baselines are shown in Fig. 3. All the interferometric phases are unwrapped using minimum cost flow with the coherence threshold of 0.4.
4.2 Results
4.2.1 Phase standard deviations of interferograms
The phase standard deviations of interferograms before and after correction are always used for assessment of the performance of atmospheric signal removal. Considering the existing deformation signals in the interferograms, we mask out the fast deforming areas with a mean velocity larger than 30 mm yr−1 (using a preliminary deformation result) for the interferograms with a temporal baseline of more than 24 days (431 interferograms), before calculating the standard deviations. The results are binned to 7 levels, as shown in Fig. 4. The positive values of the performance indicate improvements, and GACOS corrections played a positive role (the phase standard deviations decreases after correction) for more than 85 % interferograms in this case. Moreover, in the 78 negative performance pairs, there are 36 interferograms (6.6 % of the total population) whose standard deviations are less than 10 mm (2.28 rad) or the performance is larger than −10 %, which means there is, in fact, no significant difference between the corrected and original results.
Two InSAR atmospheric correction examples are shown in Fig. 5. The first column (Fig. 5a–c) shows the interferogram generated by SAR images dated on 5 and 17 October 2016 with a spatial baseline of −11.6 m. After correction (Fig. 5c), the standard deviation of the interferogram decreased by 52.24 % to 2.91 rad. In the second case (Fig. 5d–f), the standard deviation of GACOS-corrected interferogram is only about 1∕4 of that of the original interferogram. For the full population of the 549 interferograms, the median and mean of the performance are 31.15 % and 27.29 %, respectively.
4.2.2 Correlation analysis of the phase-dZTD indicator and performance
The correlation between the interferometric phases and dZTDs could be used as an indicator for the applicability of GACOS (Yu et al., 2018). Here we investigate the correlation between the indicator and GACOS performance. Figure 6 shows that the mean correlation (indicator) of the 549 interferograms is 0.71, and the mean GACOS performance is 27.29 %. The correlation between them is 0.89, determining that the performance has a significant relationship with the indicator. Statistics from the 471 interferograms with positive performances demonstrate that there is a nearly linear relationship between the indicator and the performance: the higher the phase-dZTD correlations, the more positive effect of the GACOS corrections.
4.2.3 Deformation revealed by GACOS-Corrected InSAR Stacking
The LOS deformation maps are revealed by stacking from the original and corrected interferograms, as shown in Fig. 7. We can see topography-related and long wavelength signals exist in the original deformation map (Fig. 7a), while they are largely removed by GACOS (Fig. 7b).
In Fig. 7a, the deformation pattern is obscured by the atmospheric effects, which leads to a misunderstanding of the land surface motions in the study area. GACOS solved the issue in a simple and effective way. The development of the new generation satellites as Sentinel-1 with rapid revisiting period (12 to 6 days) in the big data era, increases the attention on algorithm efficiency and estimation efficiency in the data processing practice of large-scale ground deformation general surveys. GACOS-corrected InSAR stacking, as a robust method which is straightforward and easy-to-implement, provide an effective way for general surveys of land surface deformation.
As an InSAR atmospheric correction method with external data, GACOS has the advantages of globally available, near real-time and easy to implement. In this paper, we proposed the GACOS-corrected InSAR stacking method for general surveys of the land surface deformation. The main conclusions are as follows:
-
The standard deviations of 471 interferograms decreased after GACOS corrections, i.e., more than 85 % interferograms, in this case, got positive performances.
-
The correlation between the phase-dZTD indicator and the performance was analysed, and the correlation of 0.89 demonstrated a significant relationship.
-
Deformation was revealed by InSAR stacking method from the original and corrected interferograms, showing that GACOS largely removed the topography-related and long wavelength signals.
Sentinel-1 SAR data used in this study are from European Space Agency (ESA), downloaded through Copernicus Open Access Hub at https://scihub.copernicus.eu/dhus/#/home (last access: 2 March 2020). GACOS products are provided by Newcastle University at http://ceg-research.ncl.ac.uk/v2/gacos (last access: 2 March 2020).
RX conceived and designed the experiments. RX and CY performed the experiments and analyzed the results. RX wrote the manuscript and the whole team provided suggestions and reviewed the manuscript.
The authors declare that they have no conflict of interest.
This article is part of the special issue “TISOLS: the Tenth International Symposium On Land Subsidence – living with subsidence”. It is a result of the Tenth International Symposium on Land Subsidence, Delft, the Netherlands, 17–21 May 2021.
The authors thank the reviewers and editors for their helpful comments.
This research has been supported by the National Natural Science Foundation of China (grant no. 41804005), the Fundamental Research Funds for the Central Universities (grant no. 2019B17414), and the Natural Science Foundation of Jiangsu Province (grant no. BK20170869). Ruya Xiao received support from the Chinese Scholarship Council (ref. 201806715019).
Bekaert, D. P. S., Hooper, A., and Wright, T. J.: A spatially variable power law tropospheric correction technique for InSAR data, J. Geophys. Res.-Sol. Ea., 120, 1345–1356, https://doi.org/10.1002/2014jb011558, 2015.
Costantini, M., Ferretti, A., Minati, F., Falco, S., Trillo, F., Colombo, D., Novali, F., Malvarosa, F., Mammone, C., Vecchioli, F., Rucci, A., Fumagalli, A., Allievi, J., Ciminelli, M. G., and Costabile, S.: Analysis of surface deformations over the whole Italian territory by interferometric processing of ERS, Envisat and COSMO-SkyMed radar data, Remote Sens. Environ., 202, 250–275, 2017.
Ferretti, A., Prati, C., and Rocca, F.: Permanent scatterers in SAR interferometry, IEEE T. Geosci. Remote, 39, 8–20, https://doi.org/10.1109/36.898661, 2001.
Ferretti, A., Novali, F., Giannico, C., Uttini, A., Iannicella, I., and Mizuno, T.: A SqueeSAR database over the entire Japanese territory, 2019 IEEE International Geoscience and Remote Sensing Symposium – IGARSS, Yokohama, Japan, 28 July–2 August, 2019.
Haghighi, M. H. and Motagh, M.: Sentinel-1 InSAR over Germany: Large-scale interferometry, atmospheric effects, and ground deformation mapping, zfv – Zeitschrift für Geodäsie, Geoinformation und Landmanagement, 142, 245–256, 2017.
Jolivet, R., Grandin, R., Lasserre, C., Doin, M.-P., and Peltzer, G.: Systematic InSAR tropospheric phase delay corrections from global meteorological reanalysis data, Geophys. Res. Lett., 38, L17311, https://doi.org/10.1029/2011gl048757, 2011.
Li, Z., Muller, J.-P., Cross, P., and Fielding, E. J.: Interferometric synthetic aperture radar (InSAR) atmospheric correction: GPS, Moderate Resolution Imaging Spectroradiometer (MODIS), and InSAR integration, J. Geophys. Res.-Sol. Ea., 110, B03410, https://doi.org/10.1029/2004jb003446, 2005.
Li, Z. W., Xu, W. B., Feng, G. C., Hu, J., Wang, C. C., Ding, X. L., and Zhu, J. J.: Correcting atmospheric effects on InSAR with MERIS water vapour data and elevation-dependent interpolation model, Geophys. J. Int., 189, 898–910, 2012.
Murray, K. D., Bekaert, D. P. S., and Lohman, R. B.: Tropospheric corrections for InSAR: Statistical assessments and applications to the Central United States and Mexico, Remote Sens. Environ., 232, 111326, https://doi.org/10.1016/j.rse.2019.111326, 2019.
Wright, T., Parsons, B., and Fielding, E.: Measurement of interseismic strain accumulation across the North Anatolian Fault by satellite radar interferometry, Geophys. Res. Lett., 28, 2117–2120, 2001.
Yu, C., Penna, N. T., and Li, Z.: Generation of real-time mode high-resolution water vapor fields from GPS observations, J. Geophys. Res.-Atmos., 122, 2008–2025, https://doi.org/10.1002/2016jd025753, 2017.
Yu, C., Li, Z. H., Penna, N. T., and Crippa, P.: Generic Atmospheric Correction Model for Interferometric Synthetic Aperture Radar Observations, J. Geophys. Res.-Sol. Ea., 123, 9202–9222, https://doi.org/10.1029/2017jb015305, 2018.