the Creative Commons Attribution 4.0 License.
the Creative Commons Attribution 4.0 License.
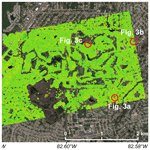
InSAR Detection of Localized Subsidence Induced by Sinkhole Activity in Suburban West-Central Florida
Talib Oliver-Cabrera
Shimon Wdowinski
Sarah Kruse
Tonian Robinson
Sinkhole activity in west-central Florida is a major hazard for people and property. Increasing frequency of sinkhole collapse is often related to an accelerated use of groundwater and land resources. In this work, we use radar interferometry acquired over a selected region in Hernando County in west-central Florida to observe small localized deformation possibly caused by sinkhole activity. The data used for the study consist of acquisitions from one TerraSAR-X frame covering a time span of approximately 1.7 years with spatial resolution of 0.25 by 0.60 m. We applied the Persistent Scatterer Interferometry (PSI) technique using the Stanford Method for Persistent Scatterers (StaMPS). Results reveal several areas of localized subsidence at rates ranging from −3.7 to −4.9 mm yr−1. Ground truthing and background verification of the subsiding locations confirmed the relationship of the subsidence with sinkhole presence.
- Article
(9028 KB) - Full-text XML
- BibTeX
- EndNote
Sinkholes form when rocks or sediments move into a void created by rock dissolution (Dobecki and Upchurch, 2006). They can induce subsidence in the form of gradual surface drop with no apparent rupture, which may later collapse due to a break of rock and soil (Ford and Williams, 2007). In Florida, sinkhole activity occurs frequently due cap rock subsidence or collapse into existing cavities within the shallow carbonate bedrock, which were formed during the Holocene and Pleistocene. Accelerated use of groundwater and land resources has promoted an increase in the rate of sinkhole formation (Tihansky, 1999; Veni et al., 2014). These circumstances have made sinkhole collapse one of the leading natural disasters in Florida, with almost 25 000 insurance claims between the years 2006–2010 (Florida Office of Insurance Regulation, 2010). Sinkholes are present through much of Florida. However, reported sinkhole incidences as subsidence and collapse occur mainly in the west-central region of the state (Florida Geological Survey, 2015) (Fig. 1). This sinkhole-active corridor extends over a large area (hundreds of km2) covering three of the most densely populated cities in Florida: Orlando, Tampa and St. Petersburg, which is located west of Tampa (Fig. 1).
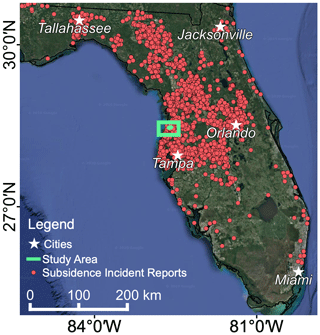
Figure 1Location map of sinkhole and subsidence reports in Florida (red circles) collected by the Florida Geological Survey (Florida Geological Survey, 2015). White stars show the location of the largest cities in the state. Green frame shows the location of the Timber Pines study area. Map data: © Google, Maxar Technologies.
Detecting a sinkhole before collapse is a difficult task, as sinkholes often display unnoticeable surface changes. Geophysical techniques such as ground penetrating radar (GPR), electrical resistivity tomography (ERT), and shallow seismic surveys (refraction tomography, reflection, surface wave inversion) are commonly used to image with high detail sinkhole characteristics beneath the surface (Dobecki et al., 2006; Theron and Engelbrecht, 2018). However, these techniques require a priori information of sinkhole location and are limited to small study areas (up to hundreds of m2). Geological and geophysical information is often used for sinkhole hazard and risk assessments, based on modelling and probabilistic approaches (Frumkin et al., 2011; Galve et al., 2011; Kim and Nam, 2014; Theron and Engelbrecht, 2018). Calculated hazard and risk maps rely on sinkhole information that is often incomplete or not available. Small sinkhole size and sparse distribution makes detection and monitoring a challenging task. In places such as Florida, monitoring techniques are needed that could cover large areas to complement and support ground-based surveys.
Interferometric Synthetic Aperture Radar (InSAR) technique is a remote sensing technique that can detect surface movements with high detail, while covering vast areas (Massonnet and Feigl, 1998; Bürgmann et al., 2000; Rosen et al., 2000). The technique has been successfully applied to observe sinkhole activity in arid places such as west Texas and the Dead Sea shores (Nof et al., 2013; Atzori et al., 2015; Baer et al., 2018; Kim and Lu, 2018), in urban settings like Heerlen in Netherlands, Gauteng province in South Africa and Quebec City in Canada (Chang and Hanssen, 2014; Theron et al., 2017; Martel et al., 2018) and in vegetated regions like Bayou Corne, Louisiana and Tampa, Florida (Jones and Blom, 2013; Oliver-Cabrera et al., 2019). The above InSAR implementation examples were conducted in areas where interferometric coherence is maintained over long periods of time, through stable radar scattering from bare surfaces, rocks, and man-made structures. In cases where land-cover changes occur rapidly and frequently, as in highly vegetated regions such as Louisiana or Florida, effective InSAR tends to be limited to areas with buildings (e.g. Oliver-Cabrera et al., 2019). An alternative to maintain high coherence in densely vegetated regions are radar systems with a very short revisit time, like the Uninhabited Aerial Vehicle SAR (UAVSAR) (Jones and Blom, 2013).
This study expands upon our previous study of InSAR-based detection of sinkhole activity in west-central Florida (Oliver-Cabrera et al., 2019), which demonstrated the capability of InSAR for detecting sinkhole related deformation in the challanging scattering environment of west-central Florida. In the current study we focus on a smaller area, the region of the Timber Pines housing development in west-central Florida, and analyze the effectivity of the InSAR retults, which are verified using both ground based observations and official reports of sinkhole induced damage.
The study area covers the region of Timber Pines in Hernando County, roughly 60 km north of Tampa in west-central Florida. Timber Pines is a private community that hosts 3452 homes, characterized by sparse residential and commercial buildings. This study site was selected because numerous homes have reported sinkhole activity. Also, the county provides openly available lists of reported sinkholes, allowing us to verify the deformation sources of our InSAR analysis.
Our data consists of TerraSAR-X (TSX) (3.1 cm wavelength) Staring SpotLight (ST) SAR imagery. TSX ST mode has a pixel resolution of 0.25 m in azimuth and 0.60 m range. A total of 45 acquisitions were obtained to cover a time span of 1.7 years between 2015–2017 with a repeated pass of 11 and 22 d. Variation in the repeated pass acquisition frequency its due to budget constraints. Sinkhole activity verification was done through the sinkhole reports provided by Hernando County through their website (https://www.hernandocounty.us/, last access: September 2019).
Our InSAR processing and analysis follows the proposed methodology of Oliver-Cabrera et al. (2019). SAR data processing to obtain InSAR time series is followed by a spatial clustering analysis. First, a stack of single master interferometric pairs is generated using the Doris (v4.02) software package to generate interferograms. Doris was developed by the Delft Institute of Earth Observation and Space Systems (DEOS) Delft University of Technology (Kampes et al., 2003). The selected master is a SAR acquisition with low noise levels and centered, as much as possible, in the middle of the time vector. No resampling (multi-looking) was applied to the interferometric products. The stack of single master interferograms was used as an input for the second processing stage, time series analysis using the Persistent Scatterer Interferometry method (PSI) (Ferretti et al., 2001). This technique focuses on the use of high backscatter signature sources (e.g. buildings), minimizing the use of pixels with low or variable backscatter (e.g. vegetation). Thus, only the Persistent Scatterers (PS) are kept. We implement the Stanford Method for PS (StaMPS) (Hooper et al., 2004) in order to estimate the displacement time series of individual points.
A PS distribution analysis post-processing is performed to find the possible sinkhole-related signals. We isolate the scatterers that display deformation trends from the stable ones, by separating the scatterers with deformation trends beyond 3 standard deviations (3σ), leaving just the peak moving scatterers. The applied threshold of 3σ was selected from a range of thresholds between 1 to 3σ as it was found most suitable to isolate the moving scatterers. To find the sinkhole-related signals we search for groups of subsiding scatterers that are concentrated spatially. A Density-Based Spatial Clustering Analysis (DBSCAN) algorithm (Ester et al., 1996) is implemented to find groups of moving scatterers based on their spatial distribution. The criteria used in the DBSCAN algorithm is a distance of 6 m, double of the average sinkhole radius reported in the Florida subsidence reports (Florida Geological Survey, 2015) and a minimum of five PS points per group.
InSAR time series results are shown as a map of surface velocities (Fig. 2). The velocity map indicates stability in most of the study area (green color in Fig. 2). Results also display points of high positive and negative velocities, which likely represent noise due to the short duration of the acquisition span (1.7 years). Small scattered areas of localized deformation can be found throughout the study area (e.g. red circles in Fig. 2). A total of 85 clusters of subsidence were obtained from the spatial clustering analysis. Results show that the majority of the clusters are located over houses. A few of the clusters are located over roads, including one with 46 PSs (Site a). From all the clusters with more than 15 PSs, just one appeared to be part of a noisy area, considered as non-reliable. Three clusters with a higher density of subsiding scatterers were selected for a detailed inspection (Sites a, b and c in Fig. 3). Site a is located in the middle of a road, approximately 30 m long. Site b and c are located in two houses of roughly 20×20 m of size. Detailed inspection of the data shows a peak downwards movement starting on the first quarter of 2016 and reaching the lowest point around August 2016. The county database does not show a sinkhole report for this area, however, there are several nearby sinkhole activity reports. Also, Oliver-Cabrera et al. (2019) found that GPR surveys show irregular strata along the road near the signal and from neighboring homes, which suggest prior and perhaps ongoing subsidence at this site. Time series observations of Site b show a downwards step-like movement in the second half of 2015. County reports confirmed sinkhole activity at this location, however no specifics regarding dates of events or collapses were found. Observations from Site c also show a step-like movement, but in this case towards the end of the first half of 2016. County documents for this site also reported sinkhole activity. A sharp peak up and down in 2016 suggest that an abrupt change happened to the building, perhaps related to repair works to the property, although no documentation was found to confirm this.
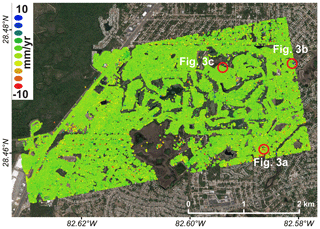
Figure 2Velocity map of the Timber Pines study area determined from TSX ST data covering a time span of 1.7 years. Red circles show the location of the clusters with highest density of subsiding scatterers. Map data: © Google, Maxar Technologies.
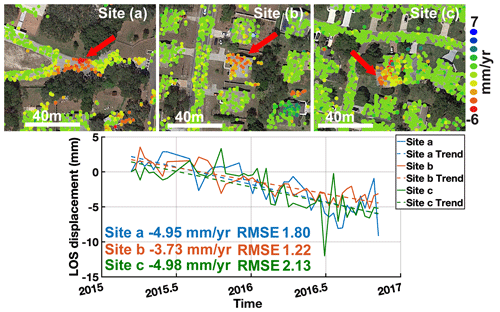
Figure 3Zoom-in to the clusters with highest density of subsiding scatterers, sites a, b and c. The red arrow shows the scatterers shown in the time series plot. Detected pixel movement time series shows subsiding trends of −4.95, −3.73 and −4.98 mm yr−1, respectively. Map data: © Google, Maxar Technologies.
Overall, results show that the technique effectively detects localized deformation and that the calculated clusters coincide with reported sinkhole locations. InSAR derived deformation for this area is limited mainly to constructed areas. Sources of displacement can be related to activity that is not associated with sinkholes, so field and background verification surveys are essential to properly define the observed deformation sources. InSAR alone is not sufficient for determining sinkhole presence. However, InSAR yields highly valuable information of location, size and magnitude of displacement from suspicious locations and provides valuable warning information. Compared to other studies, deformation observed in this work is constrained to very small areas, which together with the densely vegetated ground cover of suburban Florida makes the use of high-resolution data a key component of the analysis. The work by Oliver-Cabrera et al. (2019) explains the level of impact that high-resolution data has on areas like Timber Pines. An image with spatial resolution of 2.5×22 m (e.g. Sentinel-1) is able to detect 152 PS per km2 while and image with resolution of 0.25×0.6 m (e.g. TSX ST) can detect 69 733 PS per km2.
High-resolution InSAR time series analysis combined with a clustering algorithm appears to be a promising tool for detecting sinkhole activity. The use of high-resolution InSAR allows us to observe small subsidence signals over the size of a small house or less. Due to the short duration of the InSAR time series (1.7 year), the InSAR detected velocity maps tend to be noisy. However, the clustering algorithm allowed us to eliminate much of the noise in the results by identifying subsiding clusters, which are characteristics of subsiding buildings. The time series analysis of the TSX ST over Timber Pines reveals localized deformation at several sites. Velocity trends of three selected sites that were observed in detail, yield subsidence rates ranging between −3.7 to −4.9 mm yr−1. A cross checking with Hernando County sinkhole reports confirmed sinkhole presence in the vicinity of these three sites. Our results suggest that high-resolution InSAR subsidence detection combined with clustering analysis can serve as a useful tool for detecting sinkhole activity in challenging scattering environments, as in west-central Florida.
The principal data used in this study are X-band 10 SAR imagery provided by the German Space Agency (DLR). The actual data cannot be shared due to restrictions imposed by DLR. However, lists of SAR images used for this study are presented in the sinkhole detection website at FIU Geodesy Lab (FIUGL) within the DATA page website – http://geodesy.fiu.edu/FloridaSinkholes/dataset.html (FIU Geodesy Lab, 2020).
TOC processed the InSAR data. TOC and SW conducted the data analysis and wrote the manuscript. SK selected the study areas. SK and TR conducted the ground verifications.
The authors declare that they have no conflict of interest.
This article is part of the special issue “TISOLS: the Tenth International Symposium On Land Subsidence – living with subsidence”. It is a result of the Tenth International Symposium on Land Subsidence, Delft, the Netherlands, 17–21 May 2021.
Authors thank the German Aerospace Center (DLR) for the access to TerraSAR-X imagery through the GEO3330 project and UNAVCO for downloading and archiving the data. First author would like to acknowledge Conacyt and Fulbright for supporting this research.
This is contribution number 951 from the Southeast Environmental Research Center in the Institute of Environment at Florida International University.
This research has been supported by the National Science Foundation, Directorate for Geosciences (grant nos. EAR-1620617 and EAR-1713420).
Atzori, S., Antonioli, A., Salvi, S., and Baer, G., InSAR-Based Modeling and Analysis of Sinkholes Along the Dead Sea Coastline, Geophys. Res. Lett., 42, 8383–8390, 2015.
Baer, G., Magen, Y., Nof, R. N., Raz, E., Lyakhovsky, V., and Shalev, E.: InSAR Measurements and Viscoelastic Modeling of Sinkhole Precursory Subsidence: Implications for Sinkhole Formation, Early Warning, and Sediment Properties, J. Geophys. Res.-Earth Surf., 123, 678–693, 2018.
Bürgmann, R., Rosen, P. A., and Fielding, E. J.: Synthetic aperture radar interferometry to measure Earth’s surface topography and its deformation, Annu. Rev. Earth Planet. Sci., 28, 169–209, 2000.
Chang, L. and Hanssen, R. F.: Detection of Cavity Migration and Sinkhole Risk Using Radar Interferometric Time Series, Remote Sens. Environ., 147, 56–64, 2014.
Dobecki, T. L. and Upchurch, S. B.: Geophysical Applications to Detect Sinkholes and Ground Subsidence, The Leading Edge, 25, 336–341, 2006.
Ester, M., Kriegel, H.-P., Sander, J., and Xu, X.: A density-based algorithm for discovering clusters in large spatial databases with noise, KDD, 96, 226–231, 1996.
Ferretti, A., Prati, C., and Rocca, F.: Permanent Scatterers in SAR Interferometry, IEEE T Geosci Remote, 39, 8–20, 2001.
FIU Geodesy Lab: Lists of SAR Images Datasets, available at: http://geodesy.fiu.edu/FloridaSinkholes/dataset.html, last access: February 2020.
Florida Geological Survey: Florida Subsidence Incident Reports Geospatial Data Presentation Form: vector digital data, available at: http://publicfiles.dep.state.fl.us/otis/gis/data/FGS_SUBSIDENCE_INCIDENTS.zip (last access: October 2019), FDEP, Florida Geological Survey, 2015.
Florida Office of Insurance Regulation: Report on Review of the 2010 Sinkhole Data Call, retrieved from: https://www.floir.com/siteDocuments/Sinkholes/2010_Sinkhole_Data_Call_Report.pdf (last access: October 2019), 2010.
Ford, D. and Williams, P.: Karst Hydrogeology and Geomorphology, Chichester: John Wiley & Sons, 2007.
Frumkin, A., Ezersky, M., Al-Zoubi, A., Akkawi, E., and Abueladas, A. R.: The Dead Sea sinkhole hazard: Geophysical assessment of salt dissolution and collapse, Geomorphology, 134, 102–117, 2011.
Galve, J. P., Remondo, J., and Gutiérrez, F.: Improving sinkhole hazard models incorporating magnitude-frequency relationships and nearest neighbor analysis, Geomorphology, 134, 157–170, 2011.
Hooper, A., Zebker, H., Segall, P., and Kampes, B.: A New Method for Measuring Deformation on Volcanoes and other Natural Terrains Using InSAR Persistent Scatterers, Geophys. Res. Lett., 31, 1–5, 2004.
Jones, C. E. and Blom, R. G.: Bayou Corne, Louisiana, Sinkhole: Precursory Deformation Measured by Radar Interferometry, Geology, 42, 111–114, 2013.
Kampes, B. M., Hanssen, R. F., and Perski, Z.: Radar Interferometry with Public Domain Tools, Proceedings of FRINGE, 3, 59–68, 2013.
Kim, J.-W. and Lu, Z.: Association between localized geohazards in West Texas and human activities, recognized by Sentinel-1A/B satellite radar imagery, Scientific Reports, 8, 4727, https://doi.org/10.1038/s41598-018-23143-6, 2018.
Kim, Y. and Nam, B. H.: A Comparative Study of Karst Sinkhole Hazard Mapping Using Frequency Ratio and Artificial Neural Network for East Central Florida, 105–114, 2014.
Martel, R., Castellazzi, P., Gloaguen, E., Trépanier, L., and Garfias, J.: ERT, GPR, InSAR, and tracer tests to characterize karst aquifer systems under urban areas: The case of Quebec City, Geomorphology, 310, 45–56, 2018.
Massonnet, D. and Feigl, K. L.: Radar interferometry and its application to changes in the Earth's surface, Rev. Geophys., 36, 441–500, 1998.
Nof, R. N., Baer, G., Ziv, A., Raz, E., Atzori, S., and Salvi, S.: Sinkhole Precursors Along the Dead Sea, Israel, Revealed by SAR Interferometry, Geology, 41, 1019–1022, 2013.
Oliver-Cabrera, T., Wdowinski, S., Kruse, S., and Robinson, T.: Detection of Sinkhole Activity in West-Central Florida Using InSAR Time Series Observations, Geomorphology, submitted, 2019.
Rosen, P. A., Hensley, S., Joughin, I. R., Li, F. K., Madsen, S. N., Rodriguez, E., and Goldstein, R. M.: Synthetic aperture radar interferometry, Proceedings of the IEEE, 88, 333–382, 2000.
Theron, A. and Engelbrecht, J.: The Role of Earth Observation, with a Focus on SAR Interferometry, for Sinkhole Hazard Assessment, Remote Sensing, 10, 1506, https://doi.org/10.3390/rs10101506, 2018.
Theron, A., Engelbrecht, J., Kemp, J., Kleynhans, W., and Turnbull, T.: Detection of Sinkhole Precursors Through SAR Interferometry: Radar and Geological Considerations, IEEE Geosci. Remote Sens. Lett., 14, 871–875, 2017.
Tihansky, A. B.: Sinkholes, West-Central Florida, Land Subsidence in the United States: US Geological Survey Circular, 1182, 121–140, 1999.
Veni, G., Brashear, C. C., and Glasbrenner, D.: Building Codes to Minimize Cover Collapses in Sinkhole-Prone Areas, 14th Sinkhole Conference, 471–476, 2014.