the Creative Commons Attribution 4.0 License.
the Creative Commons Attribution 4.0 License.
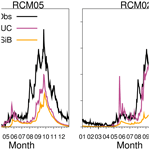
Uncertainty in simulated streamflow using runoff driven by the outputs of a high-resolution regional climate model
Aulia Febianda Anwar Tinumbang
Kazuaki Yorozu
Yasuto Tachikawa
Yutaka Ichikawa
Hidetaka Sasaki
Tosiyuki Nakaegawa
Estimating river discharge using climate model output can aid in analyzing the potential impacts of climate change on water-related disasters. This study aimed to explore the uncertainty in simulated streamflow using the non-hydrostatic regional climate model (NHRCM) outputs in Thailand. The NHRCM was simulated at 5- and 2 km resolutions. To estimate runoff, two land surface models (LSMs) were employed: the Meteorological Research Institute–Simple Biosphere Model (MRI-SiB) in NHRCM and the Simple Biosphere including Urban Canopy (SiBUC). The NHRCM rainfalls captured the seasonal pattern of rainfall in the upper Ping River Basin, although they were underestimated. The 2 km NHRCM had less rainfall, but it captured the local topography better than the 5 km model. This difference in rainfall affected the simulated streamflow. Furthermore, the uncertainty of the simulated streamflow was influenced by the different runoff schemes used by the LSMs. For instance, MRI-SiB incorporates a direct infiltration structure from the surface to the second soil layer and estimates subsurface runoff using hydraulic diffusion and gravitational flow, while SiBUC uses a gravitational-only subsurface runoff approach. These variations led to significant disparities in surface and subsurface runoff. Future work should enhance the accuracy of rainfall from climate models and runoff from LSMs for assessing the potential impacts of climate change on water-related disasters.
- Article
(3949 KB) - Full-text XML
- BibTeX
- EndNote
As global warming continues, water cycle variability and extremes will increase, causing more frequent and severe water-related disasters such as floods and droughts (IPCC, 2021). The impact of climate change is expected to vary by region, making local projections crucial. Climate models, such as general circulation models (GCMs) and regional climate models (RCMs), are the primary tools for assessing the potential impacts of climate change. RCMs are often used to investigate the local impacts of global warming owing to their high spatial resolution, which enables the capture of regional climatic features.
To mitigate and develop adaptation strategies against water-related disasters, estimating the extent to which river discharge will change owing to global warming is crucial. Much research has utilized runoff from climate models output to predict changes in future river discharge (e.g., Hirabayashi et al., 2021). The runoff can also be estimated by land surface models (LSMs) using meteorological outputs from climate models.
Nevertheless, estimated streamflow from climate models could be uncertain because of, for example, biases in the meteorological data and parameterization and structures of LSMs. Understanding the reasons for uncertainties in the model will aid in improving streamflow estimation. Past studies suggest that evapotranspiration (e.g., Haddeland et al., 2011), and snow accumulation and melt schemes (Beck et al., 2017) in the LSMs are major uncertain factors in simulated streamflow. However, the impacts of runoff schemes in LSMs as a source of uncertainties are still not well studied. This study aimed to explore to what extent runoff process representations in LSMs impact streamflow estimation.
2.1 Regional climate model output datasets
This study used the output of a non-hydrostatic regional climate model (NHRCM; Sasaki et al., 2008) with enhanced spatial resolutions of 5 and 2 km (RCM05 and RCM02, respectively) simulated for Thailand. RCM05 covered all of Thailand, and RCM02 included only the northern region, as shown in Fig. 1a. The 5 km model was nested in the general circulation model MRI-AGCM 3.2S (Mizuta et al., 2012), and the 2 km model was nested in the 5 km model. The output of the historical climate simulation from 1990 to 1999 was employed here.
2.2 Study area
The target area of this study is the upper part of the Ping River Basin in northern Thailand, as shown in Fig. 1b. It has a total catchment area of approximately 26 100 km2. The main dam in this basin is the Bhumibol Dam. The Ping River is a major tributary of the Chao Phraya River, Thailand's most important river basin.
2.3 Land surface models
Here, the outputs of two LSMs, the Meteorological Research Institute-Simple Biosphere model (MRI-SiB, Hirai et al., 2007) and the Simple Biosphere model including Urban Canopy (SiBUC; Tanaka et al., 2005) were analyzed. MRI-SiB was incorporated into the NHRCM. Both LSMs, which were developed based on the Simple Biosphere model, have common structures, including the discretization of soil into three layers and the use of the Richards equation to estimate soil moisture. However, their details vary, such as, SiBUC estimates subsurface runoff solely on gravitational flow, whereas MRI-SiB also incorporates hydraulic diffusion, and MRI-SiB has a direct infiltration structure from the ground surface to the second soil layer (Tinumbang et al., 2023).
2.4 Flow routing model
This study used a flow routing model 1K-FRM, developed based on the 1-D kinematic wave theory, to convert total runoff (surface and subsurface runoff) into river discharge. A coupled SiBUC and 1K-FRM (Yorozu and Tachikawa, 2015) was employed here. The 1K-FRM was also used to convert the MRI-SiB runoff into river discharge.
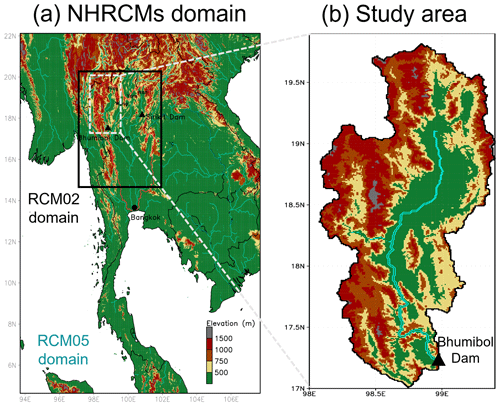
Figure 1(a) Domain of NHRCM 5 and 2 km and (b) study area in the upper part of Ping River Basin, Thailand.
2.5 Observation data
The observed rainfall from the Climate Hazards Group InfraRed Precipitation with Station data (CHIRPS; Funk et al., 2015) was used here. The observed daily inflow at the Bhumibol Dam obtained from the Electricity Generating Authority of Thailand was also employed.
2.6 Research framework
This research is divided into three sections: evaluation of RCMs rainfall, comparison of the water budget estimated by the two LSMs driven by the RCMs output, and evaluation of the streamflow estimated by LSMs runoff. The water budget estimated by MRI-SiB was obtained from the RCMs output dataset. While the water budget by SiBUC was simulated here using precipitation, air temperature, specific humidity, downward short-wave and long-wave radiation, air pressure, and wind speed data from the RCMs output. The analysis of the water budget and streamflow driven by the RCM05 output was published in our previous study (Tinumbang et al., 2019).
3.1 Rainfall evaluation
A comparison of the ten-year-mean monthly rainfall from observation and the RCMs is shown in Fig. 2. Overall, the RCMs rainfall could reproduce the seasonal cycle of observation in this basin well, with most rainfall occurring during the rainy season (May–October) and very low rainfall during the dry season (November–April). However, their amount was underestimated. The annual mean of observed rainfall in this basin is more than 1100 mm. In contrast, the rainfall from the RCM05 and RCM02 was 663 and 638 mm, respectively, as shown in Table 1.
3.2 Water budget comparison
A comparison of the water budgets estimated by the LSMs driven by the outputs of RCM05 and RCM02 is shown in Table 1. MRI-SiB estimated higher evapotranspiration and subsequently, lower runoff, than SiBUC. The MRI-SiB runoff driven by the RCM02 output was lower than that generated by RCM05. This was likely because RCM02 had less rainfall. The SiBUC runoff, however, exhibited the opposite trend. This could be due to the differences in runoff characteristics between the two LSMs, as shown in Fig. 3.
Most of the MRI-SiB runoff was generated as subsurface runoff, whereas the SiBUC runoff was dominated by surface runoff. These variations in runoff characteristics suggest a disparity in runoff schemes, particularly in infiltration capacity between the two LSMs. Because MRI-SiB generated more subsurface runoff than SiBUC, it seemed to have a higher infiltration capacity. As more rainwater infiltrates the soil, more rainwater evaporates, contributing to higher evapotranspiration from MRI-SiB than from SiBUC.
To determine why the SiBUC runoff driven by RCM02 was higher than that driven by RCM05, the spatial patterns of rainfall and runoff were analyzed in detail. Results from 1992 were selected for the analysis. This year, RCM05 and RCM02 had similar basin-average rainfall values of 597 and 581 mm, respectively. However, as shown in Fig. 4a, their spatial distribution varied significantly. The RCM05 rainfall which was close to the basin-average value, shown in orange color, spread throughout the basin. In contrast, the RCM02 rainfall varied significantly between the western and eastern regions. RCM02 rainfall was significantly higher in the western area, which is the high-elevation zone, than in the eastern area, corresponding to the low-elevation zone.
This difference in rainfall appears to have a more significant impact on the SiBUC runoff than that on the MRI-SiB runoff, as shown in Fig. 4b and c. The SiBUC runoff driven by RCM05 and RCM02 was 52 and 86 mm, respectively, whereas the MRI-SiB runoff was 25 and 18 mm, respectively. The grids showing high value by the SiBUC runoff correspond to high rainfall. In contrast, the grids showing high MRI-SiB runoff, such as in the northern region, closely matched the high saturated hydraulic conductivity, as shown in Fig. 5. Since subsurface runoff predominates in MRI-SiB, greater values of saturated hydraulic conductivity indicate a higher infiltration rate and increased runoff.
3.3 Streamflow evaluation
Figure 6 compares the mean daily streamflow between the observation and simulations. Overall, all simulated discharges were underestimated owing to the underpredicted RCMs rainfall. The estimated discharge by MRI-SiB and SiBUC runoff driven by RCM05 was 74 % and 57 % less than the observation, and that driven by RCM02 was 75 % and 25 % lower.
The streamflow simulated by the MRI-SiB runoff using RCM02 was lower than that driven by RCM05 because the RCM02 rainfall was smaller. However, the streamflow simulated by the SiBUC runoff showed the opposite response because the SiBUC runoff driven by RCM02 was higher than that driven by RCM05. This is because more surface runoff was generated owing to more heavy rainfall in RCM02, as discussed in the previous subsection. The discharge from SiBUC runoff was nearly identical to the observed flow, although the RCM02 rainfall was less than the observation. This suggests that the amount of surface runoff that dominates SiBUC runoff may have been overestimated.
In this study, the uncertainty in the simulated streamflow using LSMs runoff driven by NHRCMs output was explored. The NHRCMs rainfall could reproduce the seasonal changes in observed rainfall in the upper part of the Ping River Basin although they were underestimated.
Both NHRCMs had similar total rainfall, though their spatial patterns differed, with the 2 km model being better at capturing the local topography than the 5 km model.
The uncertainty in simulated streamflow was found to be affected by this difference in rainfall patterns and the disparity in runoff characteristics among LSMs. Both simulated streamflow by MRI-SiB runoff, dominated by subsurface runoff, underestimated the observed discharge. In contrast, the estimated streamflow by SiBUC runoff, primarily from surface runoff, was underestimated when it was driven by RCM05, while the one driven by RCM02 was close to the observed discharge. This suggests SiBUC seemed to produce too much runoff because RCM02 rainfall underestimated the observation.
This analysis revealed that the runoff scheme in LSMs could be a major source of uncertainties in streamflow simulation, unlike previous studies which suggested evapotranspiration and snow representations were the primary uncertain factors. It is recommended that the accuracy of rainfall from the climate model and the runoff from the LSMs should be enhanced to improve the reliability of assessing the potential impacts of climate change on water-related disasters.
Derived data supporting the findings of this study are available on request from the corresponding author.
AFAT and KY contributed to conceptualization and methodology; AFAT performed the investigation and wrote the original draft, supervised by KY, YT, YI, and TN; HS and TN provided the NHRCM datasets and MRI-SiB parameters.
The contact author has declared that none of the authors has any competing interests.
Publisher’s note: Copernicus Publications remains neutral with regard to jurisdictional claims made in the text, published maps, institutional affiliations, or any other geographical representation in this paper. While Copernicus Publications makes every effort to include appropriate place names, the final responsibility lies with the authors.
This article is part of the special issue “ICFM9 – River Basin Disaster Resilience and Sustainability by All”. It is a result of The 9th International Conference on Flood Management, Tsukuba, Japan, 18–22 February 2023.
This research has been supported by the Ministry of Education, Culture, Sports, Science and Technology (MEXT) Program for the Advanced Studies of Climate Change Projection (grant no. JPMXD022680734) and Integrated Research Program for Advancing Climate Models Theme C and D (grant nos. JPMXD0717935561 and JPMXD0717935498), and the Japan Science and Technology “Moonshot R&D” (grant no. JPMJMS2283).
This paper was edited by Mohamed Rasmy and reviewed by two anonymous referees.
Beck, H. E., van Dijk, A. I. J. M., de Roo, A., Dutra, E., Fink, G., Orth, R., and Schellekens, J.: Global evaluation of runoff from 10 state-of-the-art hydrological models, Hydrol. Earth Syst. Sci., 21, 2881–2903, https://doi.org/10.5194/hess-21-2881-2017, 2017.
Funk, C., Peterson, P., Landsfeld, M., Pedreros, D., Verdin, J., Shukla, S., Husak, G., Rowland, J., Harrison, L., Hoell, A., and Michaelsen, J.: The climate hazards infrared precipitation with stations-a new environmental record for monitoring extremes, Sci. Data, 2, 1–21, https://doi.org/10.1038/sdata.2015.66, 2015.
Haddeland, I., Clark, D. B., Franssen, W., Ludwig, F., Voß, F., Arnell, N. W., Bertrand, N., Best, M., Folwell, S., Gerten, D., Gomes, S., Gosling, S. N., Hagemann, S., Hanasaki, N., Harding, R., Heinke, J., Kabat, P., Koirala, S., Oki, T., Polcher, J., Stacke, T., Viterbo, P., Weedon, G. P., and Yeh, P.: Multimodel estimate of the global terrestrial water balance: setup and first results. J. Hydrometeor., 12, 869–884, https://doi.org/10.1175/2011JHM1324.1, 2011.
Hirabayashi, Y., Tanoue, M., Sasaki, Xudong, Z., and Yamazaki, D.: Global exposure to flooding from the new CMIP6 climate model projections, Sci. Rep., 11, 3740, https://doi.org/10.1038/s41598-021-83279-w, 2021.
Hirai, M., Sakashita, T., Kitagawa, H., Tsuyuki, T., Hosaka, M., and Oh'izumi, M.: Development and Validation of a New Land Surface Model for JMA's Operational Global Model Using the CEOP Observation Dataset, J. Meteor. Soc. Jpn., 85A, 1–24, https://doi.org/10.2151/jmsj.85A.1, 2007.
IPCC, Intergovernmental Panel on Climate Change Climate Change: The Physical Science Basis. Contribution of Working Group I to the Sixth Assessment Report of the Intergovernmental Panel on Climate Change, Cambridge University Press, https://doi.org/10.1017/9781009157896, 2021.
Sasaki, H., Murata, A., Hanafusa, M., Oh'izumi, M., and Kurihara, K.: Reproducibility of present climate in a non-hydrostatic regional climate model nested within an atmosphere general circulation model, SOLA, 7, 173–176, https://doi.org/10.2151/sola.2011-044, 2011.
Mizuta, R., Yoshimura, H., Murakami, H., Matsueda, M., Endo, H., Ose, T., Kamiguchi, K., Hosaka, M., Sugi, M., Yukimoto, S., Kusunoki, S., and Kitoh, A.: Climate simulations using MRI-AGCM with 20 km grid, J. Meteor. Soc. Jpn., 90A, 235–260, https://doi.org/10.2151/jmsj.2012-A12, 2012.
Tanaka, K.: Development of the new land surface scheme SiBUC commonly applicable to basin water management and numerical weather prediction model, doctoral dissertation, Kyoto University, http://hdl.handle.net/2433/145376 (last access: 5 March 2024), 2005.
Tinumbang, A. F. A, Yorozu, K., Tachikawa, Y., Ichikawa, Y., Sasaki, H., and Nakaegawa, T.: Analysis of runoff characteristics generated by land surface models and their impacts on river discharge, J. Jpn. Soc. Civ. Eng, 75, I_271–I_276, https://doi.org/10.2208/jscejhe.75.2_I_271, 2019.
Tinumbang, A. F. A., Yorozu, K., Tachikawa, Y., and Ichikawa, Y.: Developing a methodology for model intercomparison and its application to improve simulated streamflow by land surface models, J. Hydrometeor., 24, 817–833, https://doi.org/10.1175/JHM-D-22-0183.1, 2023.
Yorozu, K. and Tachikawa, Y.: The effect on river discharge estimation by considering an interaction between land surface process and river routing process, Proc. Int. Assoc. Hydrol. Sci, 369, 81–86, https://doi.org/10.5194/piahs-369-81-2015, 2015.