the Creative Commons Attribution 4.0 License.
the Creative Commons Attribution 4.0 License.
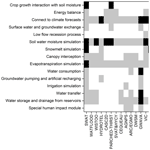
A review of the adaptability of hydrological models for drought forecasting
Zikang Xing
Miaomiao Ma
Zhicheng Su
Juan Lv
Peng Yi
Wenlong Song
Drought intensity and frequency are increasing in recent years in multiple regions across the world due to global climate change and consequently drought forecasting research has received more and more attention. Previous studies on drought forecasting mostly focus on meteorological drought based on precipitation and temperature. However, the trend of predicting agriculture and hydrological drought, which consider soil moisture and runoff, have developed rapidly in recent years. Hydrological drought forecasting is based on the hydrological models and the model structure plays a role to improve predictions. This study scrutinized more than 50 hydrological models, including lumped models, semi-distributed models, distributed models, surface water and groundwater coupled models, to explore the adaptability of hydrological models in drought simulation and forecasting. The advantages and disadvantages of typical models, such as DTVGM, GWAVA, and HEC-HMS models were analyzed to provide valuable reference for drought forecasting model development. Future work aims at improving the hydrological models to simulate the drought processes and make better prediction.
- Article
(1033 KB) - Full-text XML
- BibTeX
- EndNote
Drought intensity and frequency are increasing in past and recent years in multiple across the globe due to global climate change (Wilhite and Glantz, 1985). In this context, methods to predict drought has received more and more attention (Edwards and McKee, 1997). Previous study of drought forecasting mostly focused on meteorological drought based on precipitation and temperature, while the trend prediction of agriculture and hydrological drought, which consider soil moisture and runoff, have developed rapidly in recent years (Wilhite, 2000; Wanders et al., 2019). There are two main types of methods involved in the hydrological drought forecasting. One is the statistical method that tries to develop construct the relationship between hydrological characteristics and drought events, such as the gray forecasting method (Vishnu and Syamala, 2012), Markov chain method (Paulo and Pereira, 2007), Error back Propagation neural network (Raju et al., 2011), and correlation analysis. The second method is the methods based on hydrological models and coupled atmospheric-hydrological models (Mishra and Singh, 2011). For the latter method, the model structure plays a significant role to improve predictions.
Most hydrological models have been developed for flood simulation and prediction (Huggins and Monke, 1970). Generally, flood generation tends to focus on fast processes and peaks can form in a few days or even hours, while the process of drought development is much slower covering several months or years. Therefore, flood forecasting is a short-term high flow prediction, but the drought forecasting focuses on medium and long-term low flow prediction (Mishra and Singh, 2010). Most hydrological models could improve on drought prediction in humid watersheds as they put emphasis on peak flow simulation rather than low flow (Yu et al., 1999). If these models are used for the drought forecasting, they might suffer from the limitations of structure. Two scientific questions are addressed: what are the limitations of existing hydrological models when used for the drought forecasting, and how to improve the structure in order to make the drought prediction better? In this study, we scrutinized more than 50 hydrological models, including lumped models, semi-distributed models, distributed models, integrated surface water and groundwater models, to explore the adaptability of hydrological models in drought simulation and forecasting. Afterwards, improvements of the model structure are proposed.
2.1 Model selection and classification
More than 50 hydrological models were involved in this study. The main source was from the model review paper by Singh and Woolhiser (2002), some seldom used models were abandoned, and other recent representative hydrological models were also included (20 models). The baseline of model selection is that the model has been widely used and is easy to get the manual. According to the evolution of hydrological models, these selected models were classified into four types: lumped model, semi-distributed model, distributed model and integrated surface water and groundwater model. The selected models include: 13 lumped models (Fig. 1), 6 semi-distributed models (Fig. 2), 29 distributed models (Fig. 3) and 10 integrated surface water and groundwater models (Fig. 4).
2.2 Evaluation method for assessment of the adaptability of hydrological models for drought forecasting
2.2.1 Evaluation criteria
Considering the differences between flood and drought forecasting, their corresponding model structure need to be distinct. For drought forecasting, emphasis on low flows, therefore, the simulation of evapotranspiration, soil water moisture and the recession process need to be well developed. Snow melting, vegetation interception, groundwater and surface water exchange cannot be neglect any more. The influences of crops and soil water dynamics should be considered in the low flow simulation and prediction. In addition, human impacts, including water storage and drainage from reservoirs, water transfer, agricultural irrigation, groundwater pumping or artificial recharging, water consumption, also need to be included in drought forecasting, because they have significant effect on drought in some areas (Van Loon et al., 2016). Given that the drought forecasting needs longer lead times for prediction, hydrological models should be linked directly to the climate forecasting results in order to extend the lead times. Since drought forecasting deals with low flow, the energy balance should be considered to a certain extent to supplement the water balance calculation. To sum up, 15 evaluation criteria (Table 1) were used to explore the adaptability of hydrological models for drought forecasting in this study.
2.2.2 Evaluation method
A rank evaluation method was used in this study. Three grades were distinguished: 0, 1 and 2. “0” stands for no consideration of the function or module; “1” stands for simple calculation of the function or module; “2” stands for mature or powerful calculation of the function or module.
3.1 Lumped model evaluation
The adaptability of lumped hydrological models for drought forecasting is shown in Fig. 1. Most of selected lumped models simulate evaporation calculation except CLS model, whereas the XAJ and VM show the powerful function with a three layers evaporation calculation method. 70 % of the selected models simulate soil water moisture with simple equations, while the Sacramento model divides the soil into different layers and calculate soil moisture in each layer. Five models include simulation of canopy interception and snowmelt. Three models (i.e. EPIC, ARM and SVAT) consider the energy balance together with the water balance. Only the EPIC model has irrigation module, while the EPIC and ARM consider the influences of crop growth.
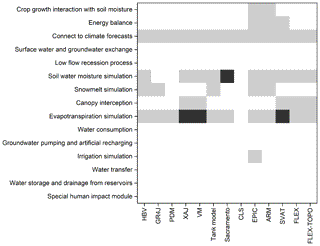
Figure 1Evaluation of the adaptability of lumped hydrological models for drought forecasting. Where white color stands for grade 0 (no consideration of the function or module); gray color stands for grade 1 (simple simulation of the function or module); black color stands for grade 2 (powerful simulation of the function or module).
3.2 Semi-distributed model evaluation
The evaluation results of six selected semi-distributed models are shown in Fig. 2. Results exhibit that all the selected semi-distributed models simulate evaporation and soil water moisture, whereas the LASCAM and ARNO appear powerful soil water moisture simulation. The ARNO model involves the spatial probability distribution of soil moisture to dynamically change the saturation contribution area. The SWRRB and SLURP consider the effects of human activities, such as water storage and drainage from reservoirs. In particular, the SLURP model includes simulation of several human activities, including water transfers, irrigation and groundwater pumping and artificial recharging. The ARNO model simulates water consumption, while the Top model takes the exchange of groundwater and surface water into consideration. All semi-distributed models only simulate water balance without taking the energy balance into account. None of the selected models consider low flow recession and crop growth influences on soil water moisture and evapotranspiration.
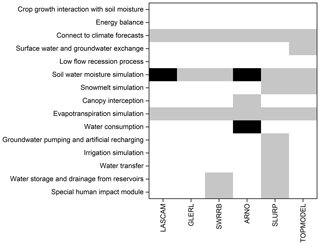
Figure 2Evaluation of the adaptability of semi-distributed hydrological models for drought forecasting. Where white color stands for grade 0 (no consideration of the function or module); gray color stands for grade 1 (simple simulation of the function or module); black color stands for grade 2 (powerful simulation of the function or module).
3.3 Distributed model evaluation
The evaluation results of the 29 selected distributed models are shown in Fig. 3. All the models simulate evapotranspiration and soil water moisture, where SWAT, WISTOO, CASC2D, PARCHED-THIRST, VIC and PRMS approach the soil water moisture simulation in different ways. SWAT, GWAVA, MIKE-SHE and DTVGM consider most of the human activities that impact the hydrological system. The four human activity modules we put forward, are ranked as follows: water storage and drainage from reservoirs, water transfer, irrigation, groundwater pumping and artificial recharging, water consumption. The impact of the reservoirs is most frequently addressed by model developers. Nearly half of the selected models consider canopy interception and snowmelt modules, which often can be explained for which catchment the model originally has been developed. Half of selected models consider groundwater and surface water exchange, among which SWIM, SHETRAN and HEC-HMS containing specialized groundwater simulation modules. Five models consider the simulation of low flow conditions, while night models (i.e. HYDROTEL, CREST, HSPF, GWAVA, VIC, Wasim-ETH, MIKE-SHE, DHSVM and GBHM) can be connected to weather forecasts well, because of their grid-based structure. Four models (i.e. SWAT, PARCHED-THIRST, SWIM, and Mike-SHE) investigate crop growth influences on the hydrological system. Compared with lumped and semi-distributed models, many distributed models start to consider the energy balance to supplement the water balance simulation.
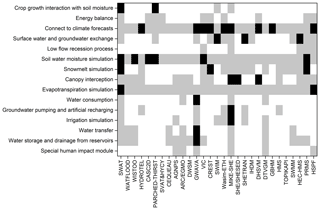
Figure 3Evaluation of the adaptability of distributed hydrological models for drought forecasting. Where white color stands for grade 0 (no consideration of the function or module); gray color stands for grade 1 (simple simulation of the function or module); black color stands for grade 2 (powerful simulation of the function or module).
3.4 Integrated surface water and groundwater model evaluation
The evaluation results of 10 selected integrated surface water and groundwater models are shown in Fig. 4. All the model studied consider evaporation, canopy interception, soil water moisture and the exchange between surface water and groundwater. The ability to simulate groundwater flow and groundwater pumping and artificial recharging makes this type of models more powerful than the other types of models. MODHMS, HydroGeoSphere and Parflow are fully coupled models of groundwater and surface water. They use partial differential equations to describe surface water and groundwater flow processes. SWATMOD, and MODHMS perform well in simulating human activities. The Parflow model is a hydrological model for parallel computing that takes the energy balance into account and can be efficiently linked to climate models. The SWATMOD as the coupled model between SWAT and Modflow consider the influence of crop growth on the hydrological system.
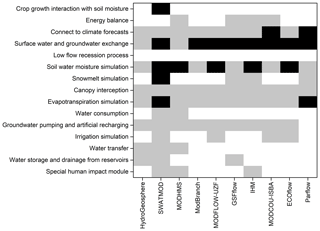
Figure 4Evaluation of the adaptability of integrated surface water and groundwater models for drought forecasting. Where white color stands for grade 0 (no consideration of the function or module); gray color stands for grade 1 (simple simulation of the function or module); black color stands for grade 2 (powerful simulation of the function or module).
The model structure of lumped hydrological model seems a bit simpler compared with the other types of models, and they seldom consider human impact (Bergstrom, 1995). While distributed models investigate more human impact in the hydrological cycle, such as the water storage and drainage from reservoirs, water transferring, water consumption, irrigation. The integrated surface water and groundwater model couple the advantages of surface water and groundwater simulations. They consider the exchange between surface water and groundwater, and enable to simulate the groundwater pumping and artificial recharging. However, they are too sophisticated to be used for drought forecasting, because they need too many data to run (Beven and Binley, 1992). In summary, distributed models are more suitable for drought forecasting compared with lumped and semi-distributed models. Results indicate that SWAT, GWAVA, MIKE-SHE and DTVGM are the most powerful in the category of the distributed models because they simulate the human impact on the hydrological system, and they solve the energy balance together with water balance.
The MIKE-SHE model covers ranges of physical processes with high requirements on parameters and data, and hence its operation seems more sophisticated than the other three above-mentioned distributed models (Refsgaard and Storm, 1995). The SWAT model is an open source model and it is updated by world researchers, which have developed several modules for human impacts and crop growth. The SWAT model has been widely used in non-point source pollution simulation (Jayakrishnan et al., 2005), but seldom has been used for drought forecasting. When applied to drought forecasting, it is better to improve the low flow simulations and the connection to climate forecasting. The advantage of GWAVA model is the human impact module that can simulate water demand, water transfer, agricultural irrigation and population distribution as a driver for human activities. However, the simulation modules for human impacts seems a bit simple. In contrast, the DTVGM model was developed based on the characteristics of Chinese Yellow River basin, with emphasis on water conservation, water consumption, reservoirs and low flow simulations (Ning et al., 2016). The highlight of HEC-HMS is reflected in the flexibility of its model structure, which can be adapted to the catchments for different natural conditions (Hydrologic Engineering Center, 2000). To sum up, combination of the model structures of SWAT, GWAVA and DTVGM might be a good solution for drought forecasting, using the flexible structure of the HEC-HMS model.
This study scrutinized more than 50 hydrological models, including lumped models, semi-distributed models, distributed models, surface water and groundwater coupled models, to explore the adaptability of hydrological models for drought simulation and forecasting. In this paper, we discuss the limitations of the wide range of selected hydrological models to be used for the drought forecasting and to propose improvement of model structures to make better fit for drought prediction. Results indicate that distributed models are more suitable for drought forecasting compared with the other types of models. A combination of SWAT, GWAVA and DTVGM might be a good solution for drought forecasting using the flexible structure of the HEC-HMS model.
All relevant data are within the paper.
PY and WS designed the research; ZS and JL collected the data; ZX and MM analyzed the data and wrote the paper.
The authors declare that they have no conflict of interest.
This article is part of the special issue “Hydrological processes and water security in a changing world”. It is a result of the 8th Global FRIEND–Water Conference: Hydrological Processes and Water Security in a Changing World, Beijing, China, 6–9 November 2018.
This research has been supported by the IWHR Research & Development Support Program (grant no. JZ0145B582017), the the National Natural Science Foundation of China (grant no. 51609257), the the Hydraulic science and technology in Hunan Province (grant no. [2017]230-36), and the the National Key R&D Program of China (grant no. 2017YFC1502406).
Bergstrom, S.: Chapter 13: The HBV model, Computer models of watershed hydrology, edited by: Singh, V. P., Water Resources Publications, Littleton, Colorado, USA, 1995.
Beven, K. J. and Binley, A. M.: The future of distributed models: model calibration and predictive uncertainty, Hydrol. Process., 6, 279–298, 1992.
Edwards, D. C. and McKee, T. B.: Characteristics of 20th century drought in the United States at multiple time scales, in: Climatology Report 97-2; Department of Atmospheric Science, Colorado State University, Fort Collins, CO, USA, 1997.
Huggins, L. F. and Monke, E. J.: Mathematical simulation of hydrologic events of ungaged watersheds, Water Resources Research Center, Tech. Rep. No. 14, Purdue Univ., West Lafayette, Ind., USA, 1970.
Hydrologic Engineering Center (HEC): Hydrologic modeling system HEC-HMS user's manual, version 2, Engineering, U.S. Army Corps of Engineers, Davis, Calif., USA, 2000.
Jayakrishnan, R., Srinivasan, R., Santhi, C., and Arnold, J. G.: Advances in the application of the SWAT model for water resources management, Hydrol. Process., 19, 749–762, 2005.
Mishra, A. K. and Singh, V. P.: A review of drought concepts, J. Hydrol., 391, 202–216, 2010.
Mishra, A. K. and Singh, V. P.: Drought modeling – A review, J. Hydrol., 403, 157–175, 2011.
Ning, L., Xia, J., Zhan, C., and Zhang, Y.: Runoff of arid and semi-arid regions simulated and projected by CLM-DTVGM and its multi-scale fluctuations as revealed by EEMD analysis, J. Arid Land, 8, 506–520, 2016.
Paulo, A. A. and Pereira, L. S.: Prediction of SPI Drought Class Transitions Using Markov Chains, Water Resour. Manag., 21, 1813–1827, 2007.
Raju, M. M., Srivastava, R. K., Bisht, D. C. S., Sharma, H. C., and Kumar, A.: Development of artificial neural-network-based models for the simulation of spring discharge, Adv. Art. Int., 2011, 1–11, https://doi.org/10.1155/2011/686258, 2011.
Refsgaard, J. C. and Storm, B.: Chapter 23: MIKE SHE, Computer models of watershed hydrology, edited by: Singh, V. P., Water Resources Publications, Littleton, Colorado, USA, 1995.
Singh, V. P. and Woolhiser, D. A.: Mathematical Modeling of Watershed Hydrology, J. Hydrol. Eng., 7, 270–292, 2002.
Van Loon, A. F., Gleeson, T., Clark, J., Van Dijk, A. I. J. M., Stahl, K., Hannaford, J., Di Baldassarre, G., Teuling, A. J., Tallaksen, L. M., Uijlenhoet, R., Hannah, D. M., Sheffield, J., Svoboda, M., Verbeiren, B., Wagener, T., Rangecroft, S., Wanders, N., and Van Lanen, H. A. J.: Drought in the Anthropocene, Nat. Geosci., 9, 89–91, https://doi.org/10.1038/ngeo2646, 2016.
Vishnu, B. and Syamala, P.: Grey Model for Stream Flow Prediction, Aceh International Journal of Science and Technology, 1, 14–19, available at: https://doaj.org/article/806ab2d8b3134e98a512ddf3409ca26f (last access: 5 August 2020), 2012.
Wanders, N., Thober, S., Kumar, R., Pan, M., Sheffield, J., Samaniego, L., and Wood, E. F.: Development and evaluation of a Pan-European multimodel seasonal hydrological forecasting system, J. Hydrometeorol., 20, 99–114, https://doi.org/10.1175/JHM-D-18-0040.1, 2019.
Wilhite, D. A. (Ed.): Droughts as a natural hazard: concepts and definitions, in: DROUGHT, A Global Assessment, vol. I, Routledge Hazards and Disasters Series, Routledge, London, UK, 3–18, 2000.
Wilhite, D. A. and Glantz, M. H.: Understanding the drought phenomenon: The role of definitions, Water Int., 10, 111–120, 1985.
Yu, Z., Lakhtakia, M. N., Yarnal, B., White, R. A., Miller, D. A., Frakes, B., Barron, E. J., Duffy, C., and Schwartz, F. W.: Simulating the river-basin response to atmospheric forcing by linking a mesoscale meteorological model and a hydrologic model system, J. Hydrol., 218, 72–91, 1999.