the Creative Commons Attribution 4.0 License.
the Creative Commons Attribution 4.0 License.
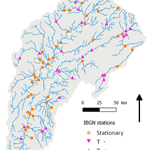
Detecting trend on ecological river status – how to deal with short incomplete bioindicator time series? Methodological and operational issues
Flavie Cernesson
Marie-George Tournoud
Nathalie Lalande
Among the various parameters monitored in river monitoring networks, bioindicators provide very informative data. Analysing time variations in bioindicator data is tricky for water managers because the data sets are often short, irregular, and non-normally distributed. It is then a challenging methodological issue for scientists, as it is in Saône basin (30 000 km2, France) where, between 1998 and 2010, among 812 IBGN (French macroinvertebrate bioindicator) monitoring stations, only 71 time series have got more than 10 data values and were studied here. Combining various analytical tools (three parametric and non-parametric statistical tests plus a graphical analysis), 45 IBGN time series were classified as stationary and 26 as non-stationary (only one of which showing a degradation). Series from sampling stations located within the same hydroecoregion showed similar trends, while river size classes seemed to be non-significant to explain temporal trends. So, from a methodological point of view, combining statistical tests and graphical analysis is a relevant option when striving to improve trend detection. Moreover, it was possible to propose a way to summarise series in order to analyse links between ecological river quality indicators and land use stressors.
- Article
(1818 KB) - Full-text XML
- BibTeX
- EndNote
The improvement or degradation of the ecological status of a river often results from the combined effect of natural environmental conditions and anthropogenic actions. Detecting the effectiveness of national policies or actions taken by local authorities is then a major challenge for policy-makers and water managers (Lalande et al., 2014; Diamantini et al., 2018). Therefore, there is a great need for tools to quantify the evolution of the ecological river status over time what we call here: trend. The trend could evolve positively, negatively, or be stable, as the ecological river status is improved, is degraded or is constant.
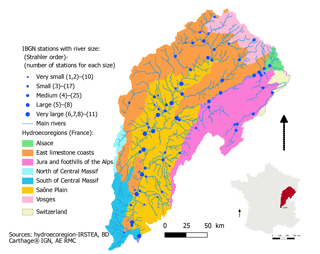
Figure 1Saône basin: hydroecoregions, main rivers and IBGN monitoring stations network. Each station is localised by a blue point and ranked by the river size: the more the point is big, the more the river is large. For each river size, Strahler orders and IBGN stations count are mentioned into the first and second brackets respectively.
Bioindicators are recognized to be good tools to monitor ecological river status, as they indicate both long-term disturbances as well as short-term perturbations that are sufficiently intense to cause the immediate death of the living organisms (Karr and Chu, 2000). Unfortunately these indicators are quite difficult to elaborate because sampling and analysing protocols are often time consuming and expensive. They are often modified by new regulation or new scientific knowledge. Hence, bioindicator series are often short and irregularly sampled data sets: stations are usually sampled at most twice a year but sometimes not at all for several consecutive years. Moreover the data sets may be non-normally distributed. So, it is not easy to analyse such series using statistical techniques (McLeod et al., 1991).
The objective of this paper is to propose a framework to qualify trends in short and irregular bioindicator time series with a focus on the French macroinvertebrate bioindicator time series: IBGN (Indice Biologique Global Normalisé). Series gathered in the Saône basin are studied here. The positive, negative or stable trends are discussed and attempt to link them to the overall characteristics of the stations is presented.
2.1 Study site: Saône basin
The Saône River, located in the East of France, is the main tributary of the Rhône River. It has a catchment area of 30 000 km2 and a river network of 9000 km. With a total of 2.6 million inhabitants, the basin is relatively sparsely urbanized: urban areas represent 5 % of the basin. Industrial activities are located near the urban areas. Semi-natural forest areas cover about 35 % of the basin. About 30 % of the catchment is covered with crops and another 30 % with grasslands: livestock dominates in the upper basin, the left bank and the lower valley are mainly devoted to grain farming and market gardening, while the right bank is occupied by vineyards.
2.2 Ecological river quality data: IBGN data set
The IBGN macroinvertebrate index is based on the abundance of river benthic invertebrates and their selective sensitivity to environmental stressors (flow, substrate, dissolved substances, temperature, light, pH, turbidity, etc.). The IBGN index is mainly used to monitor organic pollution, but it could also indicate the presence of chemical or toxic substances, or local habitat deteriorations. In France it is the most commonly used standard index since 1985 (AFNOR, NF T90-350) and is therefore one of the most studied and most abundant in terms of available data. IBGN scores range from 1 (very bad status) to 20 (very good status) as the combination of two notes related to: (i) the taxon richness which is based on the total number of taxa families harvested during sampling; (ii) the observed benthic macroinvertebrate group which is the most pollution-sensitive.
Among the 812 stations monitored by the French national Rhone-Mediterranean and Corsica water agency in the Saône River basin, 71 IBGN data series that contained at least 10 values between 1988 and 2010 are studied here. These data series represent all kinds of rivers even if they are mainly located in the Saône main watercourse and in the Doubs River (in the mid-eastern part of the watershed) as shown Fig. 1.
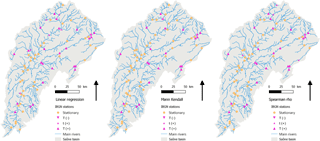
Figure 2Detected trends for the 71 IBGN series (statistical tests and FDR correction). Stationary, t(+), t(−) stands for: stable, positive and negative trend respectively. The use of the capital letter “T” instead of the “t” means the test results remains significant after the FDR correction.
Two parameters are used to characterize the monitoring stations: the hydroecoregion and the river size. The Saône basin consists of seven hydroecoregions (Fig. 1). Three main hydroecoregions are represented by 64 stations: “Saône plain”, “East limestone coasts” and “Jura and foothills of the Alps” respectively include 28, 21 and 15 stations. “Vosges”, “Alsace” and the “South of the Central Massif” are respectively represented by 3, 2 and 2 stations. Finally there is no station in the “North of the Central Massif” region. The river size is divided into five classes based on Strahler order (classes can include two or three Strahler orders). The stations are mainly monitoring medium and small size rivers, with respectively 25 and 17 stations (Fig. 1).
2.3 Method
Statistical tests are powerful tools to evaluate if a time- serie is stationary or not. For small and incomplete data sets, three statistical tests are particularly well-suited and commonly used: a parametric test – the linear regression (LR) – and two non-parametric tests – the Mann–Kendall (MK) and Spearman rho (SR) tests (Hirsch et al., 1991; Diamantini et al., 2018). In this study, the outputs of these three tests were analysed and compared simultaneously. So, an adjustment for multiple testing that reduces the risk of wrongly detecting significant effects was necessary. Among the existing methods, the False Discovery Rate adjustment (FDR), which is considered as one of the most robust was chosen here (Bar-Hen et al., 2005). A corrected p value was calculated for each test as follows: corrected_p = min, where p is the initial p value, nbp is the total number of p values calculated (here, nbp = 3) and j is the rank of the p value when the p values are ranked in ascending order. After this correction, the series were classified as stationary series (respectively non-stationary), if the three tests do not reject (respectively reject) the null hypothesis (i.e. if the three corrected_p were lower (respectively greater) to 0.05). If the three tests did not produce the same conclusion, a plot analysis was conducted to visually identify if the main characteristics (i.e. shape of plots) of the series are (or not) responsible for these discrepancies. Indeed, when the series are too short, statistical tests are sometimes inefficient while graphical methods offer a non-formal way of detecting trends in series. Unlike McLeod et al. (1991), we did not use the graphical method as explanatory tool but as an additional one, to enhance the reliability of the statistical analysis. To assist in reading the time plots we added a locally weighted scatterplot smoothing (LOESS) which is a robust regression smoothing, as the smoothed points are not distorted by extreme values or other kinds of deviant points.
The detection of trend in the IBGN time-series was then linked to the environmental conditions and characteristics of the monitoring stations. A Chi test was carried out (using a permutation procedure to consider small sample size) to study the effect of (i) the size of the river at the station, based on the Strahler order and (ii) the environmental context defined by the hydroecoregion. Indeed, Strahler river order is one of the most important factors influencing the structure and the function of river (Crunkilton and Duchrow, 1991; Hughes et al., 2011). Moreover, rivers located in the same hydroecoregion share the same natural background defined by geological, climate and relief parameters. These parameters are important factors that significantly influence river functioning and river fauna and flora composition (Cohen et al., 1998).
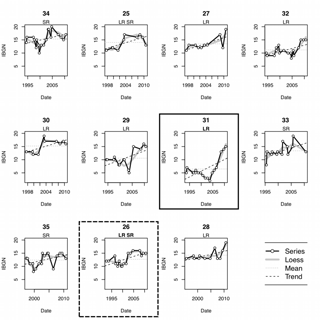
Figure 3Plots of the eleven series for which the three tests, taken individually, gave different outputs. The two box- plots refer to unclassified series after the FDR correction. Black line with white dots are the observed IBGN values, light dotted line is the mean of the series, bold dashed line is the linear regression, bold light grey line is the LOESS fitting of the series.
3.1 Trends in IBGN data set
The outputs of the three tests are showed in Fig. 2. Respectively 32, 29, 24 IBGN series were qualified as non-stationary by LR, SR, MK statistical tests.
The three tests gave the same results for 60 series: 24 series were classified as non-stationary (23 with a positive trend and one with a negative one) and 36 as stationary. Graphical analysis confirmed that the 24 series that were directly classified by the three tests as non-stationary showed a strong temporal evolution of IBGN over time. FDR correction did not modify these results.
The three tests disagreed on 11 series: the 11 series were all qualified as stationary by MK test while 6 of them were stood as non-stationary by LR test, 3 of them by SR test and the remaining 2 by both LR and SR tests. After FDR correction, the three tests MK, LR and SR agreed to qualify 9 out of 11 series as stationary series. There were still 2 unclassified series for which the test results were not modified by FDR correction: series 31 and 26 as shown on Fig. 3. A positive trend was identified in serie 31 by the LR test because IBGN scores increased by 13 points during the last 7 years. The non-stationarity of serie 26 was still significant for the LR and SR tests, because fluctuations were quite small and did not hide the significant positive jump in IBGN scores (plus 4 points) observed since 2003. Series 26 and 31 were then visually reclassified, as non-stationary series, with a positive trend.
The graphical analysis of these 11 cases showed very different shapes (Fig. 3): it indicates that trends in IBGN time series were sometimes not well-defined, due to (i) extremes values at the beginning or at the end of the time serie, (ii) fluctuations between two levels of IBGN values plotted as a two-blocks partition. Some series showed score fluctuations greater than 5 points, which makes very difficult graphical interpretation, and can introduce a statistical bias in tests and distort their results. Moreover outliers that significantly affected the shape of the graph of small series may have the same effect. Most of these outliers were due to the effect of the 2003 drought.
To conclude, out of the 71 series, our methodology classified 26 series as non-stationary (positive trend for 25 out of 26) and 45 as stationary. So, ecological river status was either stable (mainly for the Saône River) or improving (mainly for the Doubs River and the other Saône River tributaries) according to the IBGN index (Fig. 4).
3.2 Trend versus environmental characteristics
The Chi test applied to the data set showed that trends in ecological river status were significantly linked to the natural environmental background of each river defined by the hydroecoregions (χ2=13.48, p=0.01), but were not related to the river size at the station (χ2=9.01, p=0.06). Stationary and non-stationary stations are observed in all classes of river size. For very large river (i.e. Strahler order greater than 6), 10 stations among 11 showed stable trends in their ecological river status and only 1 has a positive trend. For all the other cases, both stable and non-stable trends were observed in equal parts. So, trends in the ecological river status were not really linked to river sizes in the Saône River. This result confirms the opinion issued by Hughes et al. (2011) that summarizing river size only by the Strahler order is an over-simplification of the issue. A significant link between the trend classification and hydroecoregions was found. Rivers located on the same hydroecoregion shared the same natural background. Hydroecoregion classification is a key-factor for river functioning and river fauna and flora composition. However, it is worth to remind that the number of monitoring stations in each hydroecoregion varies from 2 (“South of the Central Massif” region) to 28 (“Saône plain” region). This may have a strong influence on our findings.
Due to the very coarse scale of the two environmental factors we used here, the interpretation and explanation of observed patterns was difficult. More interesting patterns would probably emerge from analyses based on the type of waterbodies that combines both river size and natural background.
The average IBGN score of stationary series (mean 14.25 over 20–45 series) and non-stationary series (12.47 over 20–26 series) was significantly different (F=10.46, p=0.001). This could be explained by a saturation effect: as only one negative trend is observed, and as the IBGN score is limited to 20, positive trends can only be observed in sites where an IBGN increase is still possible (those with a moderate or low initial IBGN value).
Trend in ecological river status is difficult to qualify because the monitoring of bioindicators is not performed on regular basis (sampling started, is interrupted and then restarted, etc.). This leads to small and irregular time series. So a suitable methodology is required, that imposes the use of several analytical tools. It is important to apply a combination of statistical tools (e.g., parametric and not parametric methods) and less conventional ones, such as graphical analysis. The graphical analysis helped to confirm the type of trends and to underline the strengths and weaknesses of the various statistical procedures. The methodological framework proposed here combines three statistical tests (Mann–Kendall, Spearman's rho test, and the linear regression) and an a posteriori graphical visualization assisted by the LOESS procedure. On the example of the Saône River IBGN data, the combined use of different statistical and graphical tools was shown to be a relevant alternative when trying to improve trend detection, which is a key-issue in ecological river status assessment. This combined approach provides a powerful tool to improve the accuracy of the environmental diagnosis of water managers and the significance of the reporting of policy makers.
All the data we used are open data produced by french public agencies or french research institute. Hydroecoregions data are the results of Wasson et al. (2002). and can be downloaded https://www.data.gouv.fr/fr/datasets/hydroecoregions-de-niveau-1-her-1/. The main rivers, extracted from Carthage® IGN data base, can be downloaded https://www.data.gouv.fr/fr/datasets/cours-deau-metropole-2014-bd-carthage/. River size informations can be downloaded https://www.data.gouv.fr/fr/datasets/masses-deau-cours-deau-metropole-version-rapportage-2010/. IBGN monitoring stations informations are produced by the French national Rhone-Mediterranean and Corsica Agency. Localisation of monitoring stations can be downloaded http://carmen.carmencarto.fr/74/sierm.map. IBGN data can be downloaded http://sierm.eaurmc.fr/surveillance/eaux-superficielles/index.php.
The authors declare that they have no conflict of interest.
This article is part of the special issue “Innovative water resources management – understanding and balancing interactions between humankind and nature”. It is a result of the 8th International Water Resources Management Conference of ICWRS, Beijing, China, 13–15 June 2018.
This work was funded by the French National Research Agency (ANR), as part of
the ANR11_MONU14 FresQueau project and by Agence de l'Eau
Rhône-Méditerranée et Corse (agreement no. 2010 0190). The
authors thank the reviewers for their very useful comments.
Edited by: Depeng Zuo
Reviewed by: Feifei Cao and one anonymous referee
Bar-Hen, A., Daudin, J.-J., and Robin, S.: Comparaisons multiples pour les microarrays, Journal de la société française de statistique, 146, 45–62, 2005.
Cohen, P., Andriamahefa, H., and Wasson, J. G.: Towards a regionalization of aquatic habitat: Distribution of mesohabitats at the scale of a large basin, Regul. River., 14, 391–404, 1998.
Crunkilton, R. L. and Duchrow, R. M.: Use of stream order and biological indexes to assess water-quality in the osage and black river basins of Missouri, Hydrobiologia, 224, 155–166, 1991.
Diamantini, E., Lutz, S. R., Mallucci, S., Majone, B., Merz, R., and Bellin, A.: Driver detection of water quality trends in three large European river basins, Sci. Total Environ., 612, 49–62, 2018.
Hirsch, R. M., Alexander, R. B., and Smith, R. A.: Selection of Methods for the Detection and Estimation of Trends in Water-Quality, Water Resour. Res., 27, 803–813, 1991.
Hughes, R. M., Kaufmann, P. R., and Weber, M. H.: National and regional comparisons between Strahler order and stream size, J. N. Am. Benthol. Soc., 30, 103–121, 2011.
Karr, J. R. and Chu, E. W.: Sustaining living rivers, Hydrobiologia, 422, 1–14, 2000.
Lalande, N., Cernesson, F., Decherf, A., and Tournoud, M. G.: Implementing the DPSIR framework to link water quality of rivers to land use: methodological issues and preliminary field test, International Journal of River Basin Management, 12, 201–217, 2014.
McLeod, A. I., Hipel, K. W., and Bodo, B. A.: Trend analysis methodology for water-quality time-series, Environmetrics, 2, 169–200, 1991.
Wasson, G., Chandesris, A., Pella, H., and Blanc, L.: Les hydro-écorégions de France métropolitaine – approche régionale de la typologie des eaux courantes et éléments pour la définition des peuplements de référence d'invertébrés, IRSTEA-ex-Cemagref-MEDDE, 190 pp., 2002.