the Creative Commons Attribution 3.0 License.
the Creative Commons Attribution 3.0 License.
Water quality modelling of an impacted semi-arid catchment using flow data from the WEAP model
Andrew R. Slaughter
Sukhmani K. Mantel
The continuous decline in water quality in many regions is forcing a shift from quantity-based water resources management to a greater emphasis on water quality management. Water quality models can act as invaluable tools as they facilitate a conceptual understanding of processes affecting water quality and can be used to investigate the water quality consequences of management scenarios. In South Africa, the Water Quality Systems Assessment Model (WQSAM) was developed as a management-focussed water quality model that is relatively simple to be able to utilise the small amount of available observed data. Importantly, WQSAM explicitly links to systems (yield) models routinely used in water resources management in South Africa by using their flow output to drive water quality simulations. Although WQSAM has been shown to be able to represent the variability of water quality in South African rivers, its focus on management from a South African perspective limits its use to within southern African regions for which specific systems model setups exist. Facilitating the use of WQSAM within catchments outside of southern Africa and within catchments for which these systems model setups to not exist would require WQSAM to be able to link to a simple-to-use and internationally-applied systems model. One such systems model is the Water Evaluation and Planning (WEAP) model, which incorporates a rainfall-runoff component (natural hydrology), and reservoir storage, return flows and abstractions (systems modelling), but within which water quality modelling facilities are rudimentary. The aims of the current study were therefore to: (1) adapt the WQSAM model to be able to use as input the flow outputs of the WEAP model and; (2) provide an initial assessment of how successful this linkage was by application of the WEAP and WQSAM models to the Buffalo River for historical conditions; a small, semi-arid and impacted catchment in the Eastern Cape of South Africa. The simulations of the two models were compared to the available observed data, with the initial focus within WQSAM on a simulation of instream total dissolved solids (TDS) and nutrient concentrations. The WEAP model was able to adequately simulate flow in the Buffalo River catchment, with consideration of human inputs and outputs. WQSAM was adapted to successfully take as input the flow output of the WEAP model, and the simulations of nutrients by WQSAM provided a good representation of the variability of observed nutrient concentrations in the catchment. This study showed that the WQSAM model is able to accept flow inputs from the WEAP model, and that this approach is able to provide satisfactory estimates of both flow and water quality for a small, semi-arid and impacted catchment. It is hoped that this research will encourage the application of WQSAM to an increased number of catchments within southern Africa and beyond.
- Article
(741 KB) - Full-text XML
- BibTeX
- EndNote
At a global level, there is an increasing trend of water quality deterioration (Verhoeven et al., 2006). South Africa, which is typical of developing countries with a semi-arid environment, suffers from water quality pollution resulting from poor infrastructure maintenance and management (Adler et al., 2007), the main manifestations of which are inputs of unacceptably high loads of nutrients into rivers from Waste Water Treatment Plants (WWTPs), leading to eutrophic conditions. Besides eutrophication, major water quality problems experienced by South African rivers include poor microbial water quality (Britz and Sigge, 2012) and acid mine drainage (Simate and Ndlovu, 2014).
South Africa has a relatively strong history of water quantity management, with region-specific hydrology and systems models being developed that are routinely used in water resources management. These include the Pitman (1973) and the Agricultural Catchments Research Unit (ACRU) models (Schulze, 1989) (hydrological models) and the Water Resources Yield (WRYM) (Basson et al., 1994) and Water Resources Modelling Platform (WReMP) (Mallory et al., 2011) systems models, modelling natural and human-altered flow respectively. In contrast, water quality modelling tools specific for application to southern African catchments are poorly developed, and in South Africa, this has led to the water quality component of water resources management being loosely connected to the water quantity component. Although some internationally-developed water quality models have been applied to South African surface waters within scientific studies (e.g. Gorgens and de Clercq, 2006; Piesold et al., 2007), the adoption of a routinely-used water quality model within management agencies in South Africa has been hampered by the lack of observed data with which to calibrate these models and insufficient technical expertise to accurately set up these models. This led to the development of the Water Quality Systems Assessment Model (Slaughter et al., 2012, 2015, 2017; Hughes and Slaughter, 2016; Slaughter and Mantel, 2016; Slaughter, 2017; Slaughter and Mantel, 2017a), which specifically links to the routinely-used systems models in South Africa, namely the WRYM and WReMP models, and subscribes to an approach of requisite simplicity (Stirzaker et al., 2010) by only representing processes that explain the majority of variation of observed water quality data.
The approaches used and the validity of the WQSAM model have been validated in previous studies, including the monthly-to-daily flow disaggregation (Slaughter et al., 2015; Hughes and Slaughter, 2016) and the water quality modelling processes (Slaughter et al., 2012, 2017; Slaughter and Mantel, 2016; Slaughter, 2017), which include simulations of salinity as total dissolved solids (TDS), nutrients (nitrates plus nitrites, phosphates and ammonia) and microbial water quality, illustrating that the approach adopted by WQSAM is appropriate for modelling water quality in South Africa under conditions of limited observed data and management capacity.
The limitations to water quality modelling in South Africa, as a semi-arid developing country, are likely common to most countries within southern Africa, and possibly other regions globally. A broader aim should therefore be the facilitation of the application of WQSAM within other semi-arid developing regions. This would further refine and validate the water quality processes used in WQSAM, and also provide a useful water quality management tool in those regions. The plausibility of this development is constrained by WQSAM's dependence on the systems models, the WReMP and the WRYM, as although the approach used by WQSAM of connecting with these models is useful for management from a South African perspective, this approach also limits the use of WQSAM to South Africa, or in a few catchments in southern Africa where these systems models may have been applied. The Water Evaluation and Planning (WEAP) model (Sieber and Purkey, 2007) is an easy-to-use water accounting model that was developed by the Stockholm Environment Institute, and has been applied to catchments in many developing countries (e.g. Levite et al., 2003; Hollermann et al., 2010; Hamlat et al., 2013; Dimova et al., 2014; Li et al., 2015). Although the WEAP model has been proven to be enormously useful for modelling water quantity in data-poor regions, the water quality simulation capabilities of the model for long-term catchment-level management remain limited. A valid approach to increasing the utility of WQSAM outside of South Africa would be to adapt WQSAM to take as input, flow output of the WEAP model.
The aim of the current study is to describe the process of adapting the WQSAM model to accept flow data output of the WEAP model, and by application of the model to a case study catchment, to illustrate the validity of the approach.
2.1 Study area
The Buffalo River in the Eastern Cape, South Africa was used as a case study catchment for the current study (Fig. 1). This river is relatively short (125 km from headwaters to sea), with a maximum order (Strahler, 1957) of 4 and few tributaries. Although rainfall occurs year round, most rainfall occurs during summer, and there is a steep rainfall gradient between the upper (1500–2000 mm) and the middle (500–625 mm) reaches. Four impoundments are situated on the river: Maden and Rooikrans dams in the upper catchment and the larger Laing Dam and Bridle Drift Dam in the middle and lower catchment, respectively (Fig. 1). It must be emphasised that the current study modelled water quality up to Laing Dam (middle catchment). The natural salinity of the river is relatively high as the geology of the region is dominated by marine sediments of the Beaufort Series. Human activities affecting water quality include some commercial farming in the upper catchment, and once water moves through the middle catchment, there is a pronounced deterioration of water quality due to pollution from the urban/industrial complex of King Williams Town and Zwelitcha (see Fig. 1). The pollution of the middle catchment mainly manifests as eutrophication, resulting from nutrient inputs from various WWTPs. This eutrophication is mainly manifested in Laing Dam, resulting in blooms of Water Hyacinth (Eichhornia crassipes) and Microcystis.
2.2 Models used
The Water Evaluation and Planning (WEAP) Model (Sieber and Purkey, 2007) was used to simulate monthly flows within the catchment. WEAP is an “off-the-shelf” water accounting model, used for simulating natural hydrology through a rainfall-runoff function, and is also capable of simulating human-altered flow through simulating human use extractions and return flows. WEAP has been widely applied due to its ease of use. Although WEAP is a good systems model for simulating water quantity, the model incorporates only very rudimentary water quality simulation functionality, with conservative water quality variables modelled through dilution and non-conservative variables modelled though a single globally-applicable degradation coefficient. Although WEAP does allow integration of the QUAL2K model (Pelletier et al., 2006) for modelling water quality, this approach is not ideal as QUAL2K is a highly detailed water quality model with a high spatial and temporal resolution, meant for detailed modelling of small stretches of river, whereas WEAP would typically be applied at a catchment level for long-term simulations of water quantity.
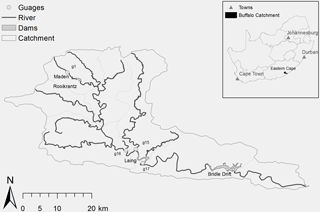
Figure 1Map of Buffalo River Catchment within the Eastern Cape, South Africa. Water quality monitoring points used in the study for calibration of the models are indicated: g1 – Maden Dam; g16 – R20D; g15 – Yellowwoods River; g17 – Laing Dam.
The Water Quality Systems Assessment Model (WQSAM) was used to simulate water quality. As mentioned in the Introduction, WQSAM was designed to link directly to systems models routinely used in South African water resource management; the flows generated by the systems models drive the water quality simulations in WQSAM. At the conceptual level, WQSAM can be represented by several levels (tiers) (Slaughter et al., 2012). The functionality of the first tier would be to facilitate the communication of WQSAM with the systems model in allowing the input of flow data. WQSAM is run within the Spatial And Time Series Information Modelling (SPATSIM) modelling framework (Hughes, 2004). Typically, the systems models (WRYM or WReMP) generate monthly flows, as monthly flows are regarded as adequate for the needs of long-term water quantity management in South Africa. Since water quality is strongly affected by transient events (Britton et al., 1993), such as rainfall-runoff events, WQSAM is operated on a daily time step. The monthly flows are therefore disaggregated to daily within WQSAM, represented by the second tier of the model. A detailed description of the disaggregation method is available in Slaughter et al. (2015), and is further explored in Hughes and Slaughter (2015). The third tier of WQSAM represents the separation of incremental flow into the three flow fractions: surface water flow, interflow and ground water flow. This process is achieved through the simple statistical baseflow separation method of Hughes et al. (2003). The flow separation is of particular importance for simulation of non-point source loads, as water quality signatures (concentrations) are assigned to the flow fractions, the values of which are guided by the predominant land covers in the sub-catchments. The disaggregation of monthly cumulative flow into daily, including return flows, abstractions and reservoir releases, is represented by the fourth tier and has been described in Hughes and Slaughter (2016). All water quality simulation, including that of nutrients, salinity, water temperatures and microbial water quality, is represented by the final tier of the model, and includes processes such as decomposition, chemical speciation and algal uptake (Slaughter et al., 2012).
Within the current study, the model functionality of the WQSAM model was updated to read in monthly flow output from the WEAP model. The updated part of the WQSAM model is conceptually represented by the first tier. Briefly, WEAP outputs simulations in various forms, including tables and graphs, but usefully for the present study, the unprocessed monthly simulations can be output to an excel spreadsheet as a comma-delimited file. The WQSAM functionality represented as tier one to read in monthly flow data was therefore updated to provide the option of reading in comma-delimited files. The user interface of WQSAM to allow this option is shown in Fig. 2.
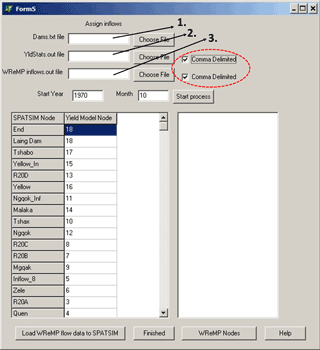
Figure 2User interface of the Water Quality Systems Assessment Model (WQSAM) (Slaughter et al., 2012) for reading in flow outputs of the systems models.
Information on dams on the system, such as dam capacity, are written to a text file, and would be input into WQSAM in the field represented as “1” in Fig. 2. The “YldStats.out” file is a standard output file of the Water Resources Modelling Platform (WReMP) (Mallory et al., 2011), and includes information on outflows from subcatchments and reservoirs and reservoir water levels at monthly time steps, and is input into WQSAM in the field represented as “2” in Fig. 2. The “inflows.out” file is a standard output file of the Water Resources Modelling Platform (WReMP) (Mallory et al., 2011), and includes information on both incremental and cumulative inflows to subcatchments and reservoirs at monthly time steps, and is input into WQSAM in the field represented as “3” in Fig. 2. By checking the check boxes indicated in the circle in Figure 2, the model functionality to read in the two WReMP files as comma delimited files is activated, thereby allowing the model to read in flow outputs from the Water Evaluation and Planning (WEAP) model (Sieber and Purkey, 2007). It must be emphasised that WQSAM cannot at this stage simply ready in the flow output files of WEAP in their raw form. The flow outputs of WEAP are reformatted and separated into the flows corresponding to the YldStats.out and inflows.out files mentioned above. This can be a time-consuming process.
Once monthly flows are read into the WQSAM model, all other processes are run without any changes: monthly flows are disaggregated to daily, flows are fractioned and water quality is simulated.
2.3 Model setup
WEAP was used to simulate future water quantity within the Buffalo River for the period 1979–2000 on a monthly time step. More information on the WEAP model setup and water use is available in Mantel et al. (2015). Briefly, historical rainfall and evaporation/temperature data were obtained from the South African WR2005 database (Middleton and Bailey, 2008). The natural hydrology was generated within WEAP using these data using a built-in rainfall-runoff module. By considering reservoirs in the catchment as well as current water demands, WEAP was able to simulate altered hydrology. A report by the South African Department of Water Affairs and Forestry (DWAF, 2008) was used to estimate current water demands for the system, and for simplification, were divided into three demand areas: the upper, middle and lower catchments. The present day (current) demand was considered to be a stationary demand in WEAP for the modelled years 1971–2000. The water losses due to evapotranspiration by invasive plants was also considered in the WEAP application for the Amatole system. Reticulation losses were also considered. Calibration of stream flows was achieved using observed data for 11 gauging stations on the system.
The WEAP monthly modelled flows were output to comma-delimited files and reformatted into the YldStats.out and Inflows.out files, following which the data were input into WQSAM through the user interface represented in Fig. 2. Monthly incremental flows were disaggregated to daily using observed daily rainfall from the rainfall database by Lynch (2004). Water temperature was simulated using the daily observed air temperature database by Schulze and Maharaj (2004). Water quality data collected by the South African Department of Water and Sanitation (DWS) were used to calibrate the model.
3.1 Inputs of flow data from the WEAP model into WQSAM
The study by Mantel et al. (2015) found that the WEAP model was in general able to represent the observed flow of the Buffalo River catchment for historical conditions. To illustrate whether the WQSAM model was able to input the monthly flow data generated by the WEAP model, we chose two example sub-catchments, R20B and Yellowwoods, for which the WEAP model simulated flow relatively inaccurately and relatively accurately, respectively. For the R20B subcatchment (Fig. 3a), the time series of simulated monthly flows generated by the WEAP model (top graph) appears to be too high compared to observed monthly flows. Once the flows were input into the WQSAM model, the process of monthly-daily disaggregation produced daily flows (middle graph) that were similarly too high compared to observed daily flows. The inaccuracies in the monthly and daily flows are illustrated in the flow duration curves (lower graph), where the comparisons between simulated and observed flows for monthly and daily time steps generated Nash-Sutcliffe Efficiency (NSEs) (Nash and Sutcliffe, 1970) of 0.16 and 0.1, respectively. For the Yellowwoods River subcatchment, the WEAP model generated monthly flows that were more representative of the observed flows, as can be seen in the top graph of Fig. 3b. The monthly-daily disaggregation in WQSAM produced daily flows that were similarly representative of the observed daily flows (middle graph). The flow duration curves within the lower graph show that the comparisons between simulated and observed flows for monthly and daily time steps generated NSEs of 0.93 and 0.98, respectively.
3.2 Water quality modelling in WQSAM
Observed water quality data were available for Maden Dam, R20D, the Yellowwoods River and Laing Dam. Maden Dam is at the headwaters of the catchment, and water quality is therefore relatively good. TDS, NO3-N + NO2-N, NH4-N and PO4-P for Maden Dam ranges between 30 to 130 mg L−1, 0 to 1.1 mg L−1, 0 to 1.4 mg L−1 and 0 to 1.0 mg L−1, respectively. To achieve calibration to the observed data for Maden Dam, signatures of surface water, interflow and groundwater flow for TDS were set at 30, 30 and 80 mg L−1, respectively. For the nutrients, only the surface water signatures were set, with values of 0.2, 0.2 and 0.1 mg L−1 for NO3-N + NO2-N, NH4-N and PO4-P, respectively. Minimal algal growth was simulated within the dam. The water quality of the R20D catchment shows a significant deterioration compared to the headwaters as this point is within the middle catchment and is affected by return flows and runoff from urban areas. TDS in the catchment shows a sharp rise, with values ranging between 100 and 5000 mg L−1. Nutrients also show a sharp rise, with highest concentrations of NO3-N + NO2-N, NH4-N and PO4-P of approximately 46, 14 and 6 mg L−1, respectively. To achieve calibration for TDS, surface water, interflow and groundwater flow concentrations were set to 100, 500 and 1200 mg L−1, respectively. For the nutrients, surface flow concentrations were set to high levels to achieve calibration, with values 1, 5 and 0.5 mg L−1 for NO3-N + NO2-N, NH4-N and PO4-P, respectively. The concentrations of return flow for the nutrients were also set at high levels to represent sewage return flow into the river at that point, with values of 10, 0.5 and 1.5 mg L−1 for NO3-N + NO2-N, NH4-N and PO4-P, respectively. The water quality of the Yellowwoods River is also fairly compromised compared to the upper catchment, as the river receives sewage return flow input and runoff from informal settlements. TDS within the river ranges between 100 and 1300 mg L−1. Nutrients are also fairly high, with concentrations of NO3-N + NO2-N, NH4-N and PO4-P being as high as 5.5, 10 and 3 mg L−1, respectively. To achieve calibration to the observed TDS, signatures of 100, 500 and 1600 mg L−1 were assigned to surface flow, interflow and groundwater flow, respectively. The surface flow concentrations for nutrients were set high to achieve calibration, with values of 5, 1.5 and 1 mg L−1 for of NO3-N + NO2-N, NH4-N and PO4-P, respectively. Return flow concentrations were set fairly low, with values of 0.5, 0.1 and 0.1 for NO3-N + NO2-N, NH4-N and PO4-P, respectively. This indicates that non-point sources have a bigger impact on the Yellowwoods River than point sources. Laing Dam appears to act as a water quality sink, as although the water quality is generally compromised, it is nevertheless an improvement compared to the water quality of the dam inflow. Observed TDS ranges between 150 to 500 mg L−1. The highest observed concentrations of nutrients are approximately 3, 1.5 and 1.5 mg L−1 for NO3-N + NO2-N, NH4-N and PO4-P, respectively.
The NSEs generated by comparing model simulations of water quality in WQSAM to observed data are shown in Table 1. The simulations of TDS appear to be most representative of observed data. The model simulations of water quality for Laing Dam represented the most inaccurate representations of the observed data. The NSEs for the other subcatchments/reservoirs ranged between 0.64–0.93, 0.84–0.93, 0.14–0.93 and 0.47–0.93 for TDS, nitrate plus nitrite, ammonia and phosphate, respectively.
Table 1Nash-Sutcliffe Efficiency (NSE) (Nash and Sutcliffe, 1970) values calculated for the Water Quality Systems Assessment Model (WQSAM) (Slaughter et al., 2012) simulations compared against historical observed data for various water quality variables for various subcatchments/reservoirs of the Buffalo River catchment.
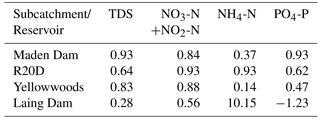
4.1 Model results
Figure 3 shows that WEAP generated a relatively poor representation of observed monthly flow in the R20B subcatchment (see Fig. 3a), with the NSE value being 0.16. This monthly flow was input into WQSAM and disaggregated from monthly to daily, and then compared to the daily observed flow, generating an NSE of 0.10. Therefore, the poor monthly flows generated by the WEAP model were carried over into the WQSAM model, shown by the observed monthly versus simulated monthly and the observed daily versus simulated daily flow duration curves, respectively. In contrast, the monthly flows generated by the WEAP model for the Yellowwoods River were a good representation of the observed data, generating an NSE value of 0.93.
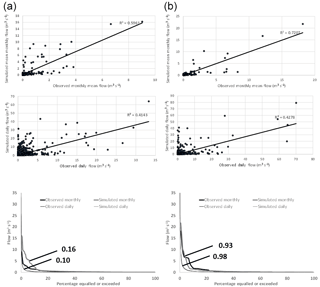
Figure 3Observed and simulated flow for: (a) R20B and; (b) Yellowwoods on the Buffalo River in the Eastern Cape for the period 1971–2000. The top graph shows the X–Y scatter plot of the observed monthly time series versus WEAP (Sieber and Purkey, 2007) model simulations of monthly flow, the middle graph shows the X–Y scatter plot of the daily observed flow versus daily simulated flow generated through monthly-daily disaggregation within the WQSAM (Slaughter et al., 2012) model and the lower graph shows daily observed versus daily simulated and monthly observed versus monthly simulated flow represented as duration curves, with the Nash-Sutcliffe efficiencies (Nash and Sutcliffe, 1970) shown between each comparison.
This good representation of flow was carried through to the WQSAM model, and subsequent the monthly-daily flow disaggregation, a comparison between daily observed and daily simulated flow yielded an NSE of 0.98. The results shown in Fig. 3 suggest that flow was successfully input from WEAP into WQSAM; therefore, the additional functionality built into WQSAM to input WEAP-generated flows appears to be effective.
Water quality simulations within WQSAM obtained mixed results. It is evident that the model struggled to simulate water quality within Laing Dam. The following summary of results therefore excludes the results for Laing Dam. Unsurprisingly, model simulations of the conservative variable TDS were relatively representative of observed data, with NSE values ranging between 0.64–0.93. Simulations of nitrates plus nitrites were also relatively representative of observed data, with NSE values ranging between 0.56–0.93. Simulations of ammonium and phosphate were mixed, and although some good results were obtained, some poor results were also obtained. The poor model NSE results for ammonium within Maden Dam as well as the Yellowwoods River and phosphates within the Yellowwoods River could be related to the scarcity of observed data as well as a few observations of very high concentrations, which could possibly be due to measurement or data capture errors: in general, the model simulations were representative of the observed data except for a few extreme spikes in the observed data. WQSAM models TDS at this stage primarily through dilution. The relatively good simulation results obtained for TDS by the model indicate that in general, the simulated flows used in the model were representative of the observed flows, which further confirms that the WQSAM model was successfully able to input the monthly flows generated by the WEAP model. The mixed results obtained for nutrient simulations by the model indicate model uncertainties and possible inadequacies in representing observed water quality that are probably not due to incorrect flow, but rather possibly due to incorrect or insufficient representation of certain water quality processes. It is also possible that the extreme spikes in the observed water quality data, particularly for nutrients, may be related to measurement or data capture errors. Since there are few observed data, the fact that WQSAM was in most cases not able to represent these spikes, sometimes led to poor NSE estimates.
4.2 The benefit of modelling water quality in WQSAM
As already mentioned in the Introduction, WQSAM aims to represent the most important water quality processes that explain the majority of variation in observed flows. In this way, relative simplicity of the model can be maintained, thereby also limiting the observed data required to calibrate the model. Many systems models, such as the WEAP application to the Buffalo River Catchment (Mantel et al., 2015) and all model setups of the WReMP and WRYM models in South Africa, are at a monthly time step. This is because simulations of water quantity at a monthly time step are generally sufficiently accurate for long-term water quantity management in South Africa. Simulation at a monthly time step would not be sufficiently accurate for water quality, as water quality is typically driven by transient events, such as rainfall-runoff events. An important advantage of WQSAM is its ability to integrate with the monthly-time-step systems models, generating water quality at a daily time step, thereby utilising the existing systems model setups and avoiding complex daily-time-step systems models. An example of the benefit of modelling at a daily time step as opposed to a monthly time step can be seen in Fig. 4a, which shows the frequency distributions of simulations of TDS at a daily time step and monthly time step by WQSAM and the WEAP model, respectively, for the R20D subcatchment in the Buffalo River Catchment, along with the frequency distribution of observed TDS. The simulation of water quality within the Buffalo River by WEAP was conducted as part of the study by Slaughter et al. (2016). It is evident that the simulation of TDS by WQSAM shows more variation, and is more representative of the observed daily data, whereas the simulation by WEAP is of monthly averages, and does not represent the full variation of the observed data. Obviously, it would be of greater use for water management to be aware of the full variation of a particular water quality variable, so as to estimate the real risk of exceeding certain management thresholds, associated with particular management actions. As can be seen in Fig. 4b, which shows the X–Y plot of WQSAM-simulated daily TDS versus observed TDS, a relatively poor correlation is obtained. It must be considered that WQSAM is not designed to accurately match observed water quality observations at a daily time scale, but rather to generate a representative frequency distribution of long-term water quality observations.
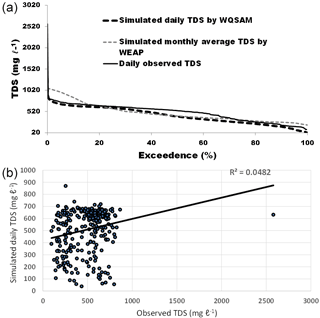
Figure 4Frequency distribution of observed total dissolved solids (TDS), modelled daily TDS by the Water Quality Systems Assessment Model (WQSAM) and modelled average monthly TDS by the monthly-time-step Water Evaluation and Planning (WEAP) model (Sieber and Purkey, 2007) for the R20D catchment on the Buffalo River, Eastern Cape, South Africa. (b) X–Y plots of observed TDS and simulated TDS by WQSAM for the same catchment.
The present study showed that the WQSAM model can be updated to take as input, monthly flow data generated by the WEAP model. This development dramatically increases the potential for application of the WQSAM model to catchments outside of South Africa, and offers the possibility of a water quality model suitable for semi-arid data-scarce catchments worldwide.
The data used in this study are available at https://doi.org/10.6084/m9.figshare.5370799 (Slaughter and Mantel, 2017b).
The authors declare that they have no conflict of interest.
This article is part of the special issue “Water quality and
sediment transport issues in surface water”. It is a result of the IAHS
Scientific Assembly 2017, Port Elizabeth, South Africa, 10–14 July
2017.
Edited by: Akhilendra B.
Gupta
Reviewed by: Jagdish Kumar Bassin
and
one anonymous referee
Adler, R. A., Claassen, M., Godfrey, L., and Turton, A.: Water, mining, and waste: an historical and economic perspective on conflict management in South Africa, The Economics of Peace and Security Journal, 2, 33–41, 2007.
Basson, M. S., Allen, R. B., Pegram, G. G. S., and van Rooyen, J. A.: Probabilistic Management of Water Resource and Hydropower Systems, Water Resources Publ., Colorado, USA, 424 pp., 1994.
Britton, D. L., Day, J. A., and Henshal-Howard, M.-P.: Hydrological response during storm events in a South African mountain catchment: the influence of antecedent conditions, Hydrobiologia, 250, 143–157, 1993.
Britz, T. J. and Sigge, G. O.: Quantitative investigation into the link between irrigation water quality and food safety: Volumes 1–4. Water Research Commission report No. 1773/1/12, Water Research Commission, Pretoria, South Africa, 2012.
Dimova, G., Tzanov, E., Ninov, P., Ribarova, I. and Kossida, M.: Complementary use of the WEAP model to underpin the development of SEEAW physical water use and supply tables, Procedia Engineering, 70, 563–572, 2014.
Gorgens, A. H. M. and de Clercq, W. P.: Research on Berg River Water Management – Summary of Water Quality Information System and Soil Quality Studies. Water Research Commission Report No TT252/06. Water Research Commission, Pretoria, South Africa, 2006.
Hamlat, A., Errih, M., and Guidoum, A.: Simulation of water resources management scenarios in western Algeria watersheds using WEAP model, Arab Journal of Geosciences, 6, 2225–2236, 2013.
Hollermann, B., Giertz, S., and Diekkruger, B.: Benin 2025-Balancing Future Water Availability and Demand Using the WEAP “Water Evaluation and Planning” System, Water Resour. Manag., 24, 3591–3613, 2010.
Hughes, D. A. (Ed.): SPATSIM, an Integrating Framework for Ecological Reserve Determination and Implementation. WRC Report No. TT 245/04. Water Research Commission, Pretoria, South Africa, 2004.
Hughes, D. A. and Slaughter, A. R.: Daily disaggregation of simulated monthly flows using different rainfall datasets in southern Africa, Journal of Hydrology: Regional Studies, 4, 153–171, 2015.
Hughes, D. A. and Slaughter, A. R.: Disaggregating the components of a monthly water resources system model to daily values for use with a water quality model, Environ. Modell. Softw., 80, 122–131, 2016.
Hughes, D. A., Hannart, P., and Watkins, D.: Continuous baseflow separation from time series of daily and monthly streamflow data, Water SA, 29, 43–48, 2003.
Levite, H., Sally, H., and Cour, J.: Testing water demand management scenarios in a water-stressed basin in South Africa: application of the WEAP model, Phys. Chem. Earth, 28, 779–786, 2003.
Li., X., Zhao, Y., Shi, C., Sha, J., Wang, Z.-L., and Wang, Y.: Application of Water Evaluation and Planning (WEAP) model for water resources management strategy estimation in coastal Binhai New Area, China, Ocean Coast. Manage., 106, 97–109, 2015.
Lynch, S. D.: Development of a raster database of annual, monthly and daily rainfall for Southern Africa. WRC Report No. 1156/1/04, Water Research Commission, Pretoria, South Africa, 2004.
Mallory, S. J. L., Odendaal, P., and Desai, A.: The Water Resources Modelling Platform User's Guide v3.3. Unpublished document available from stephen@waterresources.co.za, 2011.
Mantel, S. K., Hughes, D. A., and Slaughter, A. S.: Water Resources Management in the Context of Future Climate and Development Changes: A South African Case Study, Journal of Water and Climate Change, 6, 772–786, 2015.
Middleton, B. J. and Bailey, A. K.: Water Resources of South Africa, 2005 Study (WR2005), WRC Report No. TT381/08, Water Research Commission, Pretoria, South Africa, 2008.
Nash, J. E. and Sutcliffe, J. V.: River flow forecasting through conceptual models. Part I – a discussion of principles, J. Hydrol., 10, 282–290, 1970.
Pelletier, G. J., Chapra, S. C., and Tao, H.: QUAL2Kw – A framework for modeling water quality in streams and rivers using a genetic algorithm for calibration, Environ. Modell. Softw., 21, 419–425, 2006.
Piesold, K., Moahloli, G., Steyn, K., and Shand, N.: Berg River Dam: Further Water Quality Studies, Reservoir Modelling Report Report No. C201.51-171 R, 2007.
Pitman, W. V.: A mathematical model for generating river flows from meteorological data in South Africa. Report no. 2/73, Hydrological Research Unit, University of the Witwatersrand, Johannesburg, South Africa, 1973.
Schulze, R. E. (Ed.): ACRU: Background Concepts and Theory. ACRU Report No. 36, Dept. Agric. Eng., Univ. of Natal, Pietermaritzburg, RSA. SCHULZE RE (1995) Hydrology and Agrohydrology: A Text to Accompany the ACRU 3.00 Agrohydrological Modelling System, WRC, 1989.
Schulze, R. E. and Maharaj, M.: Development of a Database of Gridded Daily Temperatures for Southern Africa. WRC Report No. 1156/2/04. Water Research Commission, Pretoria, South Africa, 2004.
Sieber, J. and Purkey, D.: Water Evaluation and Planning System. User Guide for WEAP21. Stockholm Environment Institute, Massachusetts, USA, available at: http://www.weap21.org (last access: 1 September 2017), 2007.
Simate, G. S. and Ndlovu, S.: Acid mine drainage: challenges and opportunities, Journal of Environmental Chemistry and Engineering, 2, 1785–1803, 2014.
Slaughter, A. R.: Simulating microbial water quality in data-scarce catchments: an update of the WQSAM model to simulate the fate of Escherichia coli, Water Resour. Manag., 1–14, https://doi.org/10.1007/s11269-017-1743-1, 2017.
Slaughter, A. R. and Mantel, S. K.: The validation of algal growth processes in a water quality model using remote sensing data, Proceedings of the 8th International Environmental Modelling and Software Society (iEMSs) biennial meeting, Toulouse, France, 10–14 July 2016, http://scholarsarchive.byu.edu/cgi/viewcontent.cgi?article=1374&context=iemssconference (last access: 1 September 2017), 2016.
Slaughter, A. R. and Mantel, S. K.: Land cover models to predict non-point nutrient inputs for selected biomes in South Africa, Water SA, 43, 499–508, 2017a.
Slaughter, A. R. and Mantel, S. K.: Water quality modelling of an impacted semi-arid catchment using flow data from the WEAP model: data and graphs, https://doi.org/10.6084/m9.figshare.5370799, 2017b.
Slaughter, A. R., Hughes, D. A., and Mantel, S. K.: The development of a Water Quality Systems Assessment Model (WQSAM) and its application to the Buffalo River catchment, Eastern Cape, South Africa, Proceedings of the 6th International Environmental Modelling and Software Society (iEMSs) biennial meeting, Leipzig, Germany 1–5 July 2012, ISBN: 978-88-9035-742-8, http://www.iemss.org/sites/iemss2012//proceedings/I2_2_0497_Slaughter_et_al.pdf (last access: 1 September 2017), 2012.
Slaughter, A. R., Retief, D. C. H., and Hughes, D. A.: A method to disaggregate monthly flows to daily using daily rainfall observations: model design and testing, Hydrological Sciences Journal, 4, 153–171, https://doi.org/10.1080/02626667.2014.993987, 2015.
Slaughter, A. R., Mantel, S. K., and Hughes, D. A.: Water Quality Management in the Context of Future Climate and Development Changes: A South African Case Study, Journal of Water and Climate Change, 8, jwc2016138, https://doi.org/10.2166/wcc.2016.138, 2016.
Slaughter, A. R., Hughes, D. A., Retief, D. C. H., and Mantel, S. K.: A management-oriented water quality model for data scarce catchments, Environ. Modell. Softw., 97, 93–111, 2017.
South African Department of Water Affairs (DWAF): Development of a Reconciliation Strategy for the Amatole Bulk Water Supply System. Final Report. Two Volumes. Prepared by SSI Engineers and Environmental Consultants on behalf of the Department of Water Affairs and Forestry Director, National Water Resource, Pretoria, South Africa, 2008.
Stirzaker, R., Biggs, H., and Roux, D.: Requisite simplicities to help negotiate complex problems, AMBIO, 39, 600–607, 2010.
Strahler, A. N.: Quantitative Analysis of Watershed Geomorphology, Trans. Am. Geophys. Union, 38, 913–920, 1957.
Verhoeven, J. T. A., Arheimer, B., Yin, C., and Hefting, M.: Regional and global concerns over wetlands and water quality, Trends Ecol. Evol., 21, 96–103, 2006.